Machine learning improves global models of plant diversity
biorxiv(2022)
摘要
Despite the paramount role of plant diversity for ecosystem functioning, biogeochemical cycles, and human welfare, knowledge of its global distribution is incomplete, hampering basic research and biodiversity conservation. Here, we used machine learning (random forests, extreme gradient boosting, neural networks) and conventional statistical methods (generalised linear models, generalised additive models) to model species richness and phylogenetic richness of vascular plants worldwide based on 830 regional plant inventories including c. 300,000 species and predictors of past and present environmental conditions. Machine learning showed an outstanding performance, explaining up to 80.9% of species richness and 83.3% of phylogenetic richness. Current climate and environmental heterogeneity emerged as the primary drivers, while past environmental conditions left only small but detectable imprints on plant diversity. Finally, we combined predictions from multiple modelling techniques (ensemble predictions) to reveal global patterns and centres of plant diversity at multiple resolutions down to 7,774 km2. Our predictive maps provide the most accurate estimates of global plant diversity available to date at grain sizes relevant for conservation and macroecology.
### Competing Interest Statement
The authors have declared no competing interest.
更多查看译文
关键词
plant diversity,global models
AI 理解论文
溯源树
样例
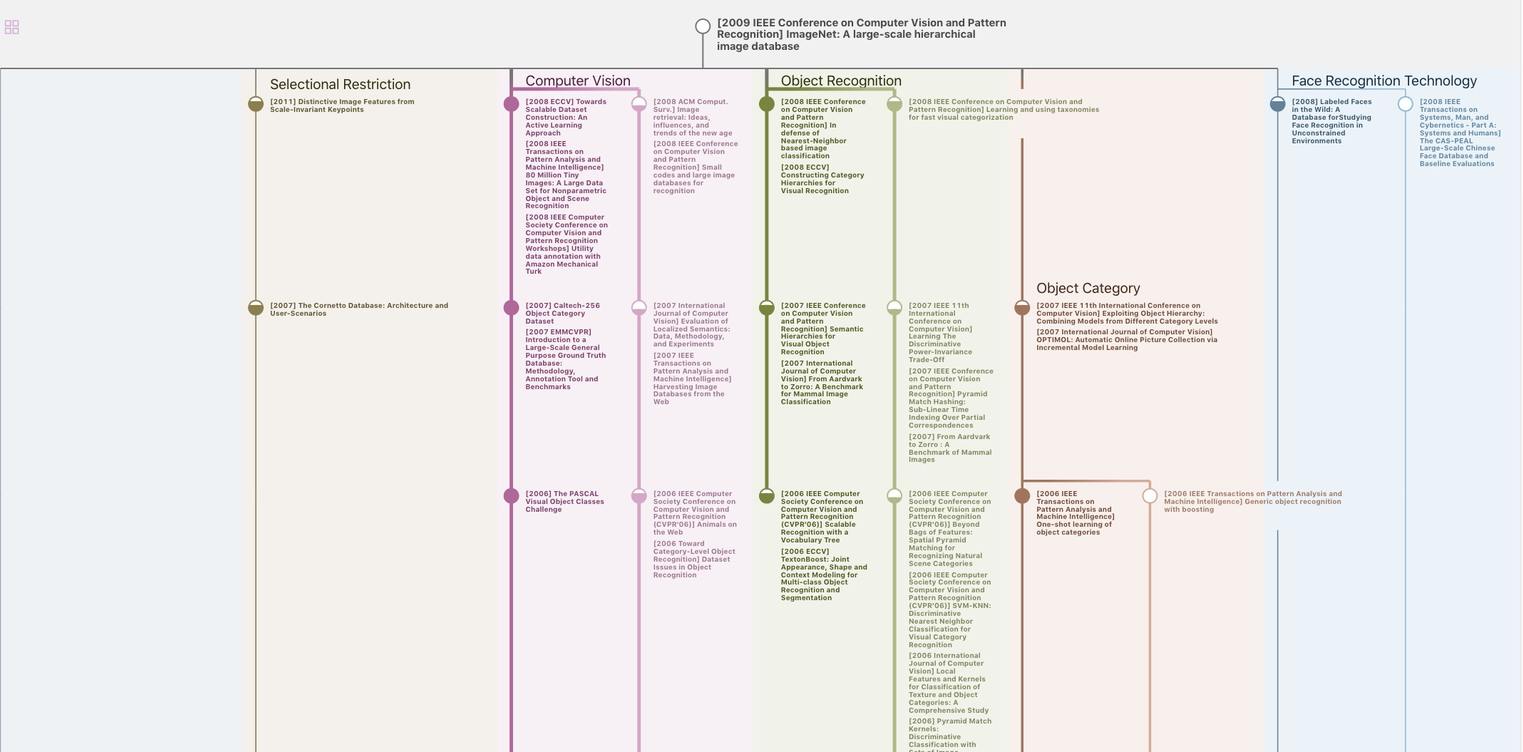
生成溯源树,研究论文发展脉络
Chat Paper
正在生成论文摘要