Large-scale nonconvex optimization: randomization, gap estimation, and numerical resolution
SIAM JOURNAL ON OPTIMIZATION(2023)
摘要
We address a large-scale and nonconvex optimization problem, involving an aggregative term. This term can be interpreted as the sum of the contributions of N agents to some common good, with N large. We investigate a relaxation of this problem, obtained by randomization. The relaxation gap is proved to converge to zeros as N goes to infinity, independently of the dimension of the aggregate. We propose a stochastic method to construct an approximate minimizer of the original problem, given an approximate solution of the randomized problem. McDiarmid's concentration inequality is used to quantify the probability of success of the method. We consider the Frank-Wolfe (FW) algorithm for the resolution of the randomized problem. Each iteration of the algorithm requires to solve a subproblem which can be decomposed into N independent optimization problems. A sublinear convergence rate is obtained for the FW algorithm. In order to handle the memory overflow problem possibly caused by the FW algorithm, we propose a stochastic FW) algorithm, which ensures the convergence in both expectation and probability senses. Numerical experiments on a mixed-integer quadratic program illustrate the efficiency of the method.
更多查看译文
关键词
large-scale and nonconvex optimization,aggregative optimization,relaxation,decentralization,Frank Wolfe algorithm,concentration inequalities,multiagent optimization,privacy-preserving methods
AI 理解论文
溯源树
样例
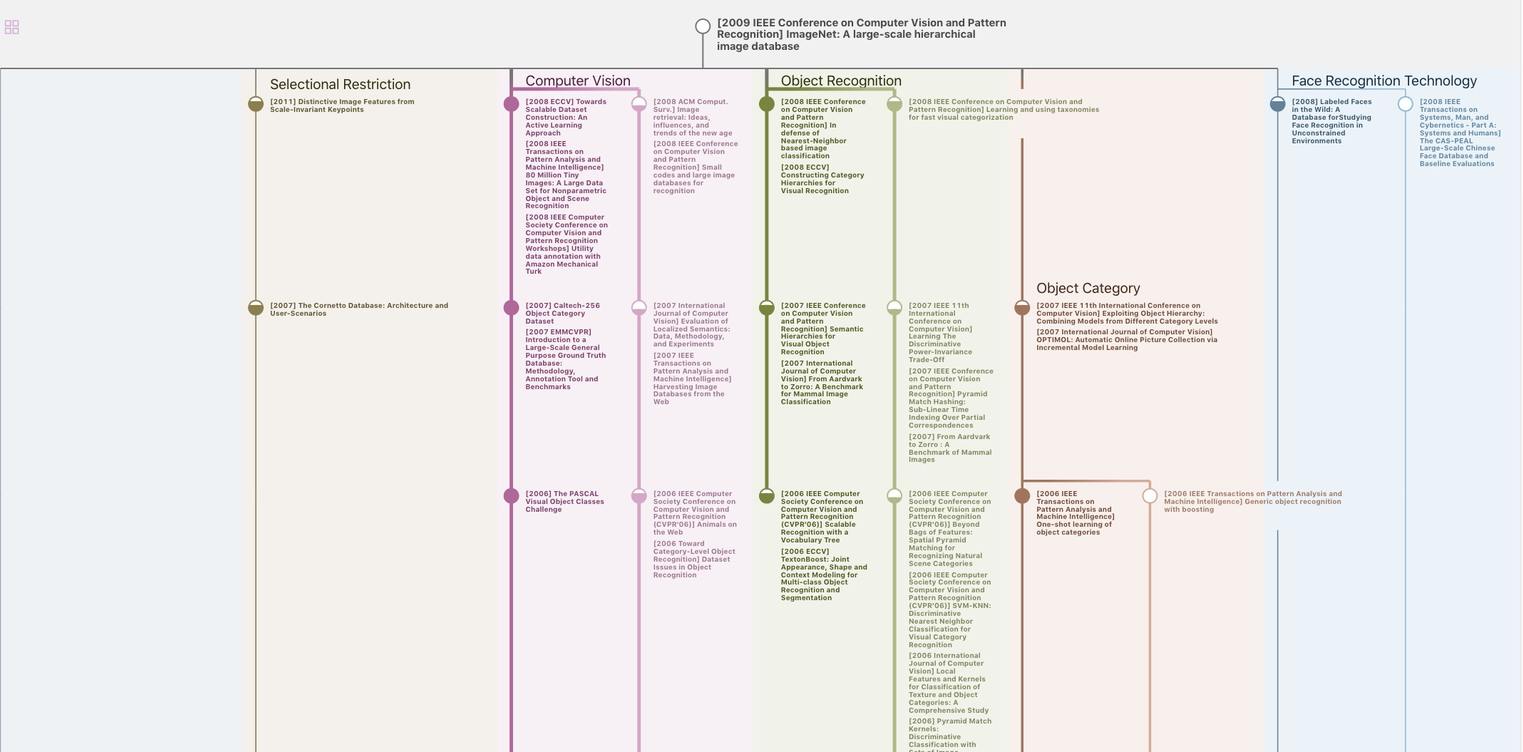
生成溯源树,研究论文发展脉络
Chat Paper
正在生成论文摘要