Bidirectional Hybrid LSTM Based Recurrent Neural Network for Multi-view Stereo.
IEEE transactions on visualization and computer graphics(2022)
摘要
Recently, deep learning based multi-view stereo (MVS) networks have demonstrated their excellent performance on various benchmarks. In this paper, we present an effective and efficient recurrent neural network (RNN) for accurate and complete dense point cloud reconstruction. Instead of regularizing the cost volume via conventional 3D CNN or unidirectional RNN like previous attempts, we adopt a bidirectional hybrid Long Short-Term Memory (LSTM) based structure for cost volume regularization. The proposed bidirectional recurrent regularization is able to perceive full-space context information comparable to 3D CNNs while saving runtime memory. For post-processing, we introduce a visibility based approach for depth map refinement to obtain more accurate dense point clouds. Extensive experiments on DTU, Tanks and Temples and ETH3D datasets demonstrate that our method outperforms previous state-of-the-art MVS methods and exhibits high memory efficiency at runtime.
更多查看译文
关键词
3D reconstruction,deep learning,multi-view stereo,recurrent neural network,point clouds
AI 理解论文
溯源树
样例
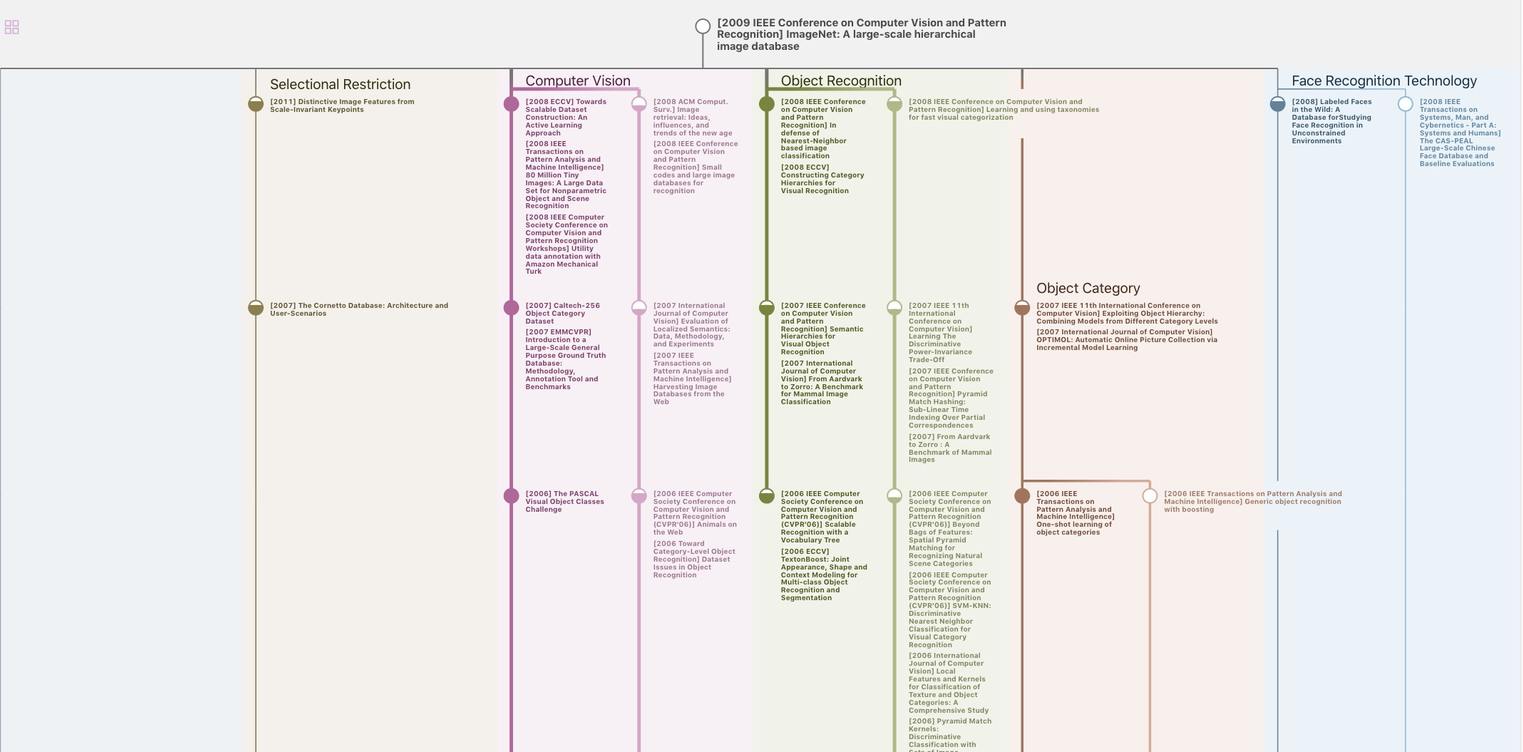
生成溯源树,研究论文发展脉络
Chat Paper
正在生成论文摘要