Modelling sea surface wind measurements on Australia’s North-West Shelf
Ocean Engineering(2022)
摘要
The environment experienced by offshore industries is inherently volatile, providing a strong impetus for accurate modelling and prediction of meteorological and oceanographic conditions. Numerical forecasts, obtained from physics-based deterministic models, are informative yet imperfect in their prediction, motivating the quantification of their associated uncertainty. To achieve this, we present a Bayesian hierarchical model that updates numerical forecasts of metocean conditions and examines their associated error structure. This involves a linear regression to remove systematic forecast biases and the use of time series techniques, namely autoregressive and generalised autoregressive conditional heteroscedasticity models, to remove residual time-evolving error structure. We find that the hierarchical statistical model outperforms a numerical weather prediction model in terms of mean squared error and continuous ranked probability score, a metric which is able to account for uncertainty information contained in probabilistic forecasts. While the method is generalisable to a range of metocean conditions with an available set of past measurements and numerical forecasts, we present our findings using a case study of sea surface wind data. The data is collected from a location on Australia’s North-West Shelf, a region home to an extent of offshore operations and often characterised by extreme unexpected metocean conditions.
更多查看译文
关键词
Probabilistic forecasts,Uncertainty quantification,Bayesian statistics,Wind forecasting,Autoregression,GARCH
AI 理解论文
溯源树
样例
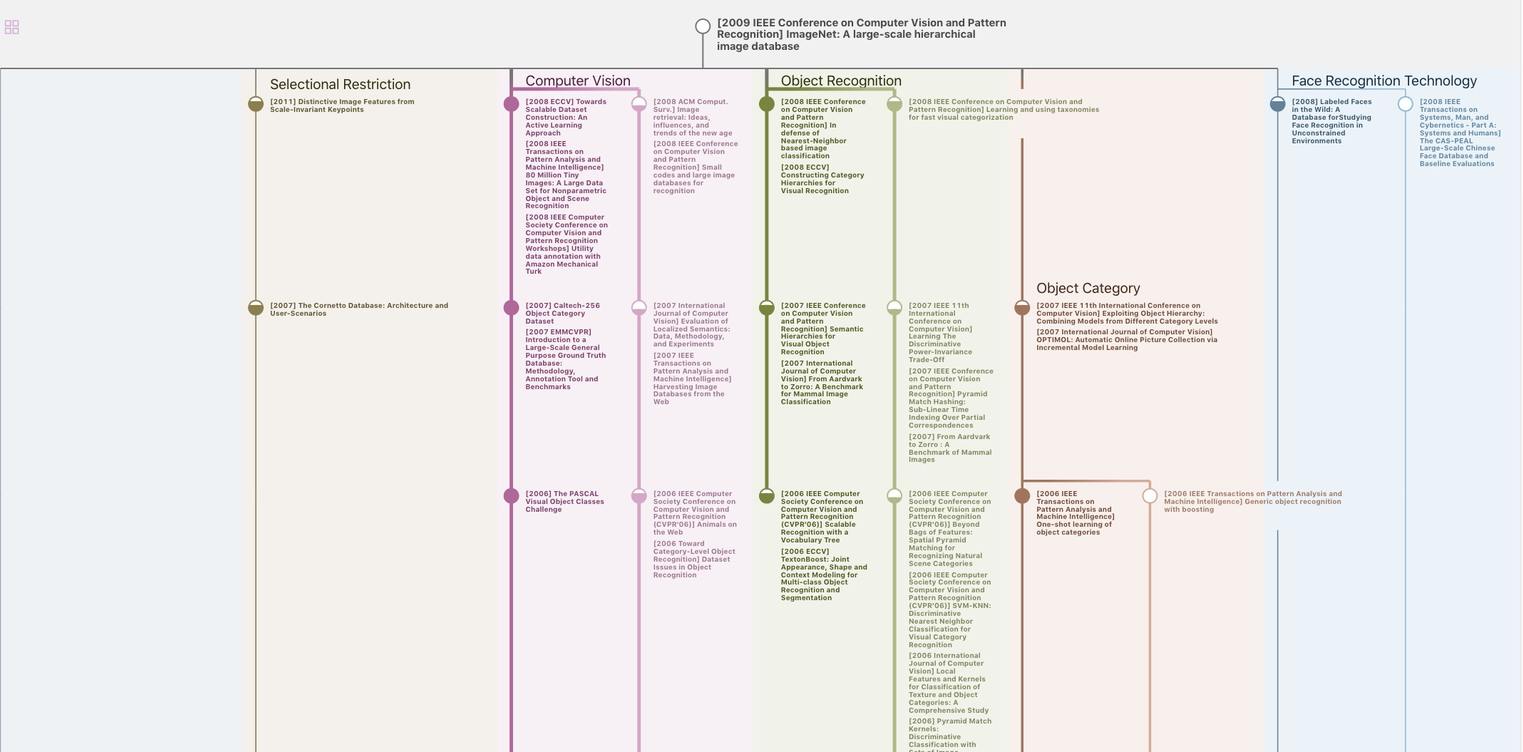
生成溯源树,研究论文发展脉络
Chat Paper
正在生成论文摘要