One-stage pulmonary nodule detection using 3-D DCNN with feature fusion and attention mechanism in CT image
Computer Methods and Programs in Biomedicine(2022)
摘要
Background and objective
Lung cancer is the most common cause of cancer-related death in the world. Low-dose computed tomography (LDCT) is a widely used modality in lung cancer detection. The nodule is an abnormal tissue and may evolve into lung cancer. Hence, it is crucial to detect nodules in the early detection stage. However, reviewing the LDCT scans to observe suspicious nodules is a time-consuming task. Recently, designing a computer-aided detection (CADe) system with convolutional neural network (CNN) architecture has been proven that it is helpful for radiologists. Hence, in this study, a 3-D YOLO-based CADe system, 3-D OSAF-YOLOv3, is proposed for nodule detection in LDCT images.
Methods
The proposed CADe system consists of data preprocessing, nodule detection, and non-maximum suppression algorithm (NMS). At first, the data preprocessing including the background elimination, the spacing normalization, and the volume of interest (VOI) extraction, are conducted to remove the non-lung region, normalize the image spacing, and divide LDCT image into numerous VOIs. Then, the VOIs are fed into the 3-D OSAF-YOLOv3 model, to detect the suspicious nodules. The proposed model is constructed by integrating the 3-D YOLOv3 with the one-shot aggregation module (OSA), the receptive field block (RFB), and the feature fusion scheme (FFS). Finally, the NMS algorithm is performed to eliminate the duplicated detection generated by the model.
Results
In this study, the LUNA-16 dataset composed 1186 nodules from 888 LDCT scans and the competition performance metric (CPM) are used to evaluate our CADe system. In the experiment results, the proposed system can achieve a sensitivities rate of 0.962 with the false positive rate of 8 and complete a CPM value of 0.905. Moreover, according to the ablation study results, the employment of OSA module, RFB, and FFS could improve the detection performance actually. Furthermore, compared to other start-of-the-art (SOTA) models, our detection system could also achieve the higher performance.
Conclusions
In this study, a YOLO-based CADe system for nodule detection in CT image system integrating additional modules and scheme is proposed for nodule detection in LDCT. The result indicates that the proposed the modification can significantly improve detection performance.
更多查看译文
关键词
Lung nodule detection,Computer-aided detection,YOLO,Receptive field block,One-shot aggregation,Feature fusion scheme
AI 理解论文
溯源树
样例
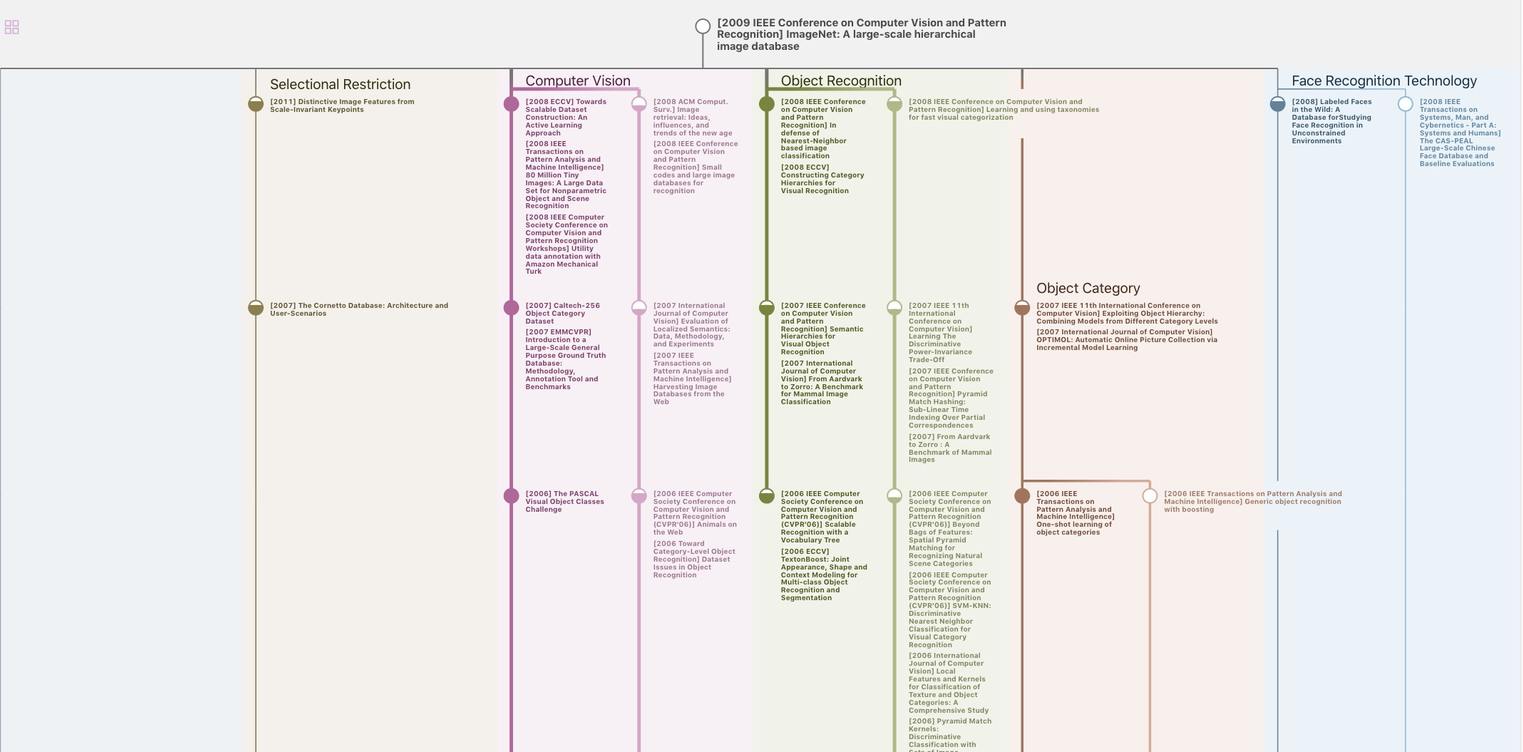
生成溯源树,研究论文发展脉络
Chat Paper
正在生成论文摘要