Model Based Meta Learning of Critics for Policy Gradients
arxiv(2022)
摘要
Being able to seamlessly generalize across different tasks is fundamental for robots to act in our world. However, learning representations that generalize quickly to new scenarios is still an open research problem in reinforcement learning. In this paper we present a framework to meta-learn the critic for gradient-based policy learning. Concretely, we propose a model-based bi-level optimization algorithm that updates the critics parameters such that the policy that is learned with the updated critic gets closer to solving the meta-training tasks. We illustrate that our algorithm leads to learned critics that resemble the ground truth Q function for a given task. Finally, after meta-training, the learned critic can be used to learn new policies for new unseen task and environment settings via model-free policy gradient optimization, without requiring a model. We present results that show the generalization capabilities of our learned critic to new tasks and dynamics when used to learn a new policy in a new scenario.
更多查看译文
关键词
model based meta learning,critics,policy
AI 理解论文
溯源树
样例
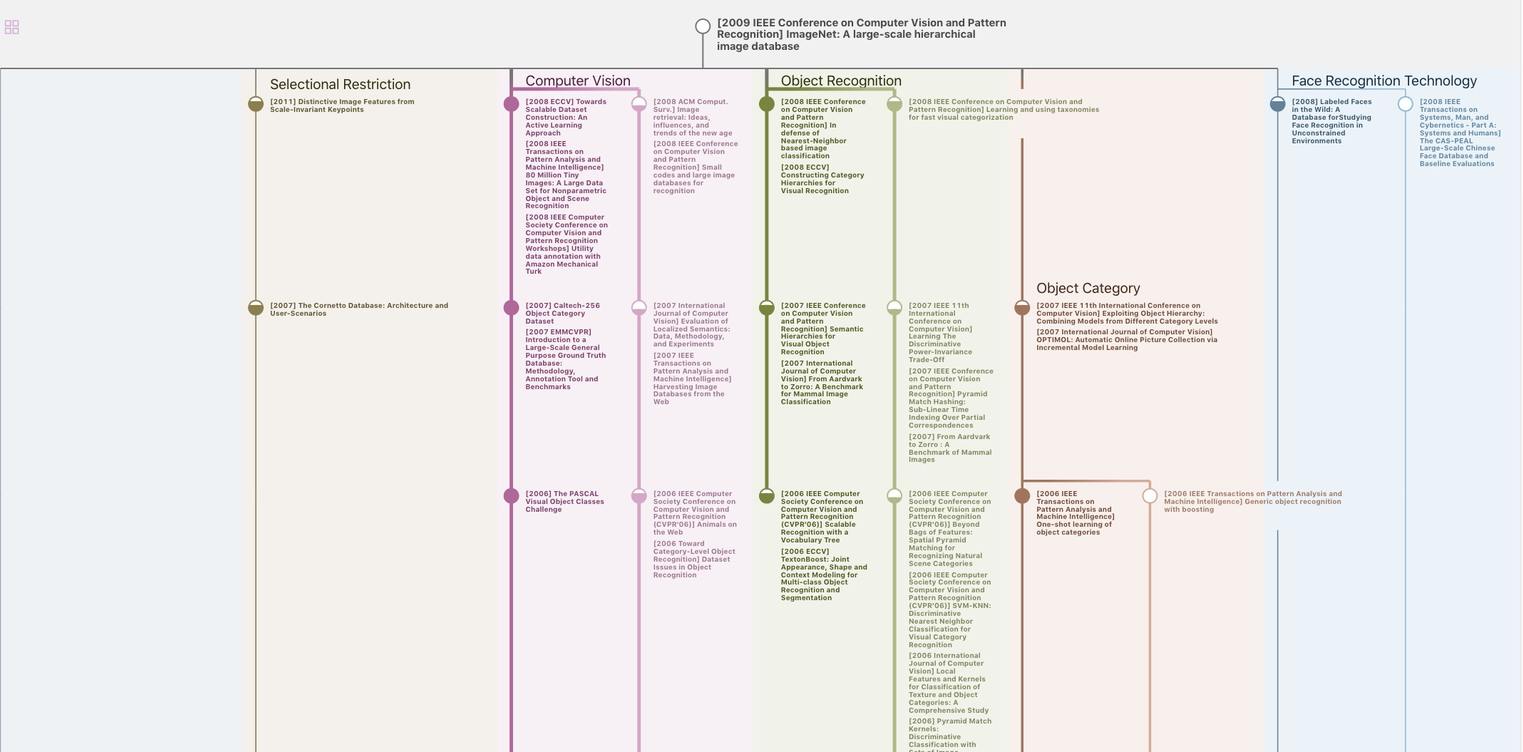
生成溯源树,研究论文发展脉络
Chat Paper
正在生成论文摘要