Deep learning reconstruction for 1.5 T cervical spine MRI: effect on interobserver agreement in the evaluation of degenerative changes
European Radiology(2022)
摘要
Objectives To investigate whether deep learning reconstruction (DLR) provides improved cervical spine MR images using a 1.5 T unit in the evaluation of degenerative changes without increasing imaging time. Methods This study included 21 volunteers (age 42.4 ± 11.9 years; 17 males) who underwent 1.5 T cervical spine sagittal T2-weighted MRI. From the imaging data with number of acquisitions (NAQ) of 1 or 2, images were reconstructed with DLR (NAQ1-DLR) and without DLR (NAQ1) or without DLR (NAQ2), respectively. Two readers evaluated the images for depiction of structures, artifacts, noise, overall image quality, spinal canal stenosis, and neuroforaminal stenosis. The two readers read studies blinded and randomly. Values were compared between NAQ1-DLR and NAQ1 and between NAQ1-DLR and NAQ2 using the Wilcoxon signed-rank test. Results The analyses showed significantly better results for NAQ1-DLR compared with NAQ1 and NAQ2 ( p < 0.023), except for depiction of disc and foramina by one reader and artifacts by both readers in the comparison between NAQ1-DLR and NAQ2. Interobserver agreements (Cohen’s weighted kappa [97.5% confidence interval]) in the evaluation of spinal canal stenosis for NAQ1-DLR/NAQ1/NAQ2 were 0.874 (0.866–0.883)/0.778 (0.767–0.789)/0.818 (0.809–0.827), respectively, and those in the evaluation of neuroforaminal stenosis were 0.878 (0.872–0.883)/0.855 (0.849–0.860)/0.852 (0.845–0.860), respectively. Conclusions DLR improved the 1.5 T cervical spine MR images in the evaluation of degenerative spine changes. Key Points • Two radiologists demonstrated that deep learning reconstruction reduced the noise in cervical spine sagittal T2-weighted MR images obtained using a 1.5 T unit . • Reduced noise in deep learning reconstruction images resulted in a clearer depiction of structures, such as the spinal cord, vertebrae, and zygapophyseal joint . • Interobserver agreement in the evaluation of spinal canal stenosis and foraminal stenosis on cervical spine MR images was significantly improved using deep learning reconstruction (0.874 and 0.878, respectively) versus without deep learning (0.778–0.818 and 0.852–0.855, respectively) .
更多查看译文
关键词
Artificial intelligence, Deep learning, Magnetic resonance imaging, Cervical vertebrae, Neurodegenerative diseases
AI 理解论文
溯源树
样例
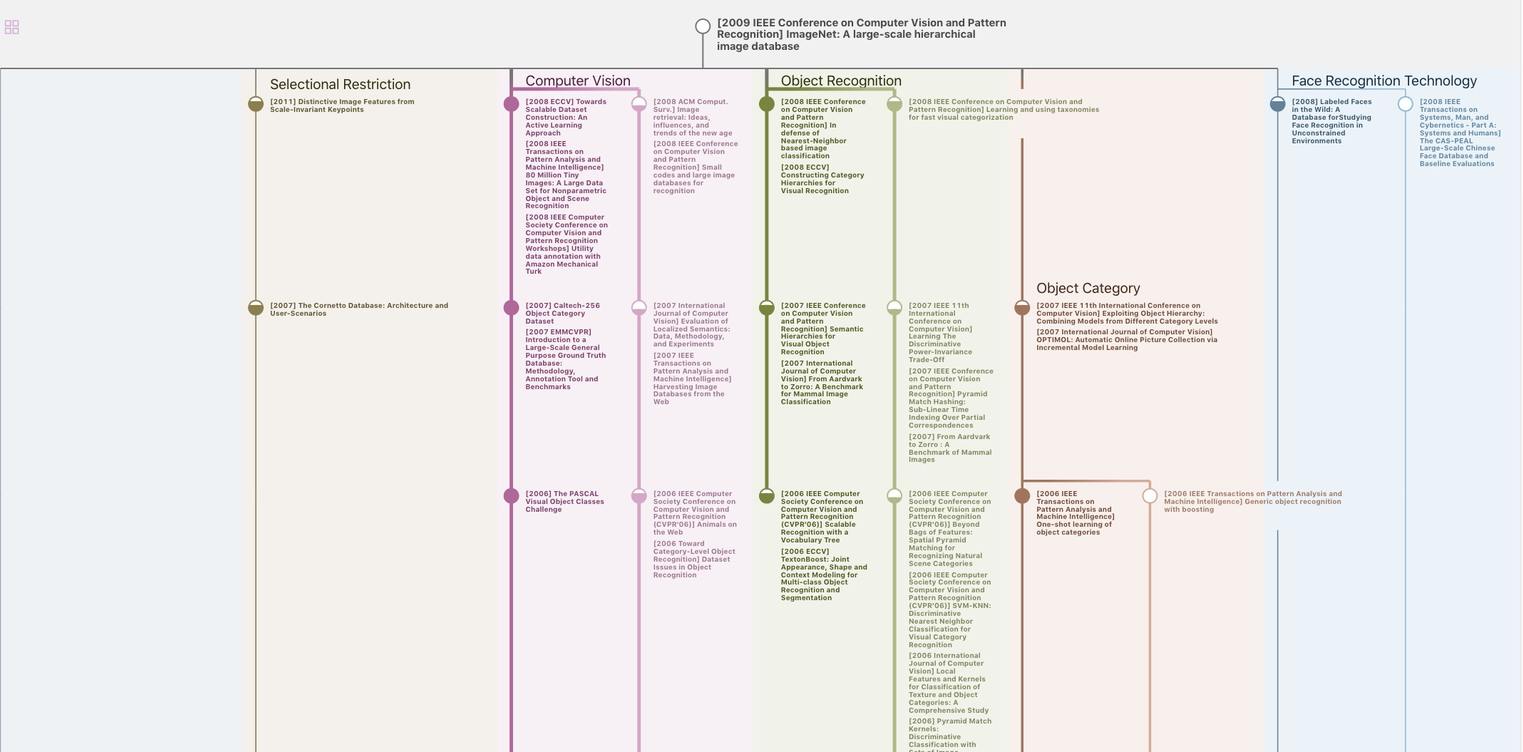
生成溯源树,研究论文发展脉络
Chat Paper
正在生成论文摘要