Improving Monocular Visual Odometry Using Learned Depth
IEEE Transactions on Robotics(2022)
摘要
Monocular visual odometry (VO) is an important task in robotics and computer vision. Thus far, how to build accurate and robust monocular VO systems that can work well in diverse scenarios remains largely unsolved. In this article, we propose a framework to exploit monocular depth estimation for improving VO. The core of our framework is a monocular depth estimation module with a strong generalization capability for diverse scenes. It consists of two separate working modes to assist the localization and mapping. With a single monocular image input, the depth estimation module predicts a relative depth to help the localization module on improving the accuracy. With a sparse depth map and an RGB image input, the depth estimation module can generate accurate scale-consistent depth for dense mapping. Compared with current learning-based VO methods, our method demonstrates a stronger generalization ability to diverse scenes. More significantly, our framework is able to boost the performances of existing geometry-based VO methods by a large margin.
更多查看译文
关键词
Monocular depth estimation,simultaneous localization and mapping (SLAM),visual odometry (VO)
AI 理解论文
溯源树
样例
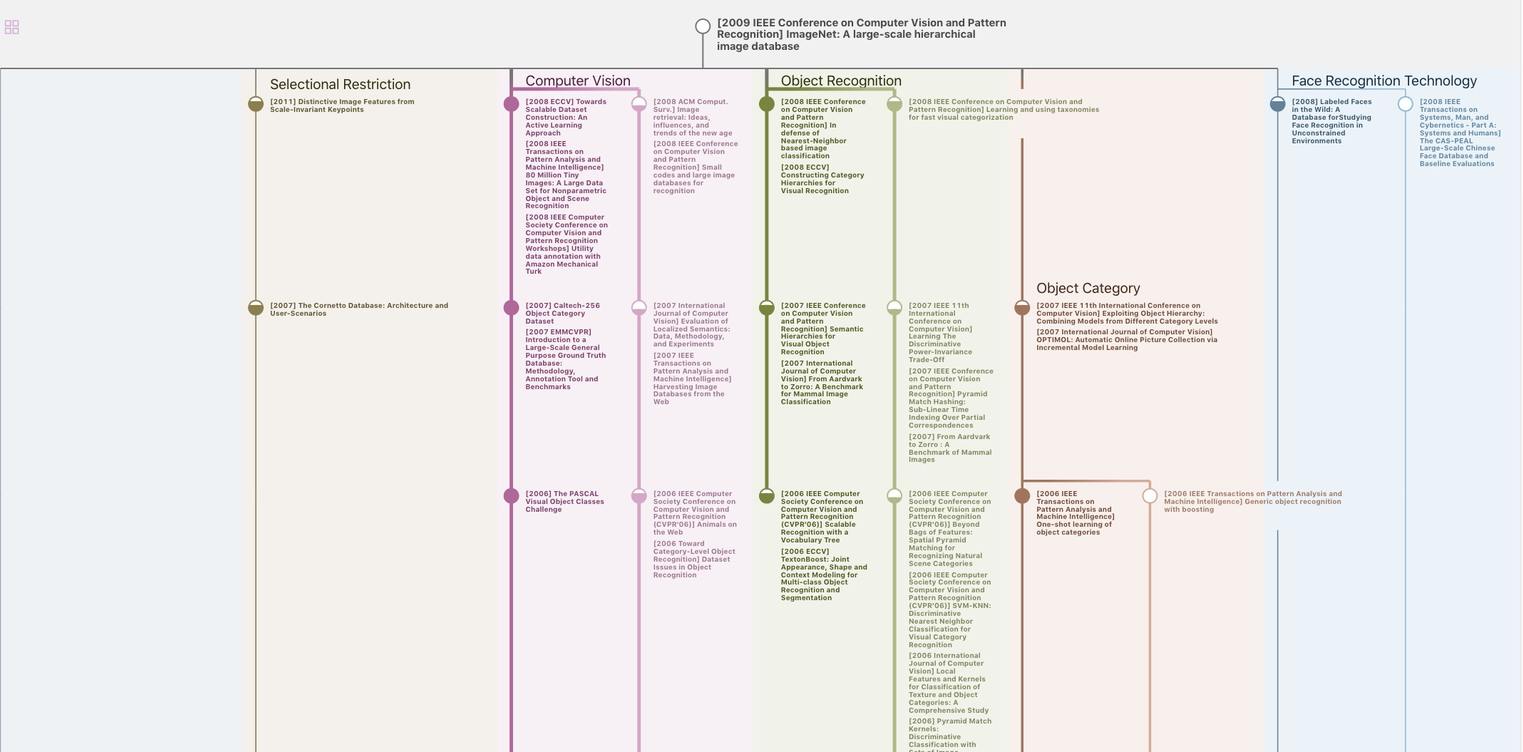
生成溯源树,研究论文发展脉络
Chat Paper
正在生成论文摘要