Cardiac CT motion artifact grading via semi-automatic labeling and vessel tracking using synthetic image-augmented training data
JOURNAL OF X-RAY SCIENCE AND TECHNOLOGY(2022)
摘要
Cardiac CT provides critical information for the evaluation of cardiovascular diseases. However, involuntary patient motion and physiological movement of the organs during CT scanning cause motion blur in the reconstructed CT images, degrading both cardiac CT image quality and its diagnostic value. In this paper, we propose and demonstrate an effective and efficient method for CT coronary angiography image quality grading via semi-automatic labeling and vessel tracking. These algorithms produce scores that accord with those of expert readers to within 0.85 points on a 5-point scale. We also train a neural network model to perform fully-automatic motion artifact grading. We demonstrate, using XCAT simulation tools to generate realistic phantom CT data, that supplementing clinical data with synthetic data improves the scoring performance of this network. With respect to ground truth scores assigned by expert operators, the mean square error of grading motion of the right coronary artery is reduced by 36% by synthetic data supplementation. This demonstrates that augmentation of clinical training data with realistically synthesized images can potentially reduce the number of clinical studies needed to train the network.
更多查看译文
关键词
Cardiac CT, motion artifacts, semi-automatic labeling, vessel tracking, deep learning
AI 理解论文
溯源树
样例
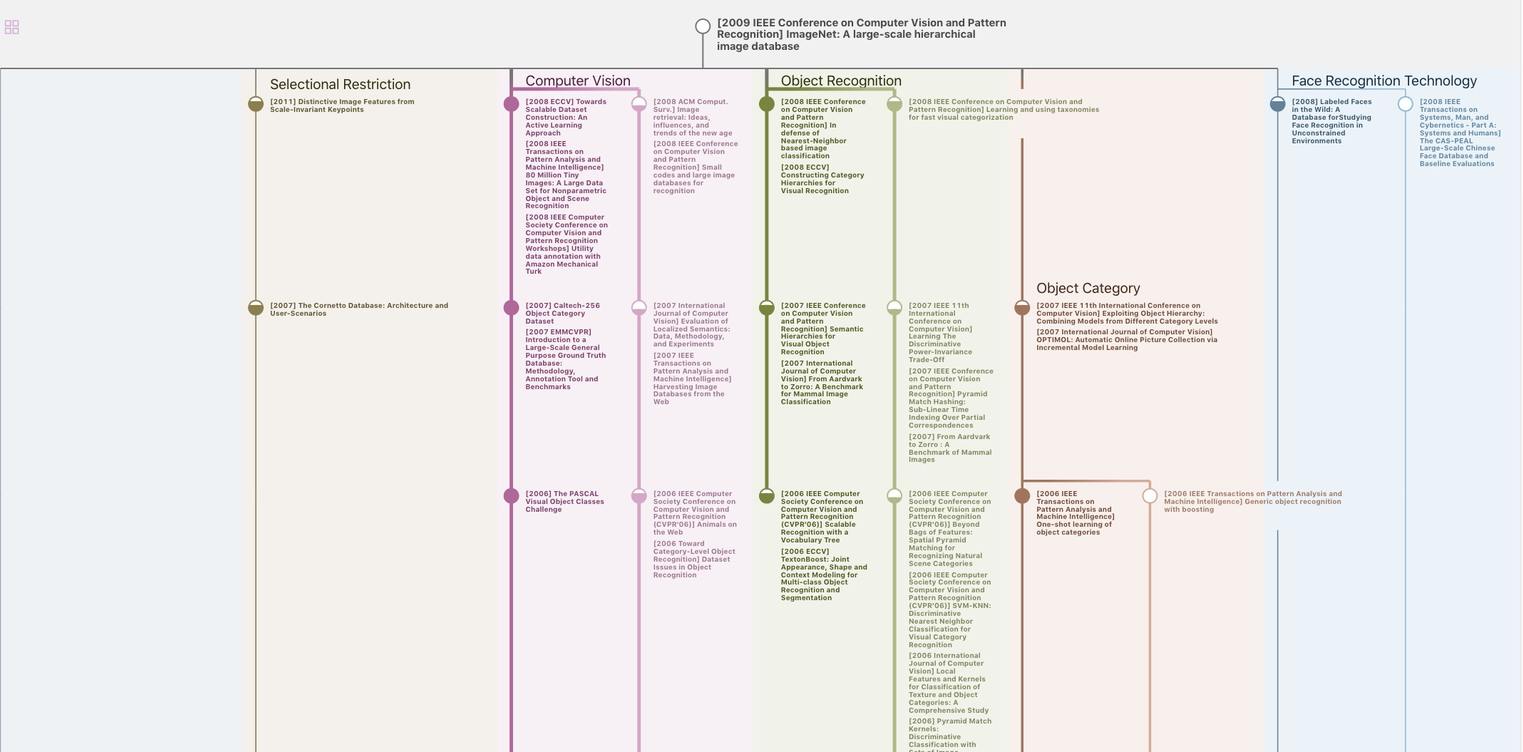
生成溯源树,研究论文发展脉络
Chat Paper
正在生成论文摘要