CrabNet: Fully Task-Specific Feature Learning for One-Stage Object Detection
IEEE TRANSACTIONS ON IMAGE PROCESSING(2022)
摘要
Object detection is usually solved by learning a deep architecture involving classification and localization tasks, where feature learning for these two tasks is shared using the same backbone model. Recent works have shown that suitable disentanglement of classification and localization tasks has the great potential to improve performance of object detection. Despite the promising performance, existing feature disentanglement methods usually suffer from two limitations. First, most of them only focus on the disentangled proposals or predication heads for classification and localization tasks after RPN. While little consideration has been given to that the features for these two different tasks actually are obtained by a shared backbone model before RPN. Second, they are suggested for two-stage objectors and are not applicable to one-stage methods. To overcome these limitations, this paper presents a novel fully task-specific feature learning method for one-stage object detection. Specifically, our method first learns disentangled features for classification and localization tasks using two separated backbone models, where auxiliary classification and localization heads are inserted at the end of the two backbone models for providing a fully task-specific features for classification and localization. Then, a feature interaction module is developed for aligning and fusing task-specific features, which are further used to produce the final detection result. Experiments on MS COCO show that our proposed method (dubbed CrabNet) can achieve clear improvement over counterparts with increasing limited inference time, while performing favorably against state-of-the-arts.
更多查看译文
关键词
Location awareness, Task analysis, Feature extraction, Object detection, Representation learning, Detectors, Proposals, Object detection, convolutional network, feature disentanglement, feature interaction
AI 理解论文
溯源树
样例
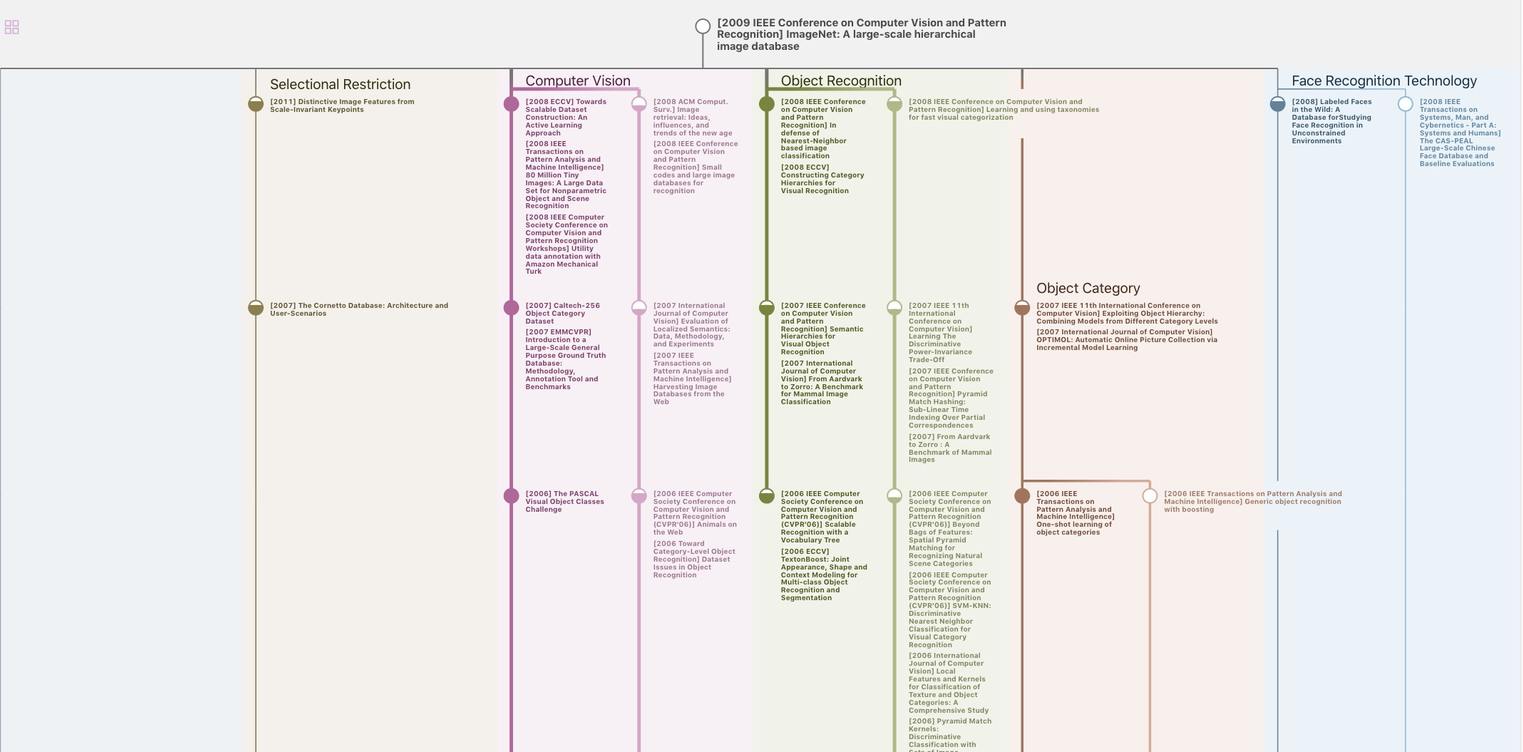
生成溯源树,研究论文发展脉络
Chat Paper
正在生成论文摘要