ELECRec: Training Sequential Recommenders as Discriminators
SIGIR '22: Proceedings of the 45th International ACM SIGIR Conference on Research and Development in Information Retrieval(2022)
摘要
Sequential recommendation is often considered as a generative task, i.e., training a sequential encoder to generate the next item of a user's interests based on her historical interacted items. Despite their prevalence, these methods usually require training with more meaningful samples to be effective, which otherwise will lead to a poorly trained model. In this work, we propose to train the sequential recommenders as discriminators rather than generators. Instead of predicting the next item, our method trains a discriminator to distinguish if a sampled item is a 'real' target item or not. A generator, as an auxiliary model, is trained jointly with the discriminator to sample plausible alternative next items and will be thrown out after training. The trained discriminator is considered as the final SR model and denoted as \modelname. Experiments conducted on four datasets demonstrate the effectiveness and efficiency of the proposed approach.
更多查看译文
关键词
Sequential Recommendation, Discriminate Modeling, Learning Efficiency
AI 理解论文
溯源树
样例
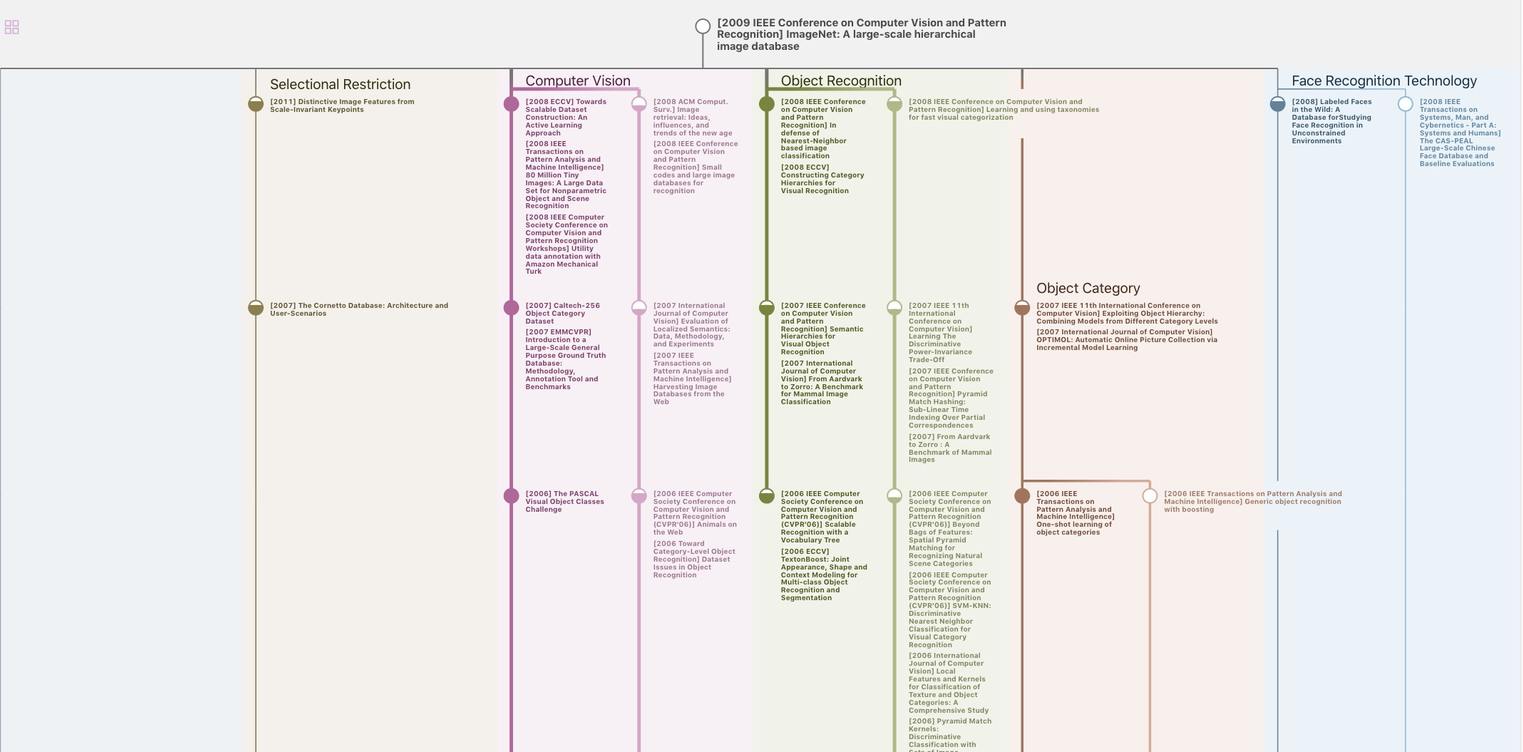
生成溯源树,研究论文发展脉络
Chat Paper
正在生成论文摘要