Unbiased Top-k Learning to Rank with Causal Likelihood Decomposition
ANNUAL INTERNATIONAL ACM SIGIR CONFERENCE ON RESEARCH AND DEVELOPMENT IN INFORMATION RETRIEVAL IN THE ASIA PACIFIC REGION, SIGIR-AP 2023(2023)
摘要
Unbiased learning to rank methods have been proposed to address biases in search ranking. These biases, known as position bias and sample selection bias, often occur simultaneously in real applications. Existing approaches either tackle these biases separately or treat them as identical, leading to incomplete elimination of both biases. This paper employs a causal graph approach to investigate the mechanisms and interplay between position bias and sample selection bias. The analysis reveals that position bias is a common confounder bias, while sample selection bias falls under the category of collider bias. These biases collectively introduce a cascading process that leads to biased clicks. Based on our analysis, we propose Causal Likelihood Decomposition (CLD), a unified method that effectively mitigates both biases in top-k learning to rank. CLD removes position bias by leveraging propensity scores and then decomposes the likelihood of selection biased data into sample selection bias term and relevance term. By maximizing the overall log-likelihood function, we obtain an unbiased ranking model from the relevance term. We also extend CLD to pairwise neural ranking. Extensive experiments demonstrate that CLD and its pairwise neural extension outperform baseline methods by effectively mitigating both position bias and sample selection bias. The robustness of CLD is further validated through empirical studies considering variations in bias severity and click noise.
更多查看译文
关键词
unbiased learning to rank,position bias,sample selection bias
AI 理解论文
溯源树
样例
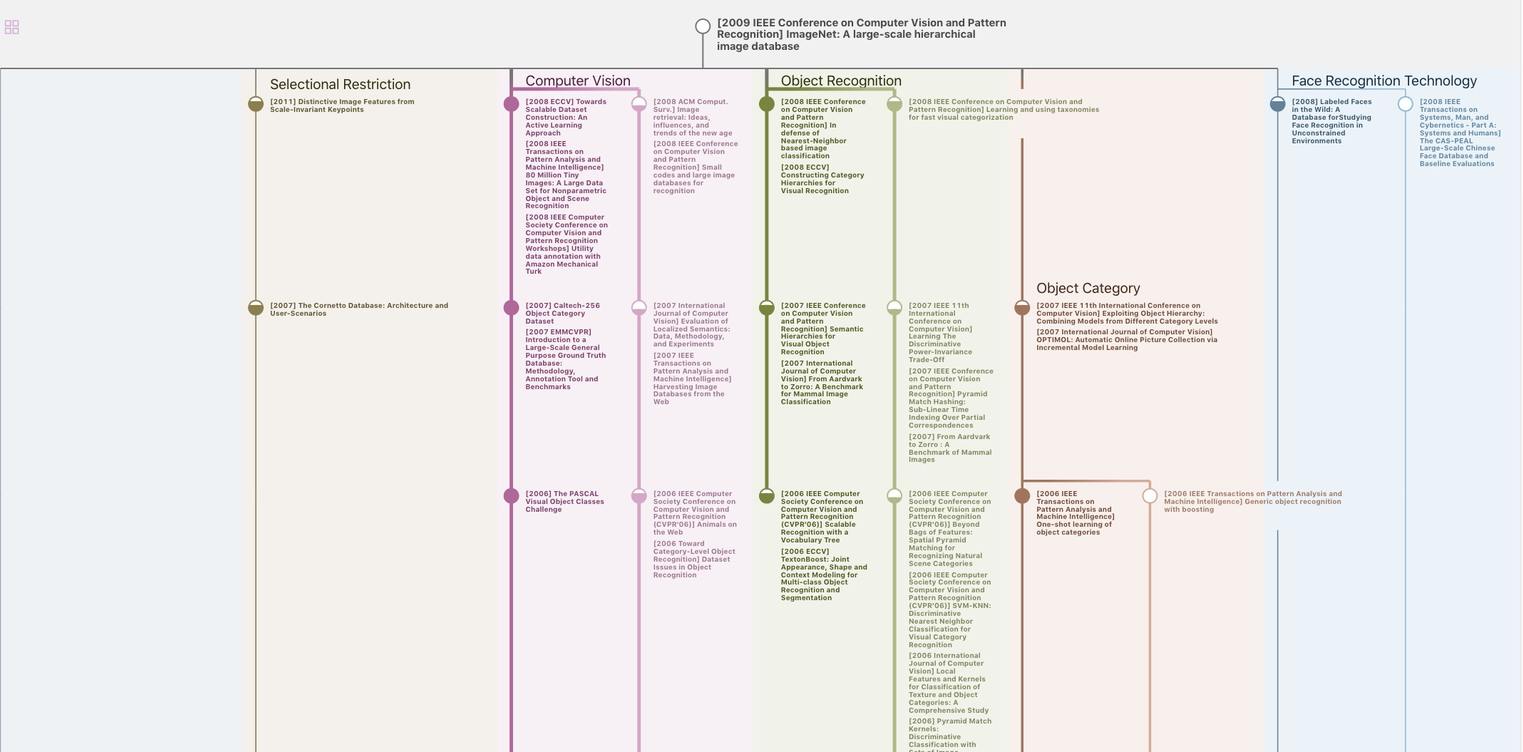
生成溯源树,研究论文发展脉络
Chat Paper
正在生成论文摘要