VALUE: Understanding Dialect Disparity in NLU
PROCEEDINGS OF THE 60TH ANNUAL MEETING OF THE ASSOCIATION FOR COMPUTATIONAL LINGUISTICS (ACL 2022), VOL 1: (LONG PAPERS)(2022)
摘要
English Natural Language Understanding (NLU) systems have achieved great performances and even outperformed humans on benchmarks like GLUE and SuperGLUE. However, these benchmarks contain only textbook Standard American English (SAE). Other dialects have been largely overlooked in the NLP community. This leads to biased and inequitable NLU systems that serve only a sub-population of speakers. To understand disparities in current models and to facilitate more dialect-competent NLU systems, we introduce the VernAcular Language Understanding Evaluation (VALUE) benchmark, a challenging variant of GLUE that we created with a set of lexical and morphosyntactic transformation rules. In this initial release (V.1), we construct rules for 11 features of African American Vernacular English (AAVE), and we recruit fluent AAVE speakers to validate each feature transformation via linguistic acceptability judgments in a participatory design manner. Experiments show that these new dialectal features can lead to a drop in model performance.
更多查看译文
关键词
dialect disparity,nlu,value
AI 理解论文
溯源树
样例
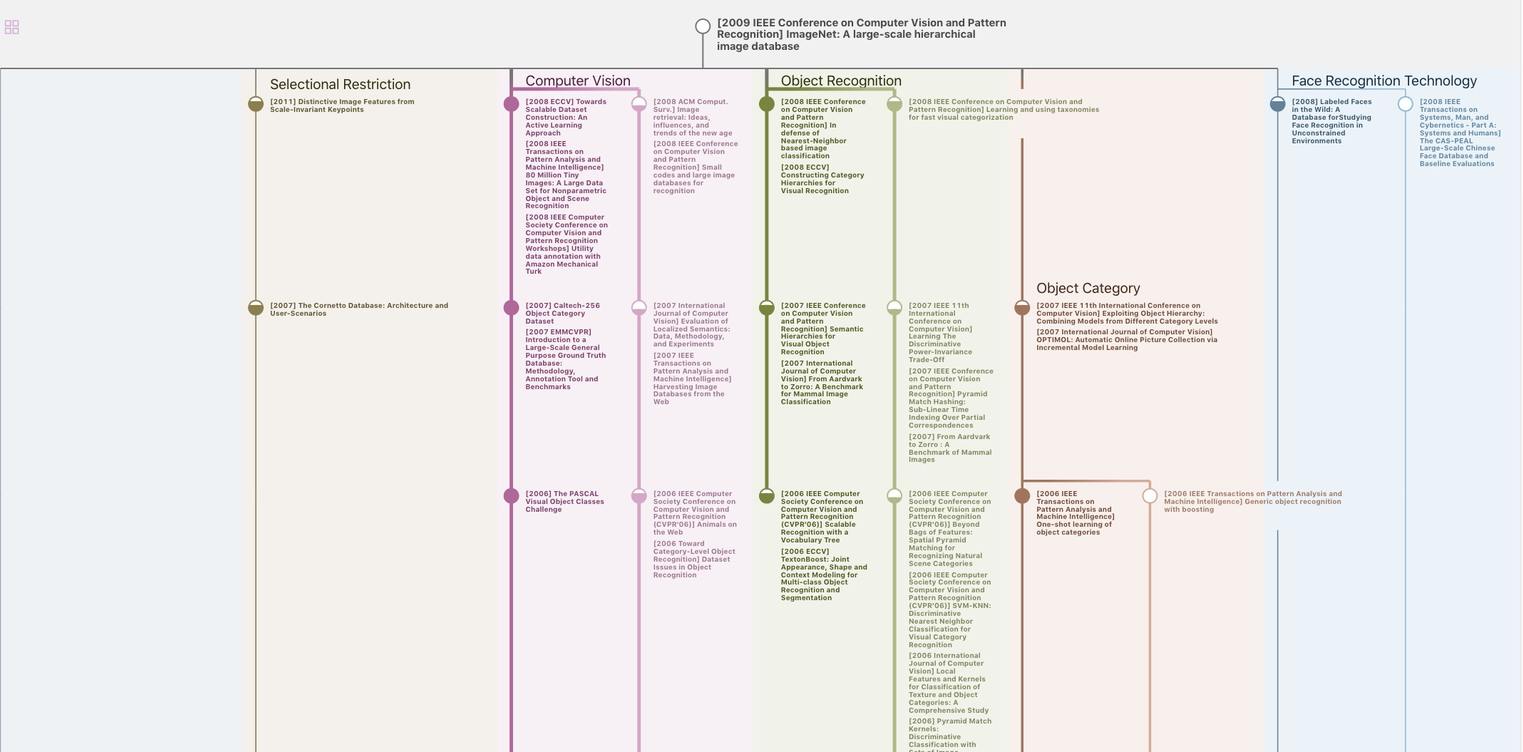
生成溯源树,研究论文发展脉络
Chat Paper
正在生成论文摘要