Dual-Graph Attention Convolution Network for 3-D Point Cloud Classification
IEEE TRANSACTIONS ON NEURAL NETWORKS AND LEARNING SYSTEMS(2022)
摘要
Three-dimensional point cloud classification is fundamental but still challenging in 3-D vision. Existing graph-based deep learning methods fail to learn both low-level extrinsic and high-level intrinsic features together. These two levels of features are critical to improving classification accuracy. To this end, we propose a dual-graph attention convolution network (DGACN). The idea of DGACN is to use two types of graph attention convolution operations with a feedback graph feature fusion mechanism. Specifically, we exploit graph geometric attention convolution to capture low-level extrinsic features in 3-D space. Furthermore, we apply graph embedding attention convolution to learn multiscale low-level extrinsic and high-level intrinsic fused graph features together. Moreover, the points belonging to different parts in real-world 3-D point cloud objects are distinguished, which results in more robust performance for 3-D point cloud classification tasks than other competitive methods, in practice. Our extensive experimental results show that the proposed network achieves state-of-the-art performance on both the synthetic ModelNet40 and real-world ScanObjectNN datasets.
更多查看译文
关键词
Point cloud compression,Convolution,Feature extraction,Shape,Deep learning,Convolutional neural networks,Aggregates,3-D point cloud,geometric attention mechanism,graph convolution networks,intrinsic and extrinsic features
AI 理解论文
溯源树
样例
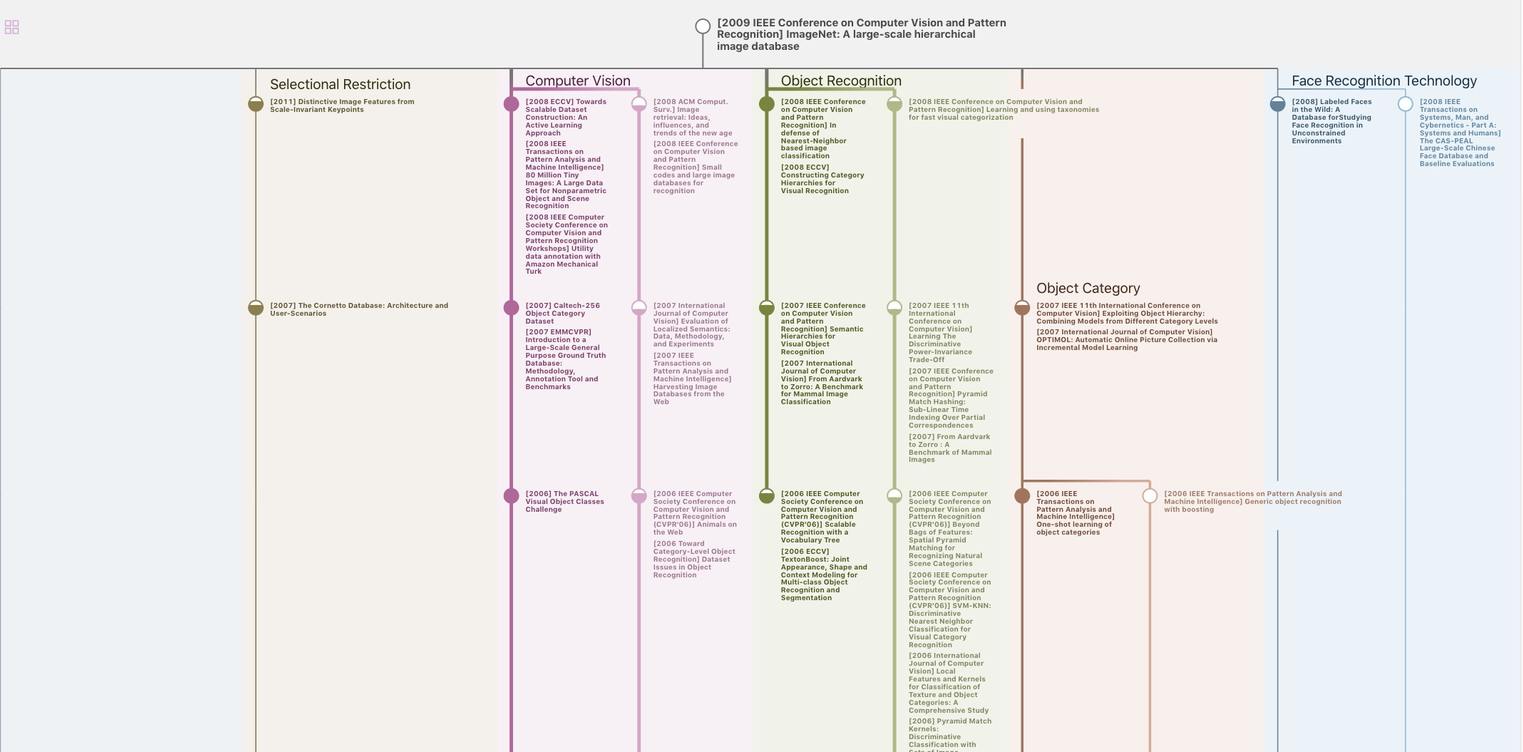
生成溯源树,研究论文发展脉络
Chat Paper
正在生成论文摘要