Video-Text Representation Learning via Differentiable Weak Temporal Alignment
IEEE Conference on Computer Vision and Pattern Recognition(2022)
摘要
Learning generic joint representations for video and text by a supervised method requires a prohibitively substantial amount of manually annotated video datasets. As a practical alternative, a large-scale but uncurated and narrated video dataset, HowTo100M, has recently been introduced. But it is still challenging to learn joint embeddings of video and text in a self-supervised manner, due to its ambiguity and non-sequential alignment. In this paper, we propose a novel multi-modal self-supervised framework Video-Text Temporally Weak Alignment-based Contrastive Learning (VT-TWINS) to capture significant information from noisy and weakly correlated data using a variant of Dynamic Time Warping (DTW). We observe that the standard DTW inherently cannot handle weakly correlated data and only considers the globally optimal alignment path. To address these problems, we develop a differentiable DTW which also reflects local information with weak temporal alignment. Moreover, our proposed model applies a contrastive learning scheme to learn feature representations on weakly correlated data. Our extensive experiments demonstrate that VT-TWINS attains significant improvements in multi-modal representation learning and outperforms various challenging downstream tasks. Code is available at https://github.com/mlvlab/VT-Twins.
更多查看译文
关键词
Vision + language, Representation learning, Self-& semi-& meta- Video analysis and understanding
AI 理解论文
溯源树
样例
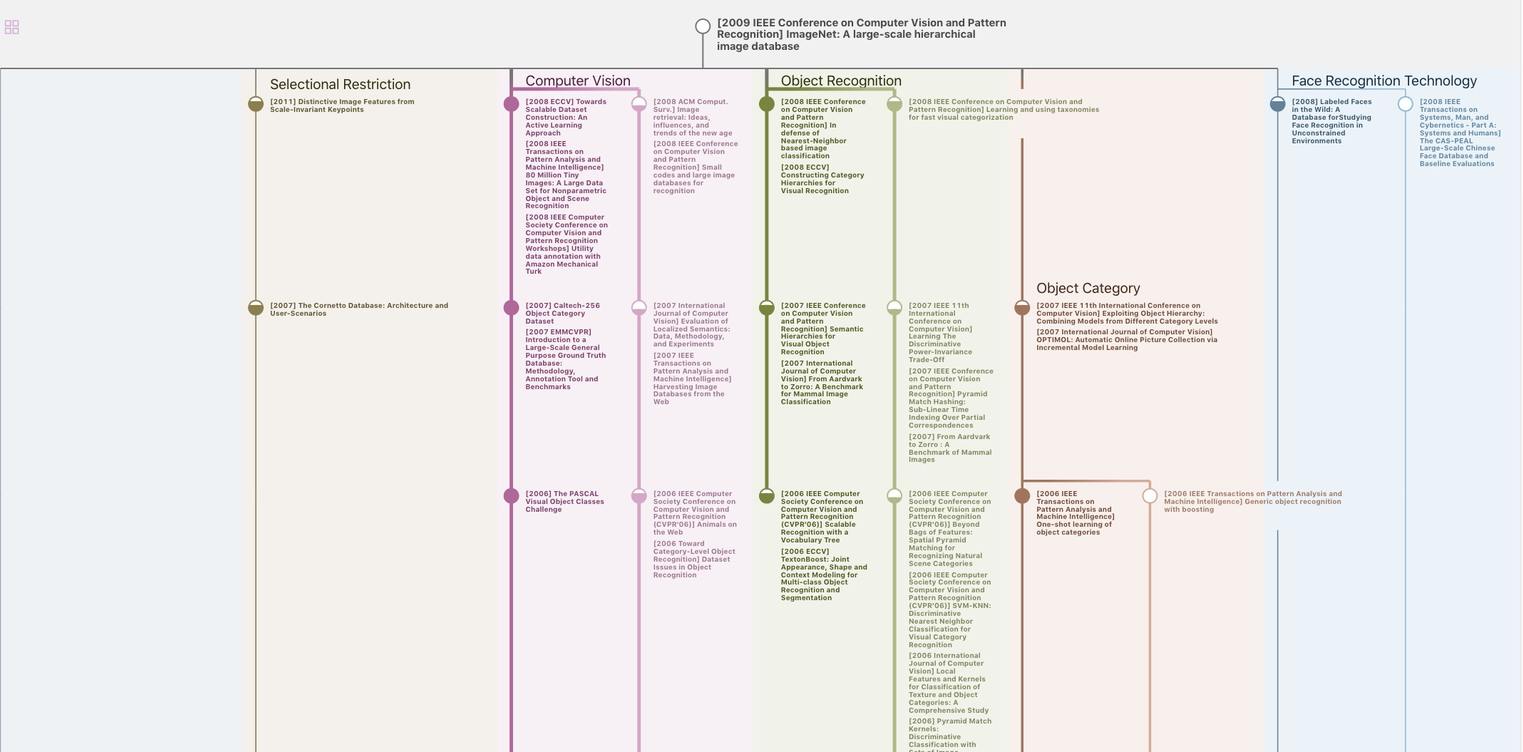
生成溯源树,研究论文发展脉络
Chat Paper
正在生成论文摘要