Enhancing LSTM for sequential image classification by modifying data aggregation
2021 International Conference on Electrical, Computer and Energy Technologies (ICECET)(2021)
摘要
Recurrent Neural Networks (RNN) model sequential information and are commonly used for the analysis of time series. The most usual operation to fuse information in RNNs is the sum. In this work, we use a RNN extended type, Long Short-Term Memory (LSTM) and we use it for image classification, to which we give a sequential interpretation. Since the data used may not be independent to each other, we modify the sum operator of an LSTM unit using the n-dimensional Choquet integral, which considers possible data coalitions. We compare our methods to those based on usual aggregation functions, using the datasets Fashion-MNIST and MNIST.
更多查看译文
关键词
Long Short-Term Memory,Recurrent Neural Network,Sequential Image Classification,Aggregation Functions,Choquet Integral
AI 理解论文
溯源树
样例
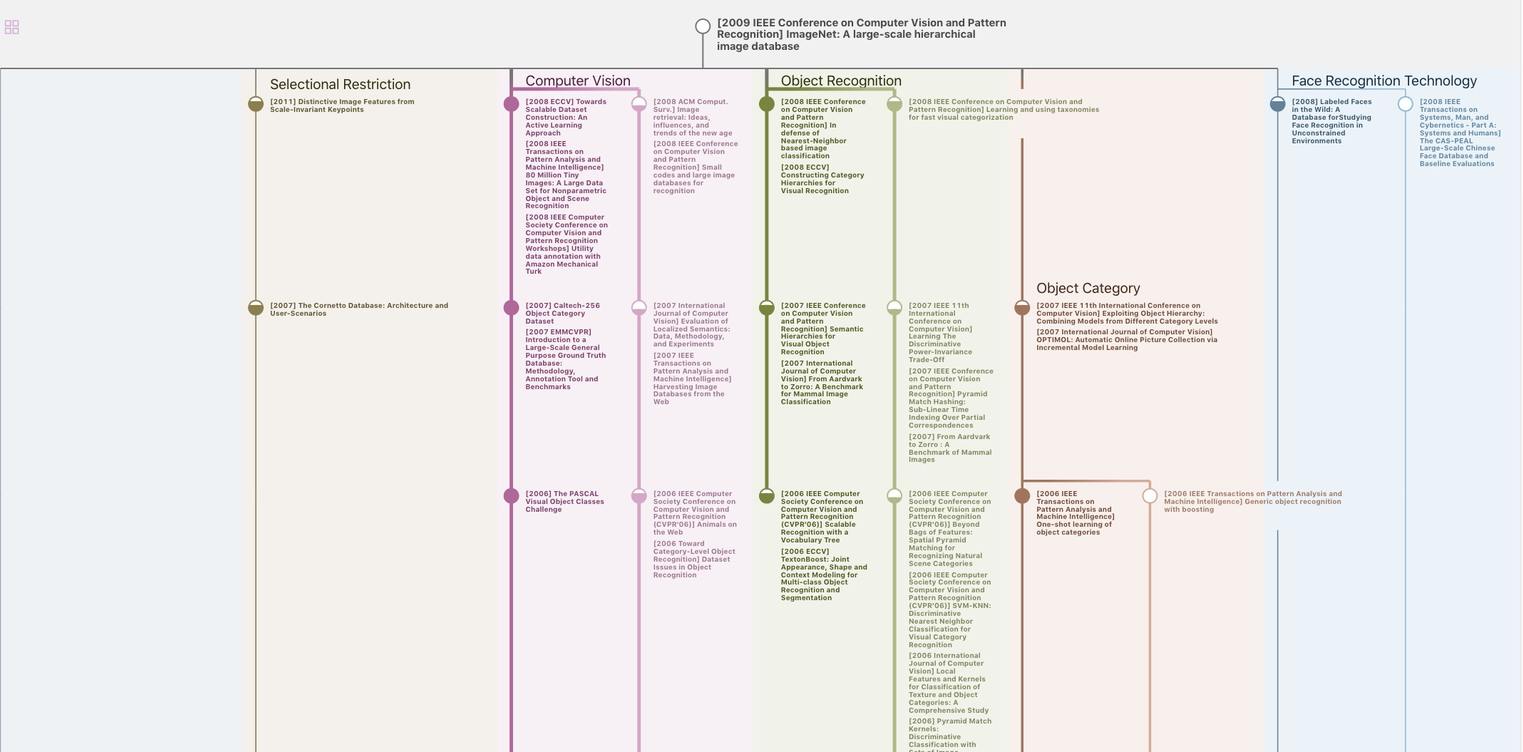
生成溯源树,研究论文发展脉络
Chat Paper
正在生成论文摘要