Comparison of logP and logD correction models trained with public and proprietary data sets
Journal of Computer-Aided Molecular Design(2022)
摘要
In drug discovery, partition and distribution coefficients, log P and log D for octanol/water, are widely used as metrics of the lipophilicity of molecules, which in turn have a strong influence on the bioactivity and bioavailability of potential drugs. There are a variety of established methods, mostly fragment or atom-based, to calculate log P while log D prediction generally relies on calculated log P and p Ka for the estimation of neutral and ionized populations at a given pH. Algorithms such as Clog P have limitations generally leading to systematic errors for chemically related molecules while p Ka estimation is generally more difficult due to the interplay of electronic, inductive and conjugation effects for ionizable moieties. We propose an integrated machine learning QSAR modeling approach to predict log D by training the model with experimental data while using Clog P and p Ka predicted by commercial software as model descriptors. By optimizing the loss function for the Clog D calculated by the software, we build a correction model that incorporates both descriptors from the software and available experimental log D data. Additionally, we calculate log P from the log D model using the software predicted pKa’s. Here, we have trained models using publicly or commercial available log D data to show that this approach can improve on commercial software predictions of lipophilicity. When applied to other log D data sets, this approach extends the domain of applicability of log D and log P predictions over commercial software. Performance of these models favorably compare with models built with a larger set of proprietary log D data.
更多查看译文
关键词
Partition coefficient, Distribution coefficient, LogP
, LogD
, ClogP
, ClogD
, pKa
, BioByte, ChEMBL, Machine learning, QSAR models
AI 理解论文
溯源树
样例
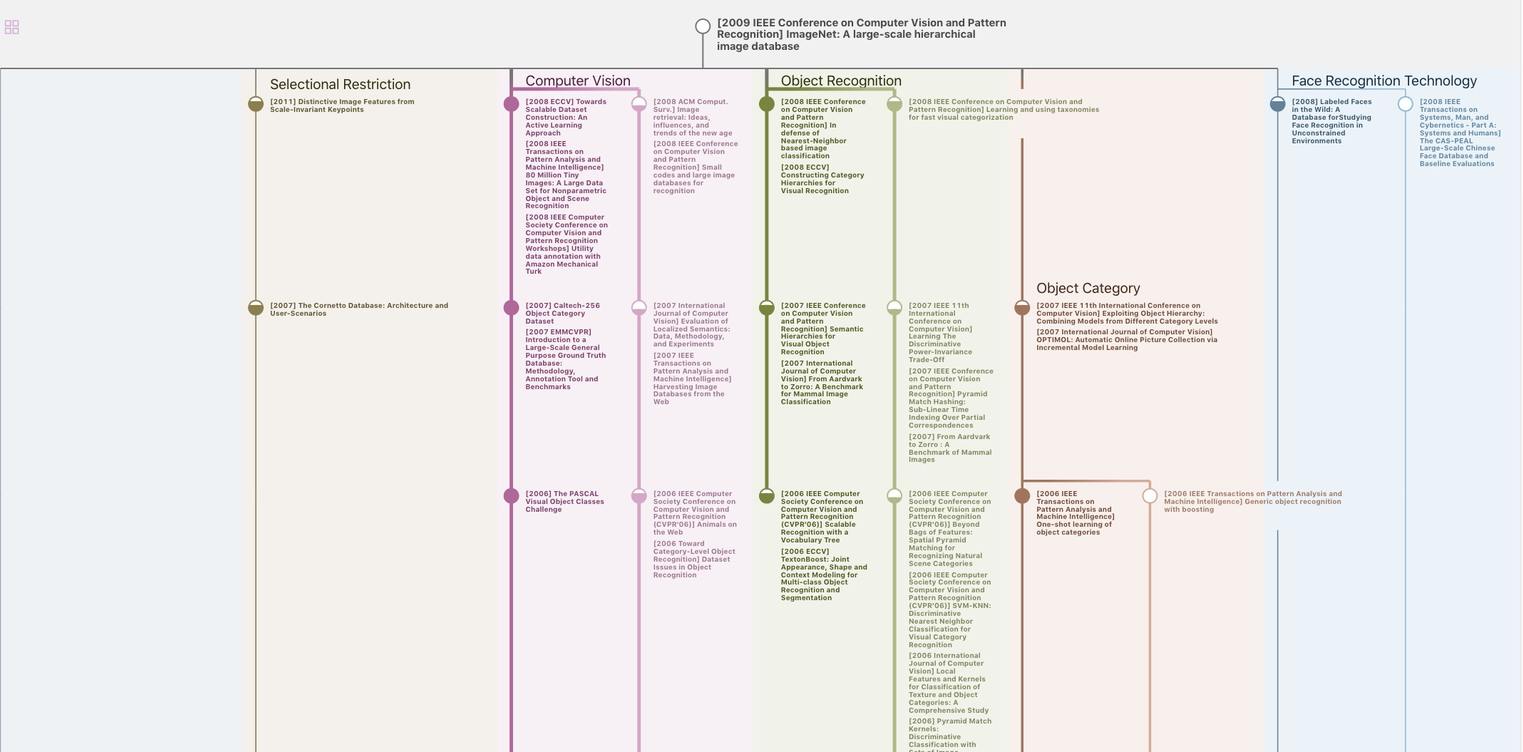
生成溯源树,研究论文发展脉络
Chat Paper
正在生成论文摘要