Stochastic Conservative Contextual Linear Bandits
arxiv(2022)
摘要
Many physical systems have underlying safety considerations that require that the strategy deployed ensures the satisfaction of a set of constraints. Further, often we have only partial information on the state of the system. We study the problem of safe real-time decision making under uncertainty. In this paper, we formulate a conservative stochastic contextual bandit formulation for real-time decision making when an adversary chooses a distribution on the set of possible contexts and the learner is subject to certain safety/performance constraints. The learner observes only the context distribution and the exact context is unknown, and the goal is to develop an algorithm that selects a sequence of optimal actions to maximize the cumulative reward without violating the safety constraints at any time step. By leveraging the UCB algorithm for this setting, we propose a conservative linear UCB algorithm for stochastic bandits with context distribution. We prove an upper bound on the regret of the algorithm and show that it can be decomposed into three terms: (i) an upper bound for the regret of the standard linear UCB algorithm, (ii) a constant term (independent of time horizon) that accounts for the loss of being conservative in order to satisfy the safety constraint, and (ii) a constant term (independent of time horizon) that accounts for the loss for the contexts being unknown and only the distribution being known. To validate the performance of our approach we perform extensive simulations on synthetic data and on real-world maize data collected through the Genomes to Fields (G2F) initiative.
更多查看译文
关键词
conservative linear UCB algorithm,conservative stochastic contextual bandit formulation,constant term,context distribution,cumulative reward,exact context,forecasting mechanism,noisy measurement,optimal actions,possible contexts,real-time decision making,safety constraint,standard linear UCB algorithm,stochastic bandits,stochastic conservative contextual linear,time horizon,upper confidence bound algorithm
AI 理解论文
溯源树
样例
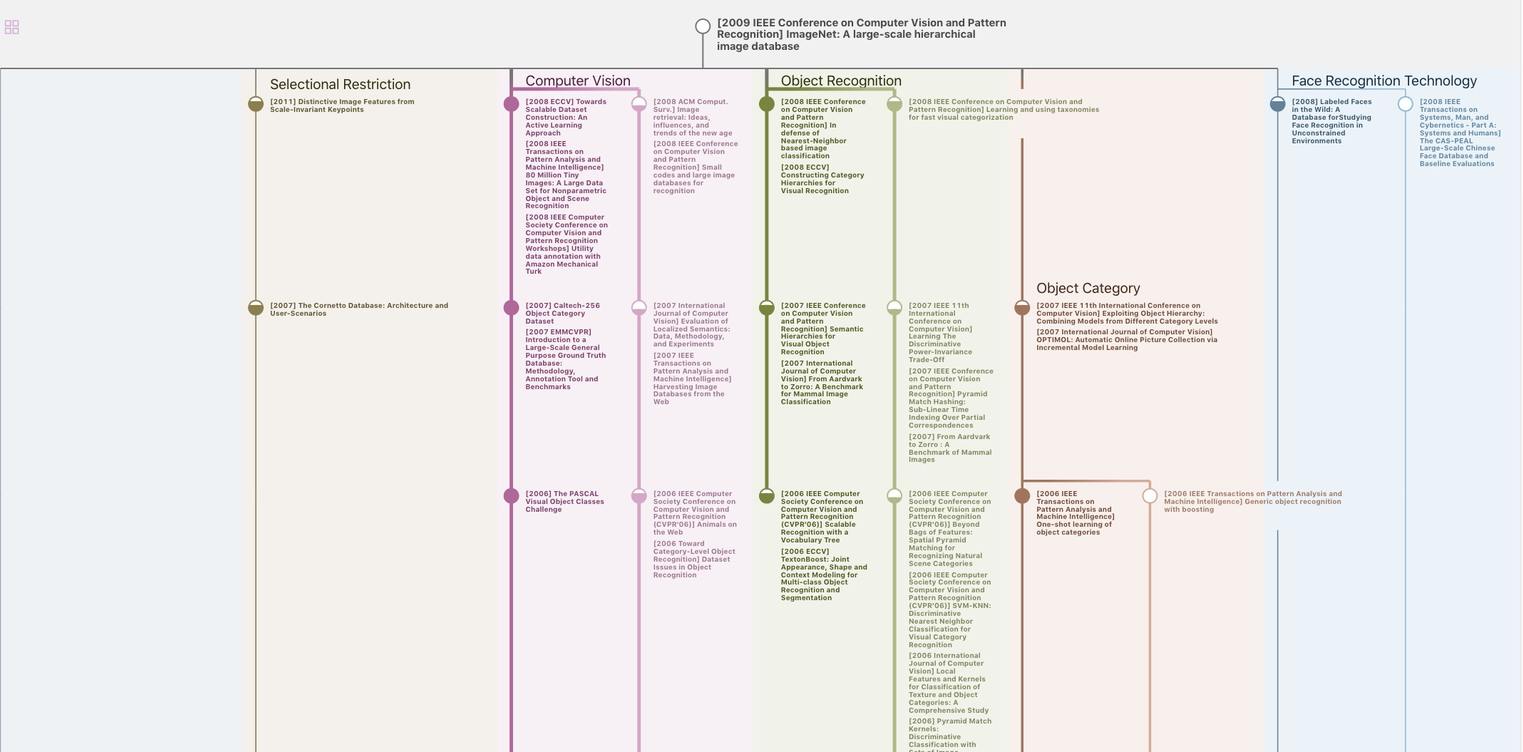
生成溯源树,研究论文发展脉络
Chat Paper
正在生成论文摘要