Hybrid Handcrafted and Learnable Audio Representation for Analysis of Speech Under Cognitive and Physical Load
Conference of the International Speech Communication Association (INTERSPEECH)(2022)
摘要
As a neurophysiological response to threat or adverse conditions, stress can affect cognition, emotion and behaviour with potentially detrimental effects on health in the case of sustained exposure. Since the affective content of speech is inherently modulated by an individual's physical and mental state, a substantial body of research has been devoted to the study of paralinguistic correlates of stress-inducing task load. Historically, voice stress analysis (VSA) has been conducted using conventional digital signal processing (DSP) techniques. Despite the development of modern methods based on deep neural networks (DNNs), accurately detecting stress in speech remains difficult due to the wide variety of stressors and considerable variability in the individual stress perception. To that end, we introduce a set of five datasets for task load detection in speech. The voice recordings were collected as either cognitive or physical stress was induced in the cohort of volunteers, with a cumulative number of more than a hundred speakers. We used the datasets to design and evaluate a novel self-supervised audio representation that leverages the effectiveness of handcrafted features (DSP-based) and the complexity of data-driven DNN representations. Notably, the proposed approach outperformed both extensive handcrafted feature sets and novel DNN-based audio representation learning approaches.
更多查看译文
关键词
computational paralinguistics, voice stress analysis, audio representation learning, DSP features, deep learning
AI 理解论文
溯源树
样例
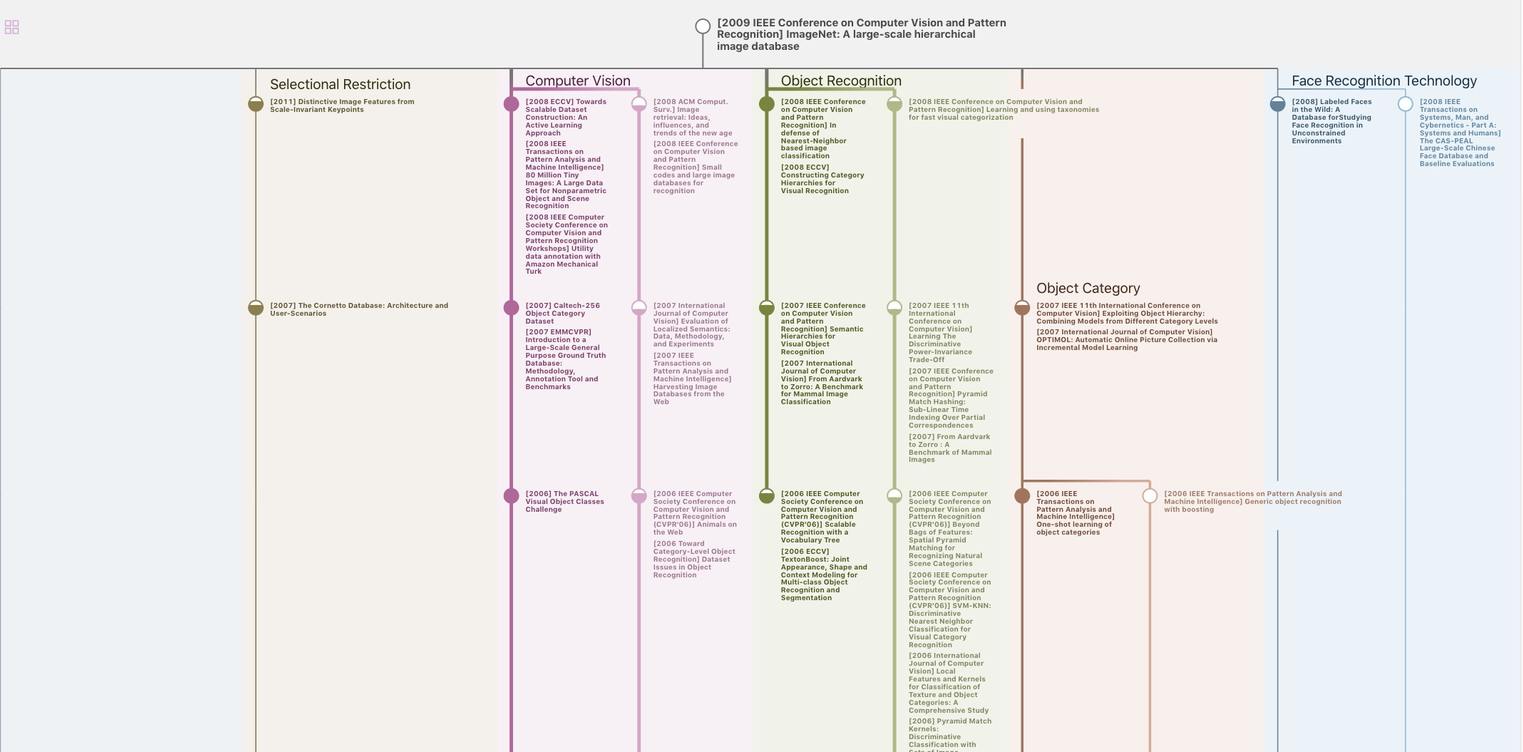
生成溯源树,研究论文发展脉络
Chat Paper
正在生成论文摘要