Field-Based Physical Inference From Peculiar Velocity Tracers
arxiv(2022)
摘要
We present a Bayesian hierarchical modelling approach to reconstruct the initial cosmic matter density field constrained by peculiar velocity observations. As our approach features a model for the gravitational evolution of dark matter to connect the initial conditions to late-time observations, it reconstructs the final density and velocity fields as natural byproducts. We implement this field-based physical inference approach by adapting the Bayesian Origin Reconstruction from Galaxies (BORG) algorithm, which explores the high-dimensional posterior through the use of Hamiltonian Monte Carlo sampling. We test the self-consistency of the method using random sets of mock tracers, and assess its accuracy in a more complex scenario where peculiar velocity tracers are non-linearly evolved mock haloes. We find that our framework self-consistently infers the initial conditions, density and velocity fields, and shows some robustness to model mis-specification. As compared to the state-of-the-art approach of constrained Gaussian random fields/Wiener filtering, our method produces more accurate final density and velocity field reconstructions. It also allows us to constrain the initial conditions by peculiar velocity observations, complementing in this aspect previous field-based approaches based on other cosmological observables.
更多查看译文
关键词
methods: data analysis, galaxies: distances and redshifts, large-scale structure of Universe
AI 理解论文
溯源树
样例
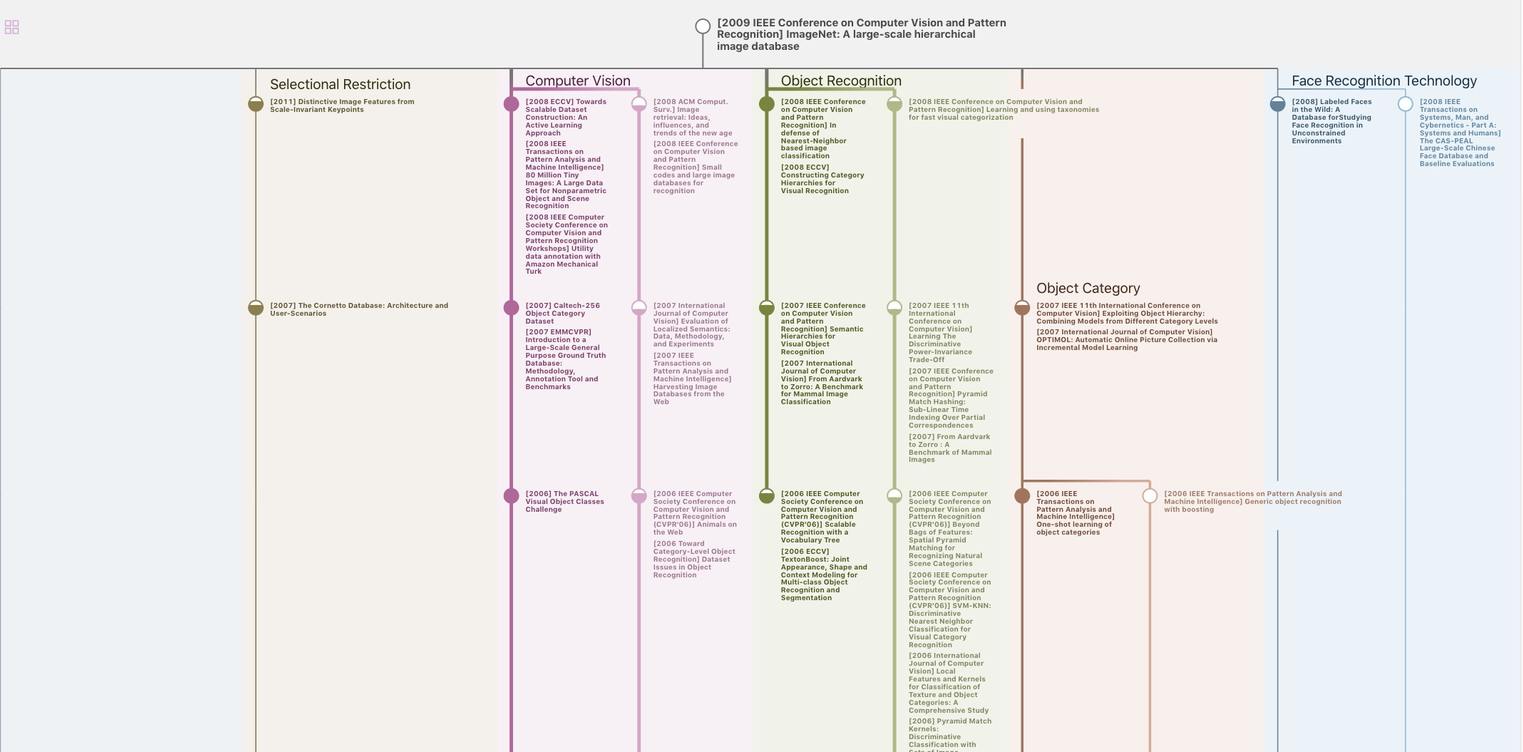
生成溯源树,研究论文发展脉络
Chat Paper
正在生成论文摘要