The Self-Optimal-Transport Feature Transform
arxiv(2022)
摘要
The Self-Optimal-Transport (SOT) feature transform is designed to upgrade the set of features of a data instance to facilitate downstream matching or grouping related tasks. The transformed set encodes a rich representation of high order relations between the instance features. Distances between transformed features capture their direct original similarity and their third party agreement regarding similarity to other features in the set. A particular min-cost-max-flow fractional matching problem, whose entropy regularized version can be approximated by an optimal transport (OT) optimization, results in our transductive transform which is efficient, differentiable, equivariant, parameterless and probabilistically interpretable. Empirically, the transform is highly effective and flexible in its use, consistently improving networks it is inserted into, in a variety of tasks and training schemes. We demonstrate its merits through the problem of unsupervised clustering and its efficiency and wide applicability for few-shot-classification, with state-of-the-art results, and large-scale person re-identification.
更多查看译文
关键词
self-optimal-transport
AI 理解论文
溯源树
样例
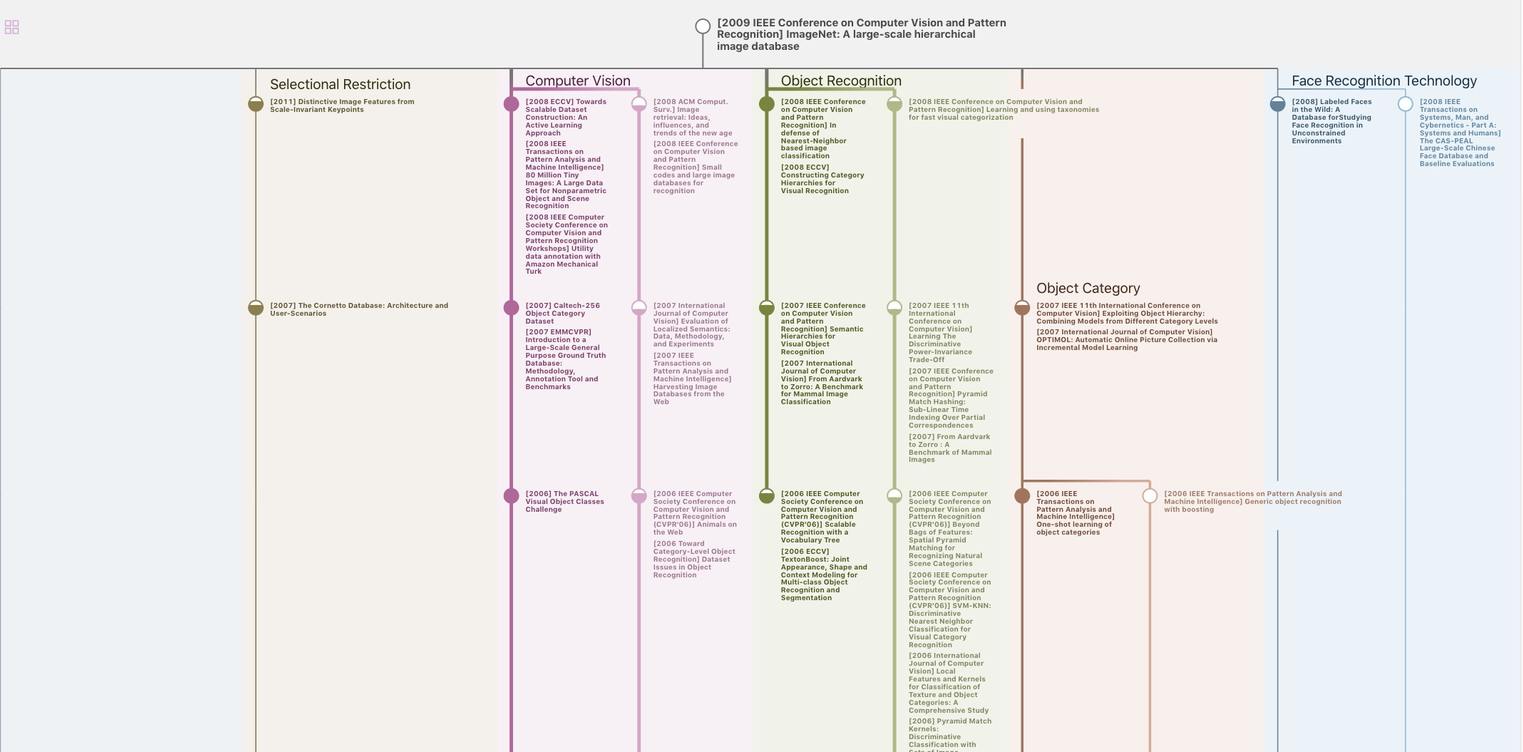
生成溯源树,研究论文发展脉络
Chat Paper
正在生成论文摘要