Test Against High-Dimensional Uncertainties: Accelerated Evaluation of Autonomous Vehicles with Deep Importance Sampling
ArXiv(2022)
摘要
Evaluating the performance of autonomous vehicles (AV) and their complex subsystems to high precision under naturalistic circumstances remains a challenge, especially when failure or dangerous cases are rare. Rarity does not only require an enormous sample size for a naive method to achieve high confidence estimation, but it also causes dangerous underestimation of the true failure rate and it is extremely hard to detect. Meanwhile, the state-of-the-art approach that comes with a correctness guarantee can only compute an upper bound for the failure rate under certain conditions, which could limit its practical uses. In this work, we present Deep Importance Sampling (Deep IS) framework that utilizes a deep neural network to obtain an efficient IS that is on par with the state-of-the-art, capable of reducing the required sample size 43 times smaller than the naive sampling method to achieve 10% relative error and while producing an estimate that is much less conservative. Our high-dimensional experiment estimating the misclassification rate of one of the state-of-the-art traffic sign classifiers further reveals that this efficiency still holds true even when the target is very small, achieving over 600 times efficiency boost. This highlights the potential of Deep IS in providing a precise estimate even against high-dimensional uncertainties.
更多查看译文
关键词
autonomous vehicles,accelerated evaluation,sampling,high-dimensional
AI 理解论文
溯源树
样例
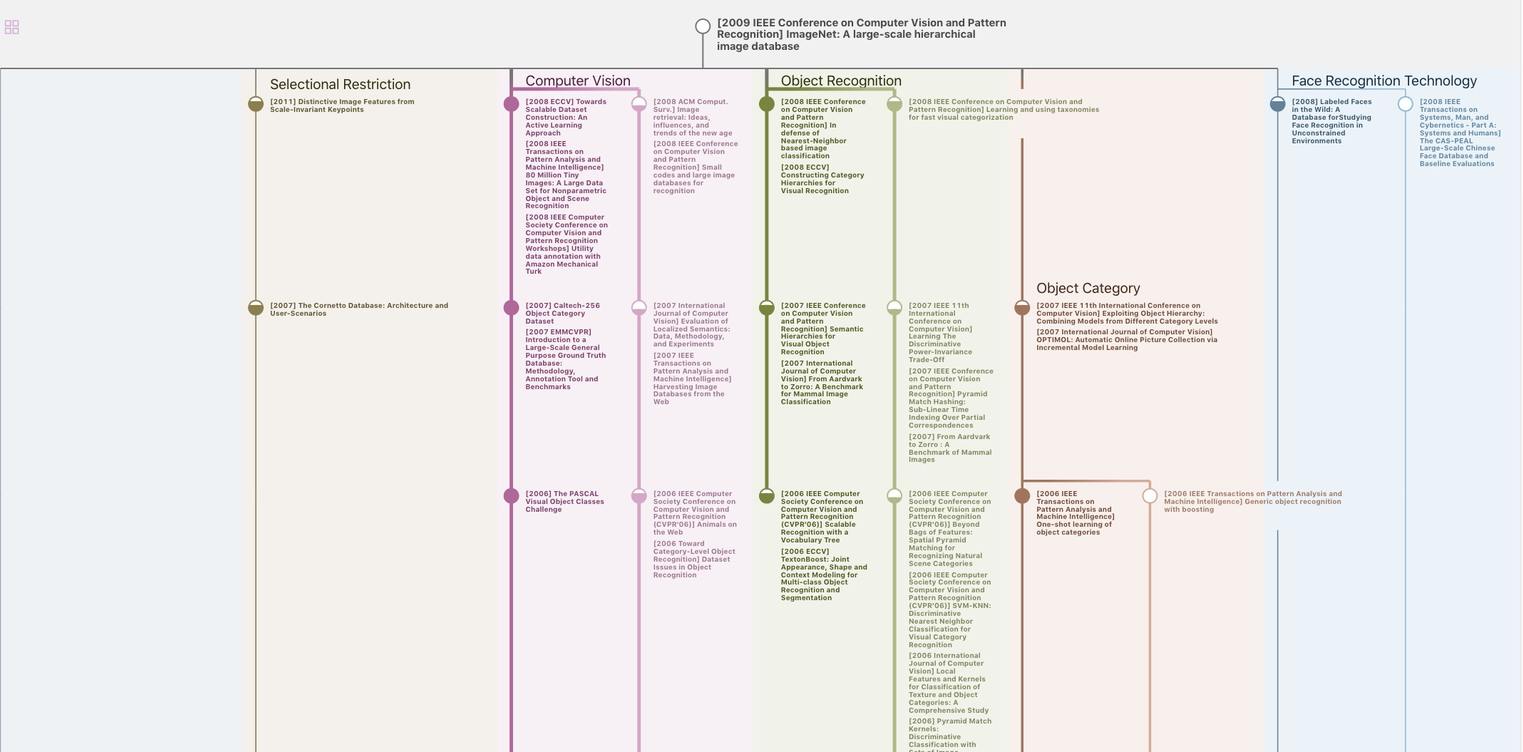
生成溯源树,研究论文发展脉络
Chat Paper
正在生成论文摘要