Spatio-Temporal Graph Convolutional Neural Networks for Physics-Aware Grid Learning Algorithms
arxiv(2023)
摘要
This paper proposes a model-free Volt-VAR control (VVC) algorithm via the spatio-temporal graph ConvNet-based deep reinforcement learning (STGCN-DRL) framework, whose goal is to control smart inverters in an unbalanced distribution system. We first identify the graph shift operator (GSO) based on the power flow equations. Then, we develop a spatio-temporal graph ConvNet (STGCN), testing both recurrent graph ConvNets (RGCN) and convolutional graph ConvNets (CGCN) architectures, aimed at capturing the spatiotemporal correlation of voltage phasors. The STGCN layer performs the feature extraction task for the policy function and the value function of the reinforcement learning architecture, and then we utilize the proximal policy optimization (PPO) to search the action spaces for an optimum policy function and to approximate an optimum value function. We further utilize the low-pass property of voltage graph signal to introduce an GCN architecture for the the policy whose input is a decimated state vector, i.e. a partial observation. Case studies on the unbalanced 123-bus systems validate the excellent performance of the proposed method in mitigating instabilities and maintaining nodal voltage profiles within a desirable range.
更多查看译文
关键词
grid,networks,learning,spatio-temporal,physics-aware
AI 理解论文
溯源树
样例
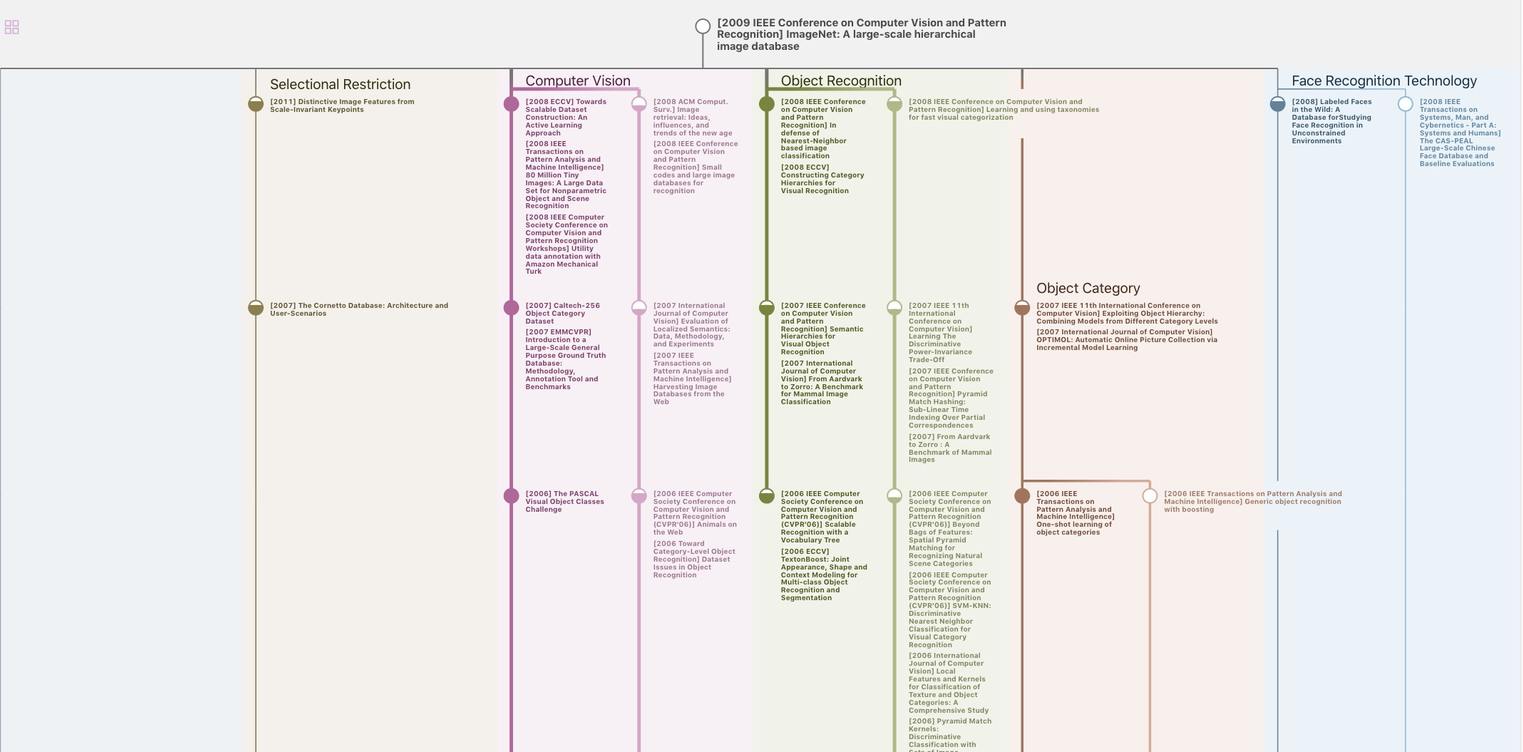
生成溯源树,研究论文发展脉络
Chat Paper
正在生成论文摘要