Does Simultaneous Speech Translation need Simultaneous Models?
arxiv(2022)
摘要
In simultaneous speech translation (SimulST), finding the best trade-off between high translation quality and low latency is a challenging task. To meet the latency constraints posed by different application scenarios, multiple dedicated SimulST models are usually trained and maintained, causing high computational costs and increased environmental impact. In this paper, we show that a single model trained offline can effectively serve not only offline but also simultaneous tasks at different latency regimes, bypassing any training/adaptation procedures. This single-model solution does not only facilitate the adoption of well-established offline techniques and architectures without affecting latency but also yields similar or even better translation quality compared to the same model trained in the simultaneous setting. Experiments on En$\rightarrow$\{De, Es\} indicate the effectiveness of our approach, showing competitive results with the SimulST state of the art.
更多查看译文
关键词
simultaneous speech translation,simultaneous models
AI 理解论文
溯源树
样例
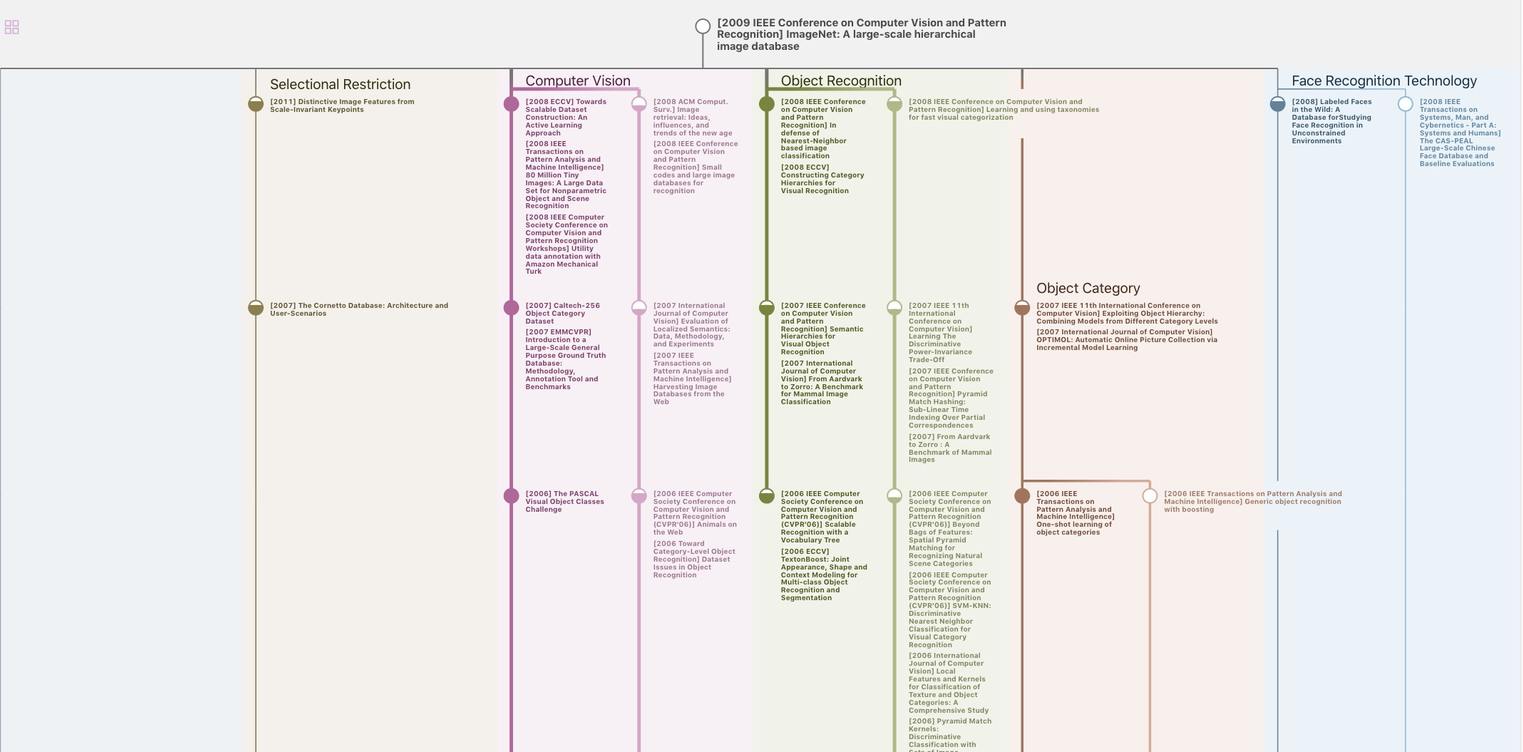
生成溯源树,研究论文发展脉络
Chat Paper
正在生成论文摘要