Envedit: Environment Editing for Vision-and-Language Navigation
IEEE Conference on Computer Vision and Pattern Recognition(2022)
摘要
In Vision-and-Language Navigation (VLN), an agent needs to navigate through the environment based on nat-ural language instructions. Due to limited available data for agent training and finite diversity in navigation environments, it is challenging for the agent to generalize to new, unseen environments. To address this problem, we propose Envedit, a data augmentation method that cre-ates new environments by editing existing environments, which are used to train a more generalizable agent. Our augmented environments can differ from the seen environ-ments in three diverse aspects: style, object appearance, and object classes. Training on these edit-augmented environments prevents the agent from overfitting to existing en-vironments and helps generalize better to new, unseen en-vironments. Empirically, on both the Room-to-Room and the multi-lingual Room-Across-Room datasets, we show that our proposed Envedit method gets significant im-provements in all metrics on both pre-trained and non-pre-trained VLN agents, and achieves the new state-of-the-art on the test leaderboard. We further ensemble the VLN agents augmented on different edited environments and show that these edit methods are complementary. 1 1 Code and data are available at https://github.com/jialuli-luka/EnvEdit.
更多查看译文
关键词
Vision + language
AI 理解论文
溯源树
样例
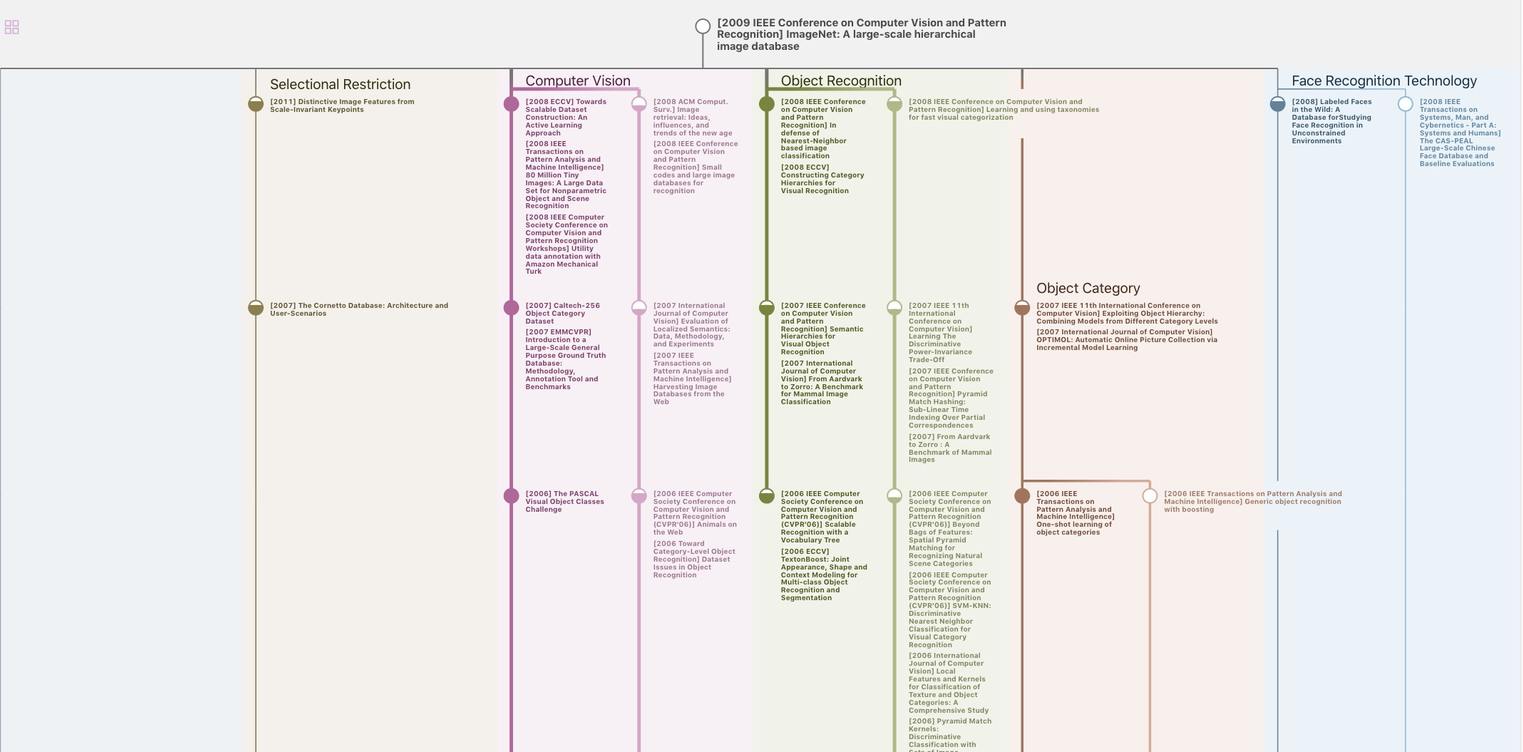
生成溯源树,研究论文发展脉络
Chat Paper
正在生成论文摘要