Self-Supervised Learning for Recommender Systems: A Survey
IEEE TRANSACTIONS ON KNOWLEDGE AND DATA ENGINEERING(2024)
摘要
In recent years, neural architecture-based recommender systems have achieved tremendous success, but they still fall short of expectation when dealing with highly sparse data. Self-supervised learning (SSL), as an emerging technique for learning from unlabeled data, has attracted considerable attention as a potential solution to this issue. This survey paper presents a systematic and timely review of research efforts on self-supervised recommendation (SSR). Specifically, we propose an exclusive definition of SSR, on top of which we develop a comprehensive taxonomy to divide existing SSR methods into four categories: contrastive, generative, predictive, and hybrid. For each category, we elucidate its concept and formulation, the involved methods, as well as its pros and cons. Furthermore, to facilitate empirical comparison, we release an open-source library SELFRec (https://github.com/Coder-Yu/SELFRec), which incorporates a wide range of SSR models and benchmark datasets. Through rigorous experiments using this library, we derive and report some significant findings regarding the selection of self-supervised signals for enhancing recommendation. Finally, we shed light on the limitations in the current research and outline the future research directions.
更多查看译文
关键词
Recommendation,self-supervised learning,contrastive learning,pre-training,data augmentation
AI 理解论文
溯源树
样例
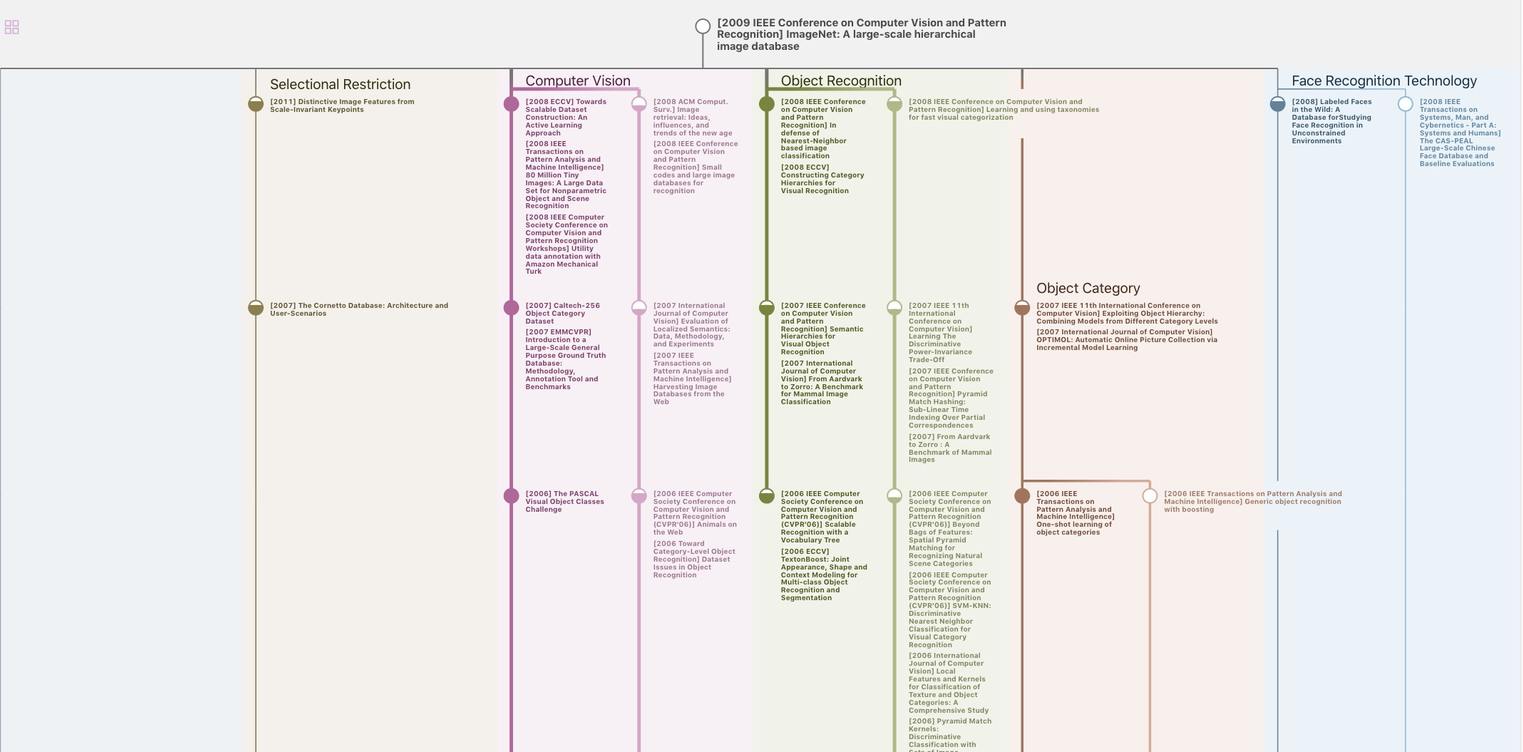
生成溯源树,研究论文发展脉络
Chat Paper
正在生成论文摘要