Ordered community detection in directed networks
PHYSICAL REVIEW E(2022)
摘要
We develop a method to infer community structure in directed networks where the groups are ordered in a latent one-dimensional hierarchy that determines the preferred edge direction. Our nonparametric Bayesian approach is based on a modification of the stochastic block model (SBM), which can take advantage of rank alignment and coherence to produce parsimonious descriptions of networks that combine ordered hierarchies with arbitrary mixing patterns between groups. Since our model also includes directed degree correction, we can use it to distinguish nonlocal hierarchical structure from local in-and out-degree imbalance-thus, removing a source of conflation present in most ranking methods. We also demonstrate how we can reliably compare with the results obtained with the unordered SBM variant to determine whether a hierarchical ordering is statistically warranted in the first place. We illustrate the application of our method on a wide variety of empirical networks across several domains.
更多查看译文
关键词
community detection,networks
AI 理解论文
溯源树
样例
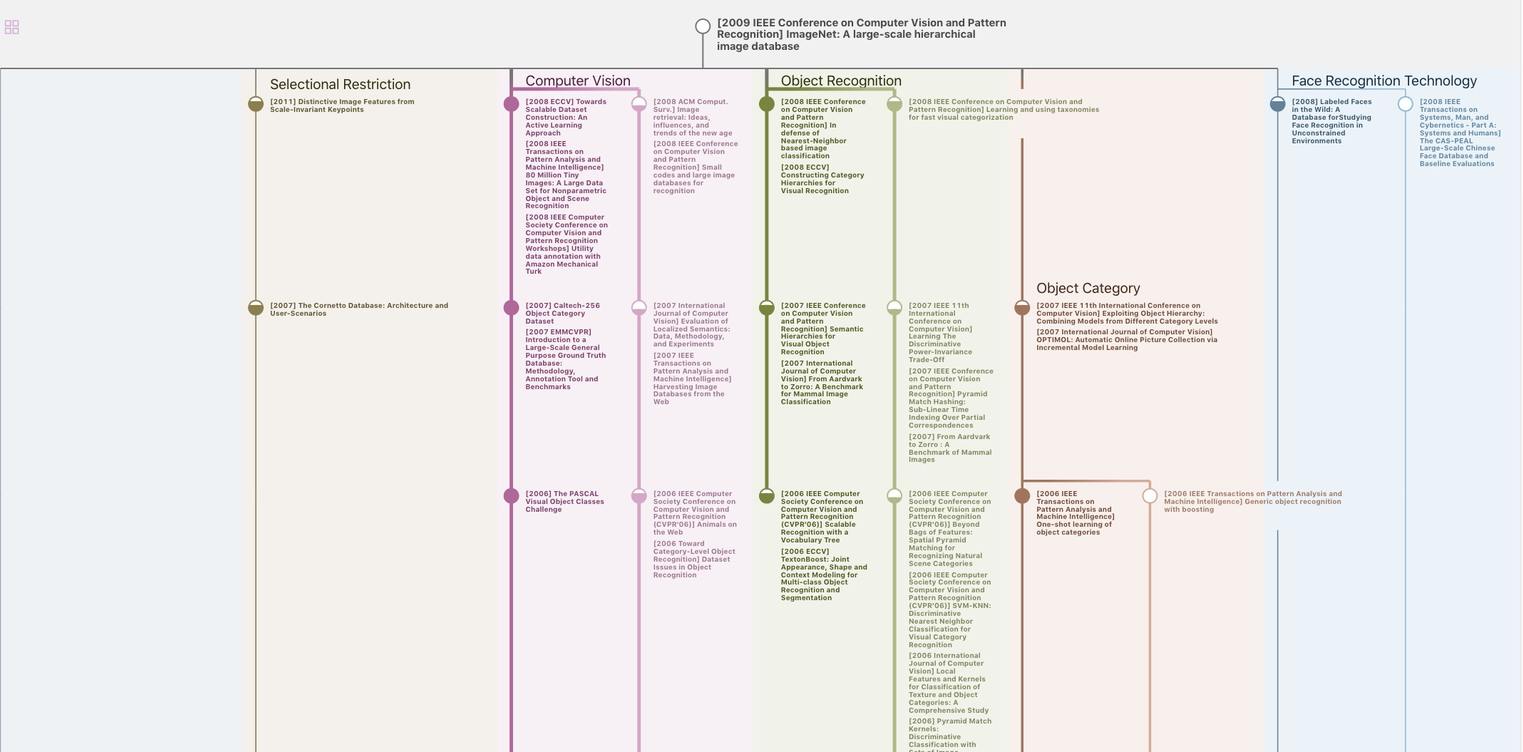
生成溯源树,研究论文发展脉络
Chat Paper
正在生成论文摘要