ConsInstancy: learning instance representations for semi-supervised panoptic segmentation of concrete aggregate particles
Machine Vision and Applications(2022)
摘要
We present a semi-supervised method for panoptic segmentation based on ConsInstancy regularisation, a novel strategy for semi-supervised learning. It leverages completely unlabelled data by enforcing consistency between predicted instance representations and semantic segmentations during training in order to improve the segmentation performance. To this end, we also propose new types of instance representations that can be predicted by one simple forward path through a fully convolutional network (FCN), delivering a convenient and simple-to-train framework for panoptic segmentation. More specifically, we propose the prediction of a three-dimensional instance orientation map as intermediate representation and two complementary distance transform maps as final representation, providing unique instance representations for a panoptic segmentation. We test our method on two challenging data sets of both, hardened and fresh concrete, the latter being proposed by the authors in this paper demonstrating the effectiveness of our approach, outperforming the results achieved by state-of-the-art methods for semi-supervised segmentation. In particular, we are able to show that by leveraging completely unlabelled data in our semi-supervised approach the achieved overall accuracy (OA) is increased by up to 5% compared to an entirely supervised training using only labelled data. Furthermore, we exceed the OA achieved by state-of-the-art semi-supervised methods by up to 1.5%.
更多查看译文
关键词
ConsInstancy training,Semi supervision,Panoptic segmentation,Instance representations,Concrete aggregate
AI 理解论文
溯源树
样例
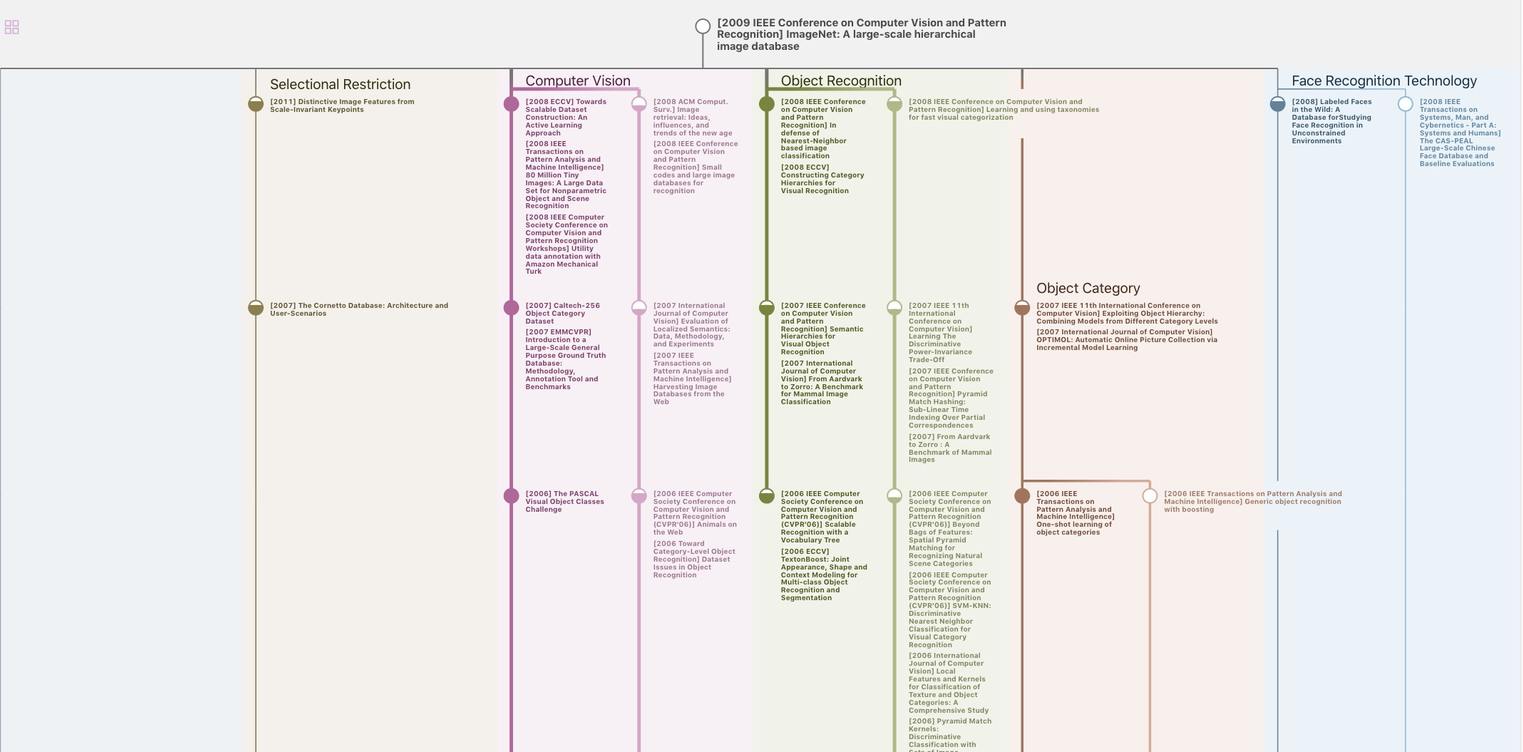
生成溯源树,研究论文发展脉络
Chat Paper
正在生成论文摘要