Instance Segmentation of Unlabeled Modalities via Cyclic Segmentation GAN
arxiv(2022)
摘要
Instance segmentation for unlabeled imaging modalities is a challenging but essential task as collecting expert annotation can be expensive and time-consuming. Existing works segment a new modality by either deploying a pre-trained model optimized on diverse training data or conducting domain translation and image segmentation as two independent steps. In this work, we propose a novel Cyclic Segmentation Generative Adversarial Network (CySGAN) that conducts image translation and instance segmentation jointly using a unified framework. Besides the CycleGAN losses for image translation and supervised losses for the annotated source domain, we introduce additional self-supervised and segmentation-based adversarial objectives to improve the model performance by leveraging unlabeled target domain images. We benchmark our approach on the task of 3D neuronal nuclei segmentation with annotated electron microscopy (EM) images and unlabeled expansion microscopy (ExM) data. Our CySGAN outperforms both pretrained generalist models and the baselines that sequentially conduct image translation and segmentation. Our implementation and the newly collected, densely annotated ExM nuclei dataset, named NucExM, are available at https://connectomics-bazaar.github.io/proj/CySGAN/index.html.
更多查看译文
关键词
cyclic segmentation,unlabeled modalities,gan
AI 理解论文
溯源树
样例
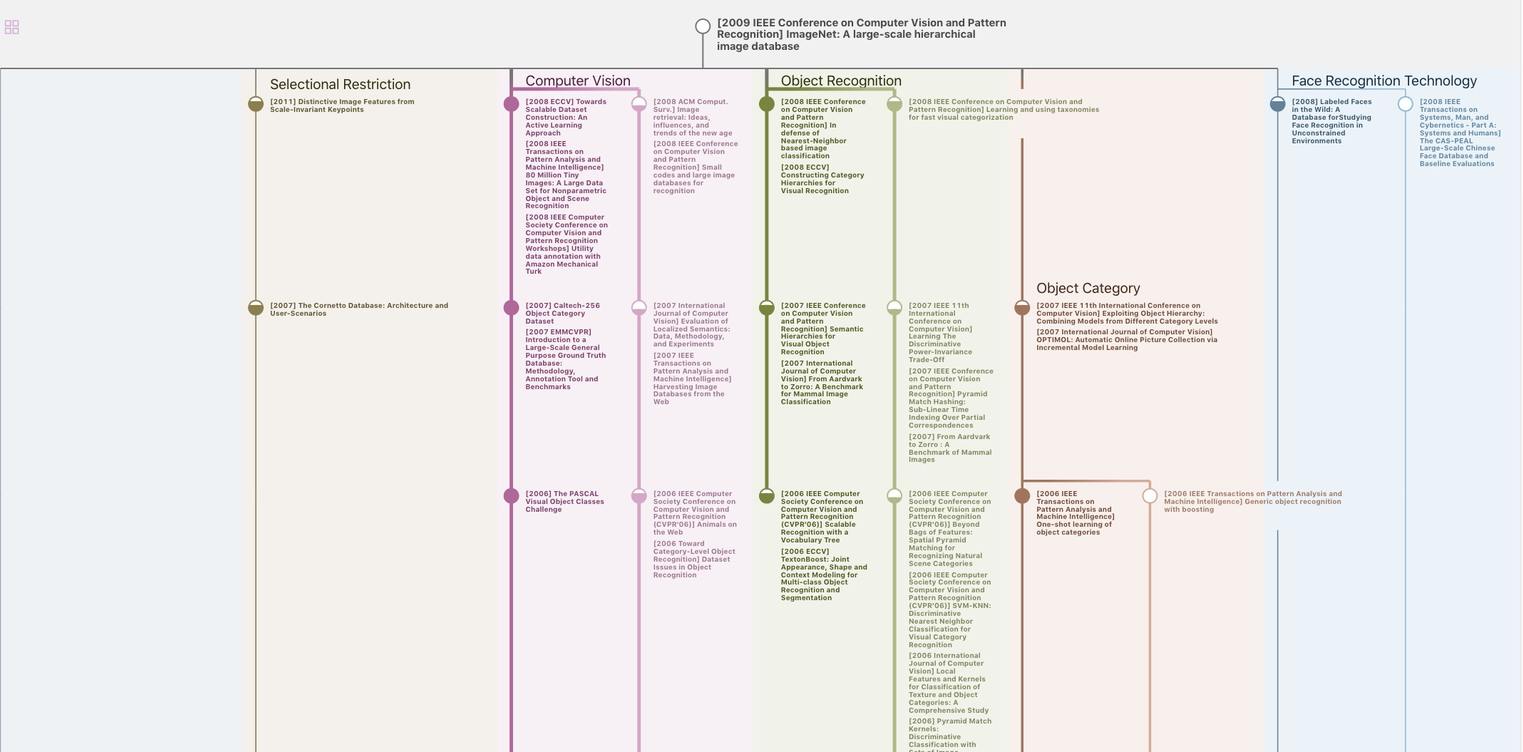
生成溯源树,研究论文发展脉络
Chat Paper
正在生成论文摘要