Development and validation of a composite AI model for the diagnosis of levator ani muscle avulsion
European Radiology(2022)
摘要
Objective To assess the feasibility and reliability of a composite AI model for the diagnosis of levator ani muscle (LAM) avulsion of tomographic ultrasound imaging (TUI). Methods Ultrasonic images of the pelvic floor from a total of 304 patients taken from January 2018 to October 2020 were included. All patients included underwent standardized interviews and transperineal ultrasound (TPUS). Transfer-learning and ensemble-learning methods were adopted to develop the proposed model on the basis of three classic convolutional neural networks (CNN). Confusion matrix (CM) and the ROC statistic were used to assess the effectiveness of the proposed model. Gradient-weighted class activation mappings (Grad-CAMs) were used to help enhance the interpretability of the proposed model. Results Of the 304 patients included, 208 were in the derivation cohort (108 LAM avulsion and 100 normal) and 96 (39 LAM avulsion and 57 normal) were in the validation cohort. The proposed model in LAM avulsion diagnosis outperformed other models and a junior clinician in both the test set of derivation cohort and the validation cohort, with accuracies of 0.95 and 0.81, and AUCs of 0.98 and 0.86, respectively. According to the heatmap of Grad-CAMs, the proposed model mainly localizes areas between the pubic symphysis and the bilateral insertion point of LAM when making a diagnosis, which is exactly the region of interest in clinical practice. Conclusion The proposed model using ultrasonic images of the pelvic floor may be a promising tool in assisting the diagnosis of LAM avulsion in clinical practice. Key Points • First AI–assisted model for levator ani muscle avulsion diagnosis • Diagnosis accuracy of less-experienced clinicians could be improved using the proposed model.
更多查看译文
关键词
Levator ani muscle avulsion, Deep learning, Ultrasonography
AI 理解论文
溯源树
样例
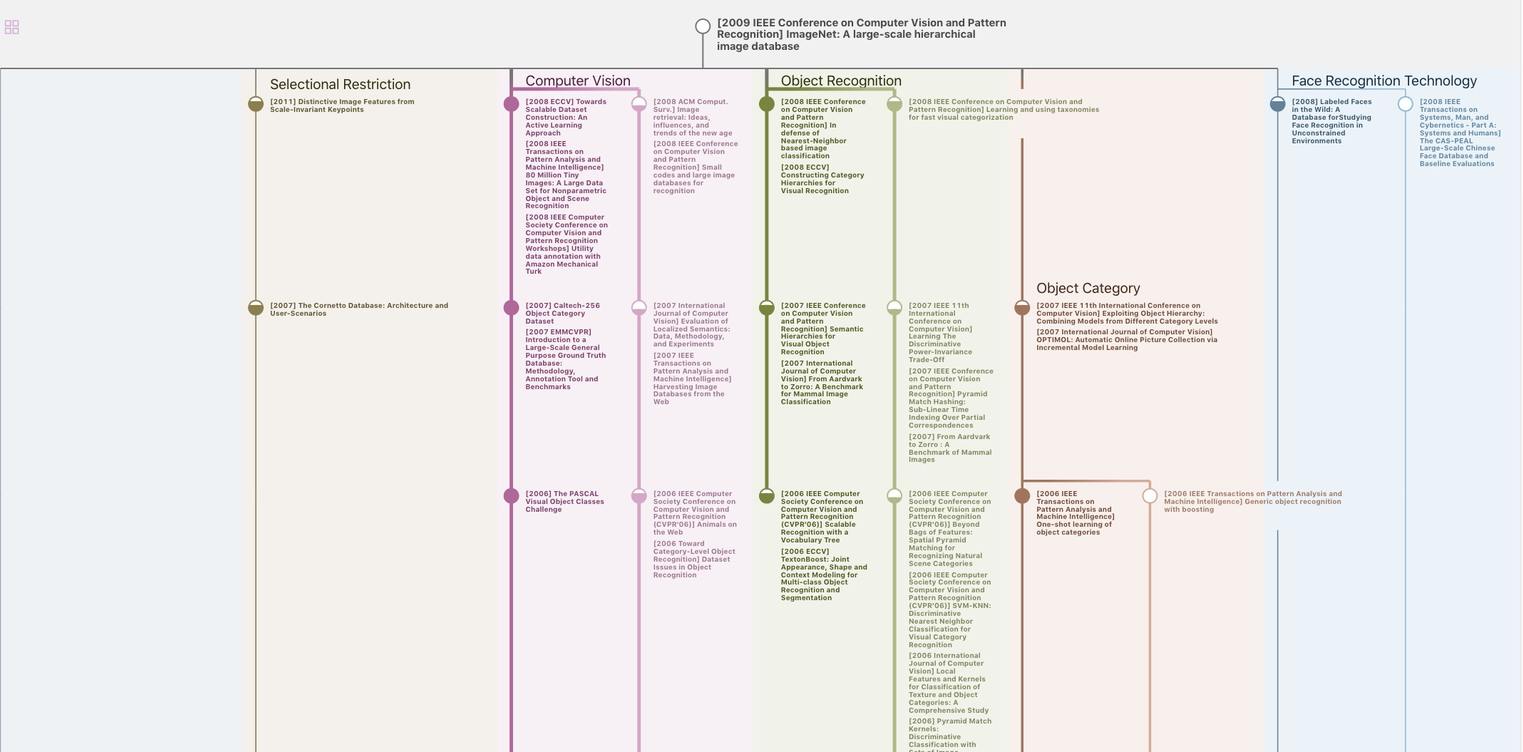
生成溯源树,研究论文发展脉络
Chat Paper
正在生成论文摘要