Domain Invariant Siamese Attention Mask for Small Object Change Detection via Everyday Indoor Robot Navigation
2022 IEEE/RSJ International Conference on Intelligent Robots and Systems (IROS)(2022)
摘要
The problem of image change detection via every-day indoor robot navigation is explored from a novel perspective of the self-attention technique. Detecting semantically non-distinctive and visually small changes remains a key challenge in the robotics community. Intuitively, these small non-distinctive changes may be better handled by the recent paradigm of the attention mechanism, which is the basic idea of this work. However, existing self-attention models require significant retraining cost per domain, so it is not directly applicable to robotics applications. We propose a new self-attention technique with an ability of unsupervised on-the-fly domain adaptation, which introduces an attention mask into the intermediate layer of an image change detection model, without modifying the input and output layers of the model. Experiments, in which an indoor robot aims to detect visually small changes in everyday navigation, demonstrate that our attention technique significantly boosts the state-of-the-art image change detection model. Our datset is available at https://github.com/KojiTakeda00/Small_object_change_detection
更多查看译文
关键词
small object change detection,attention,robot
AI 理解论文
溯源树
样例
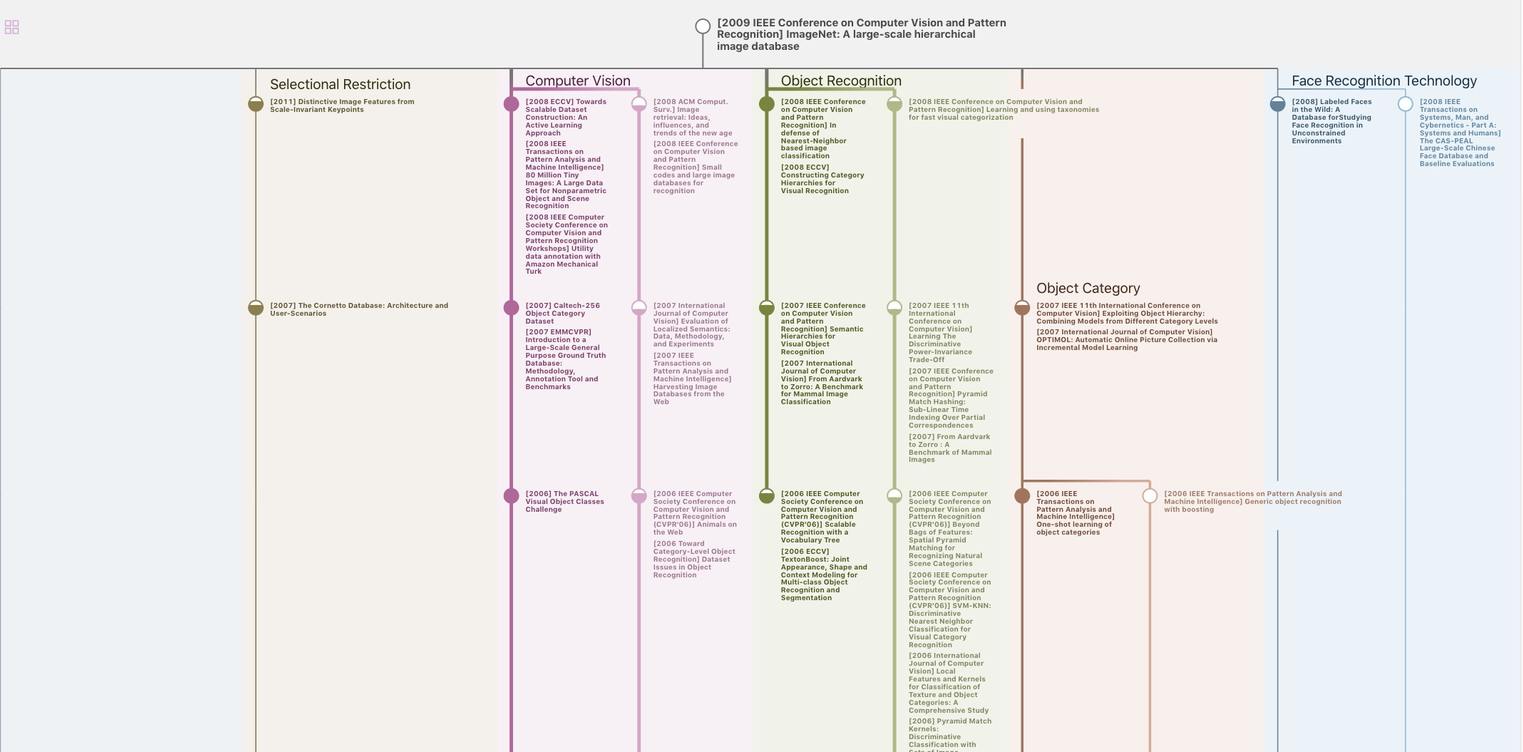
生成溯源树,研究论文发展脉络
Chat Paper
正在生成论文摘要