Compact Neural Architecture Designs by Tensor Representations.
Frontiers in Artificial Intelligence(2022)
摘要
We propose a framework of tensorial neural networks (TNNs) extending existing linear layers on low-order tensors to multilinear operations on higher-order tensors. TNNs have three advantages over existing networks: First, TNNs naturally apply to higher-order data without flattening, which preserves their multi-dimensional structures. Second, compressing a pre-trained network into a TNN results in a model with similar expressive power but fewer parameters. Finally, TNNs interpret advanced compact designs of network architectures, such as bottleneck modules and interleaved group convolutions. To learn TNNs, we derive their backpropagation rules using a novel suite of generalized tensor algebra. With backpropagation, we can either learn TNNs from scratch or pre-trained models using knowledge distillation. Experiments on VGG, ResNet, and Wide-ResNet demonstrate that TNNs outperform the state-of-the-art low-rank methods on a wide range of backbone networks and datasets.
更多查看译文
关键词
deep learning,model compression,neural networks,tensor decomposition,tensor networks
AI 理解论文
溯源树
样例
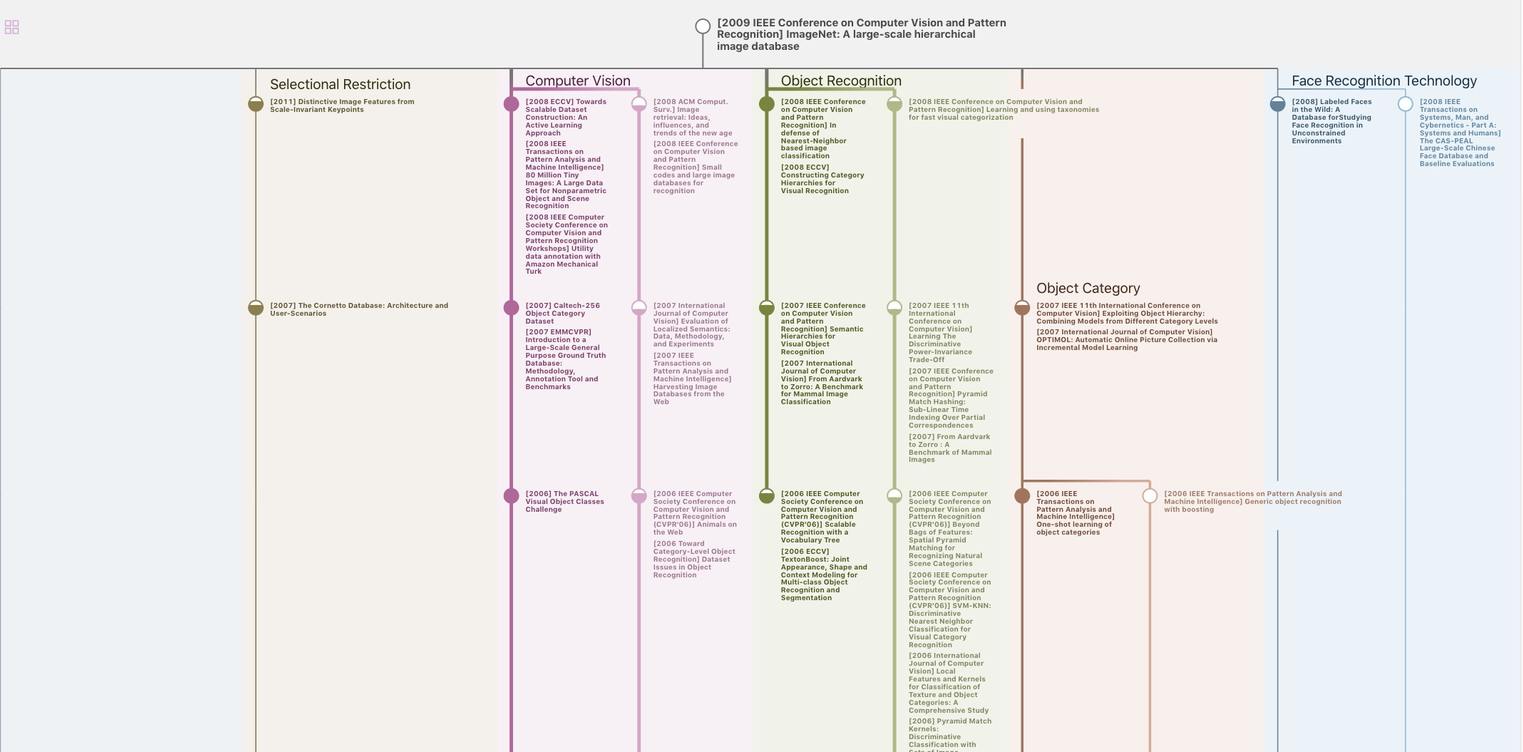
生成溯源树,研究论文发展脉络
Chat Paper
正在生成论文摘要