A novel nonconvex, smooth-at-origin penalty for statistical learning
arxiv(2022)
摘要
Nonconvex penalties are utilized for regularization in high-dimensional statistical learning algorithms primarily because they yield unbiased or nearly unbiased estimators for the parameters in the model. Nonconvex penalties existing in the literature such as SCAD, MCP, Laplace and arctan have a singularity at origin which makes them useful also for variable selection. However, in several high-dimensional frameworks such as deep learning, variable selection is less of a concern. In this paper, we present a nonconvex penalty which is smooth at origin. The paper includes asymptotic results for ordinary least squares estimators regularized with the new penalty function, showing asymptotic bias that vanishes exponentially fast. We also conducted an empirical study employing deep neural network architecture on three datasets and convolutional neural network on four datasets. The empirical study showed better performance for the new regularization approach in five out of the seven datasets.
更多查看译文
关键词
novel nonconvex,learning,smooth-at-origin
AI 理解论文
溯源树
样例
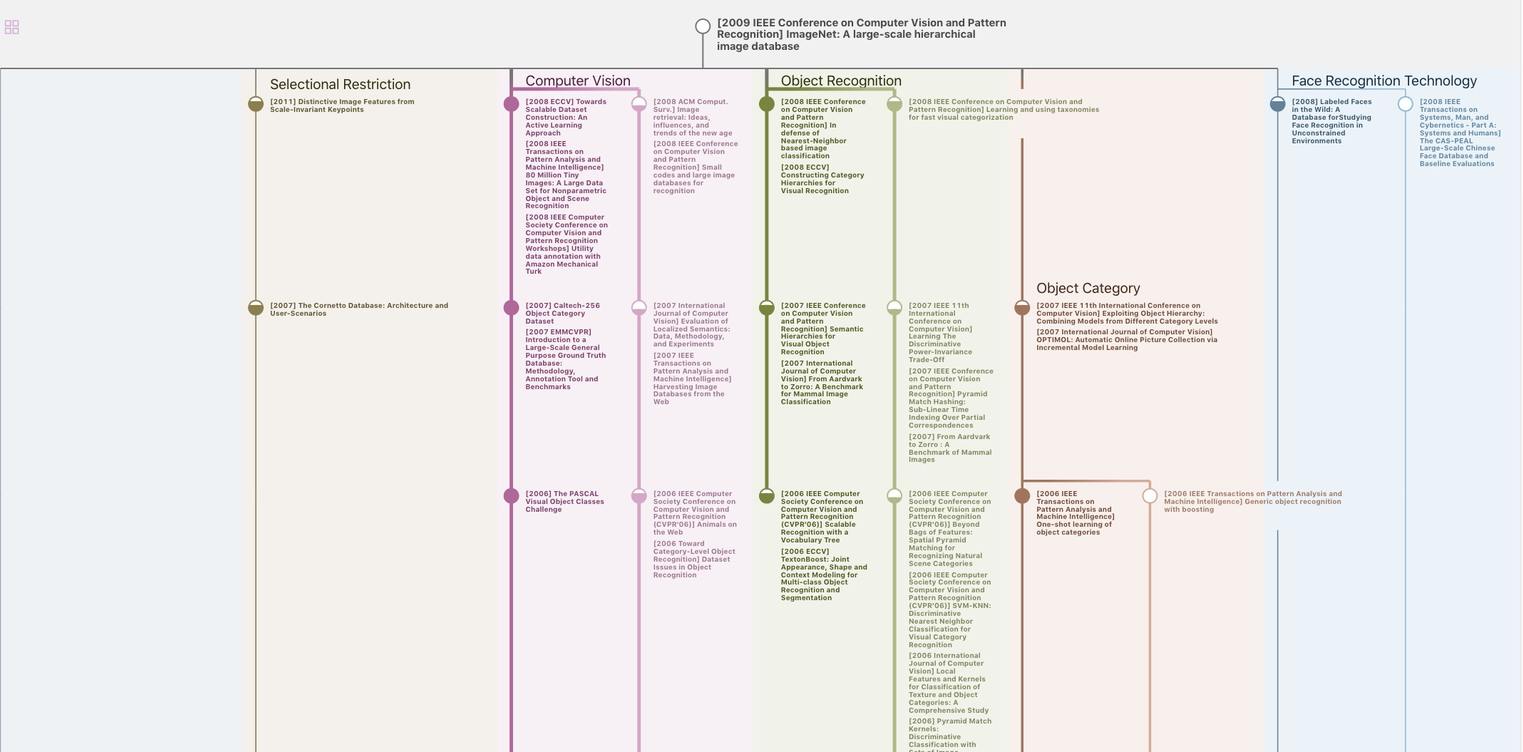
生成溯源树,研究论文发展脉络
Chat Paper
正在生成论文摘要