Iterative 3D Deformable Registration from Single-view RGB Images using Differentiable Rendering
PROCEEDINGS OF THE 17TH INTERNATIONAL JOINT CONFERENCE ON COMPUTER VISION, IMAGING AND COMPUTER GRAPHICS THEORY AND APPLICATIONS (VISAPP), VOL 5(2022)
摘要
For autonomous robotic systems, comprehensive 3D scene parsing is a prerequisite. Machine learning techniques used for 3D scene parsing that incorporate knowledge about the process of 2D image generation from 3D scenes have a big potential. This has sparked an interest in differentiable renderers that provide approximate gradients of the rendered image with respect to scene and object parameters. An efficient differentiable renderer facilitates approaching many 3D scene parsing problems using a render-and-compare framework, where the object and scene parameters are optimized by minimizing the difference between rendered and observed images. In this work, we introduce StilllebenDR, a light-weight scalable differentiable renderer built as an extension to the Stillleben library and use it for 3D deformable registration from single-view RGB images. Our end-to-end differentiable pipeline achieves results comparable to state-of-the-art methods without any training and outperforms the competing methods significantly in the presence of pose initialization errors.
更多查看译文
关键词
Differentiable Rendering, Deformable Registration, Latent Shape-space Model
AI 理解论文
溯源树
样例
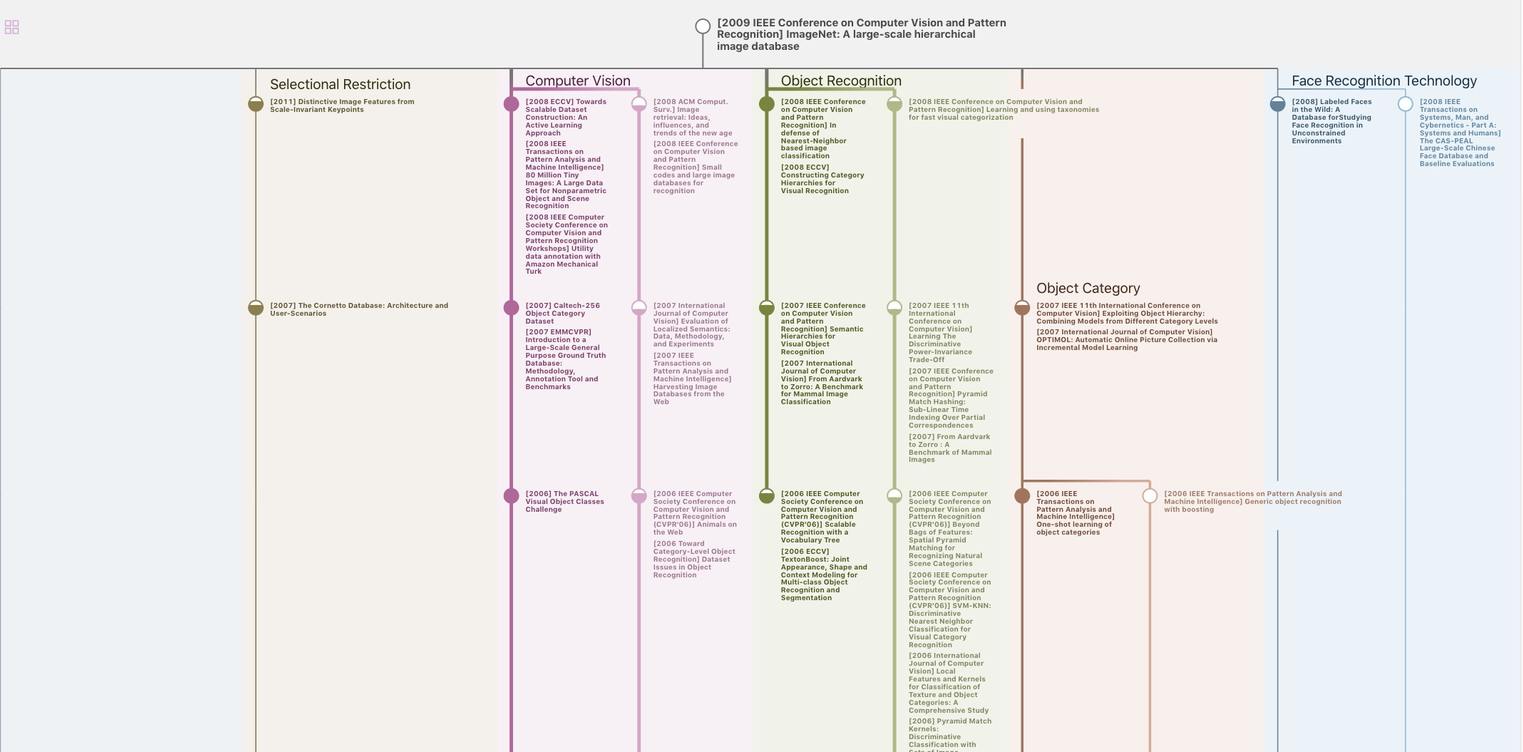
生成溯源树,研究论文发展脉络
Chat Paper
正在生成论文摘要