Generative Enriched Sequential Learning (ESL) Approach for Molecular Design via Augmented Domain Knowledge.
Canadian Conference on Artificial Intelligence (AI)(2022)
摘要
Deploying generative machine learning techniques to generate novel chemical structures based on molecular fingerprint representation has been well established in molecular design. Typically, sequential learning (SL) schemes such as hidden Markov models (HMM) and, more recently, in the sequential deep learning context, recurrent neural network (RNN) and long short-term memory (LSTM) were used extensively as generative models to discover unprecedented molecules. To this end, emission probability between two states of atoms plays a central role without considering specific chemical or physical properties. Lack of supervised domain knowledge can mislead the learning procedure to be relatively biased to the prevalent molecules observed in the training data that are not necessarily of interest. We alleviated this drawback by augmenting the training data with domain knowledge, e.g. quantitative estimates of the drug-likeness score (QEDs). As such, our experiments demonstrated that with this subtle trick called enriched sequential learning (ESL), specific patterns of particular interest can be learnt better, which led to generating de novo molecules with ameliorated QEDs.
更多查看译文
关键词
molecular
AI 理解论文
溯源树
样例
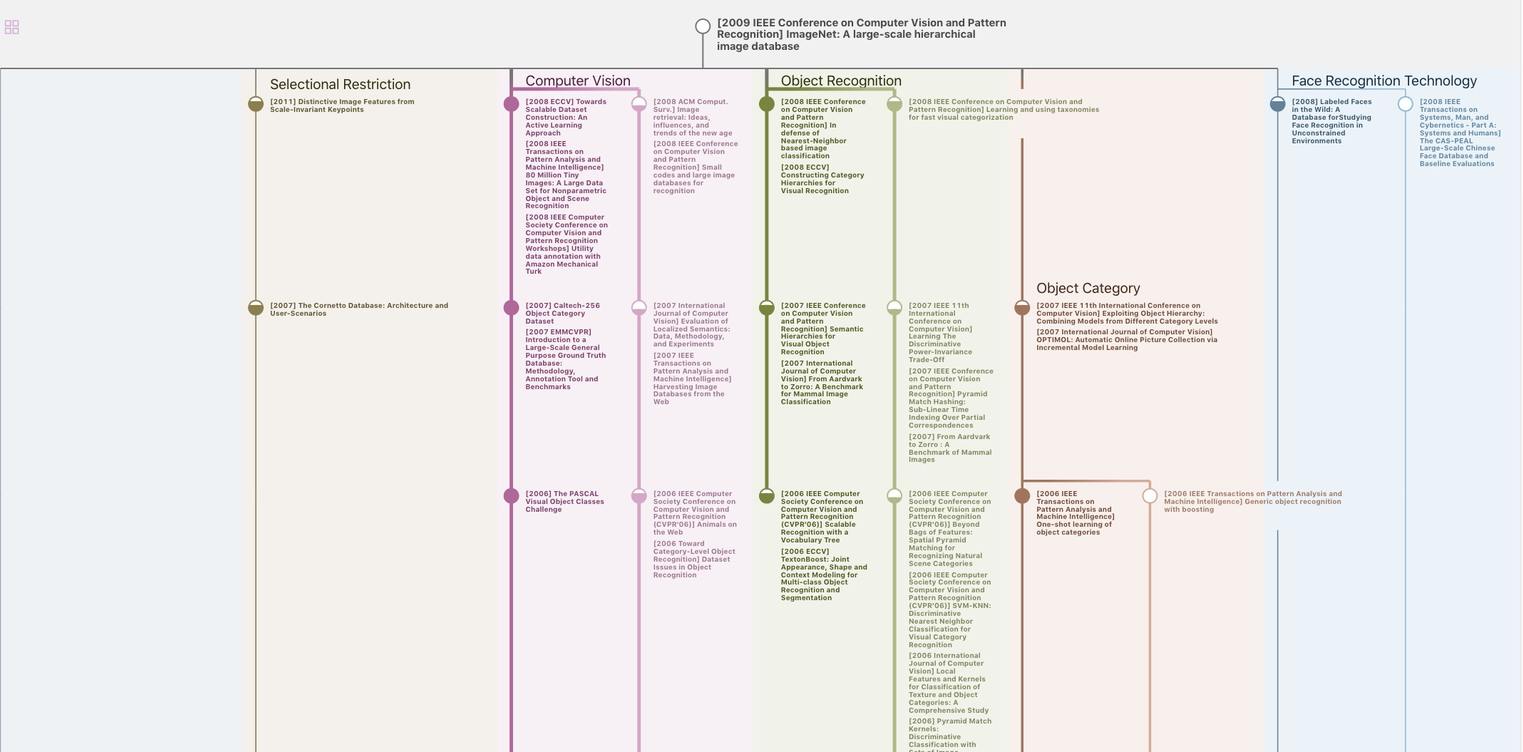
生成溯源树,研究论文发展脉络
Chat Paper
正在生成论文摘要