An Efficient Sampling-Based Attention Network for Semantic Segmentation
IEEE TRANSACTIONS ON IMAGE PROCESSING(2022)
摘要
Self-attention is widely explored to model long-range dependencies in semantic segmentation. However, this operation computes pair-wise relationships between the query point and all other points, leading to prohibitive complexity. In this paper, we propose an efficient Sampling-based Attention Network which combines a novel sample method with an attention mechanism for semantic segmentation. Specifically, we design a Stochastic Sampling-based Attention Module (SSAM) to capture the relationships between the query point and a stochastic sampled representative subset from a global perspective, where the sampled subset is selected by a Stochastic Sampling Module. Compared to self-attention, our SSAM achieves comparable segmentation performance while significantly reducing computational redundancy. In addition, with the observation that not all pixels are interested in the contextual information, we design a Deterministic Sampling-based Attention Module (DSAM) to sample features from a local region for obtaining the detailed information. Extensive experiments demonstrate that our proposed method can compete or perform favorably against the state-of-the-art methods on the Cityscapes, ADE20K, COCO Stuff, and PASCAL Context datasets.
更多查看译文
关键词
Stochastic processes, Sampling methods, Semantics, Image segmentation, Computational complexity, Pattern recognition, Convolution, Semantic segmentation, stochastic sampling-based attention, deterministic sampling-based attention
AI 理解论文
溯源树
样例
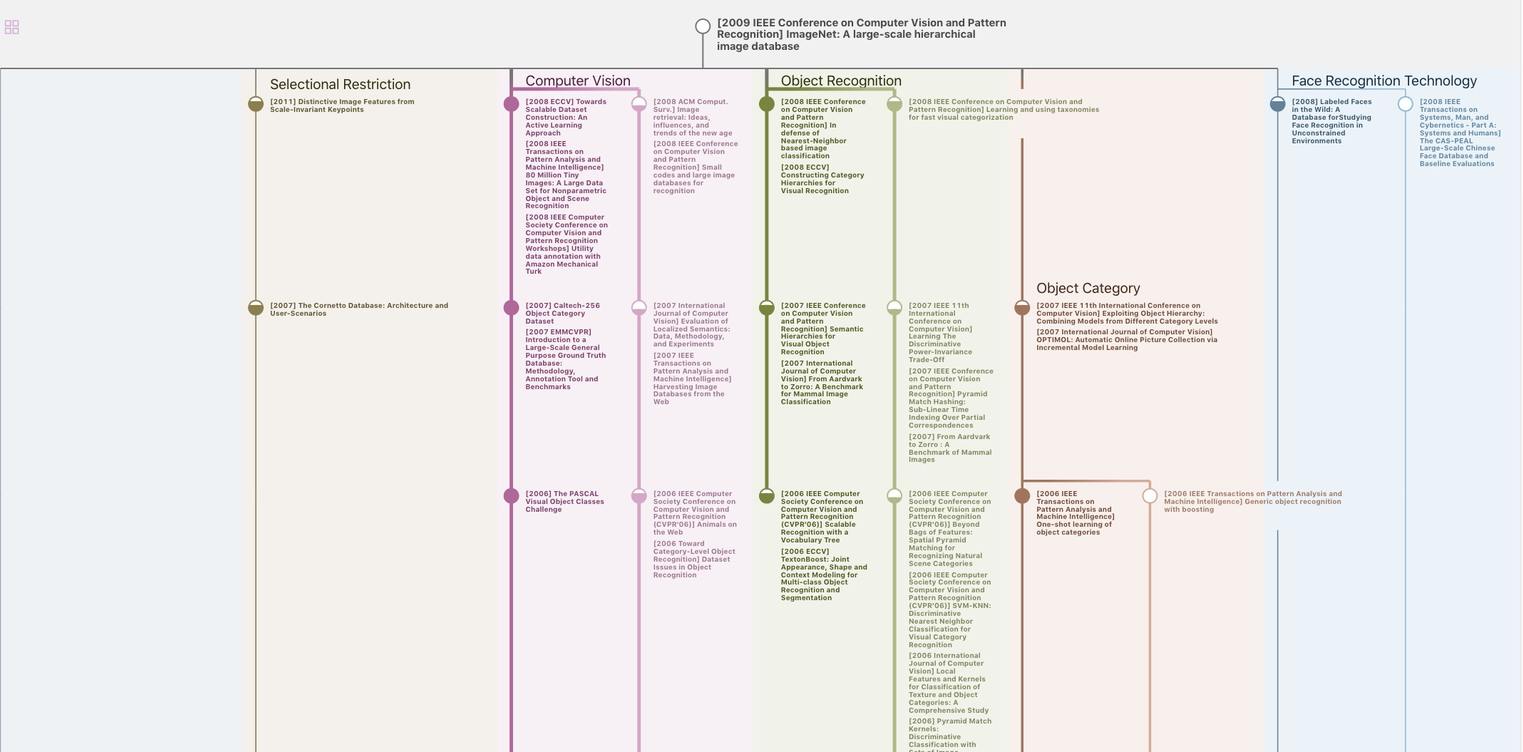
生成溯源树,研究论文发展脉络
Chat Paper
正在生成论文摘要