Multi-attribute scientific documents retrieval and ranking model based on GBDT and LR.
MATHEMATICAL BIOSCIENCES AND ENGINEERING(2022)
摘要
Scientific documents contain a large number of mathematical expressions and texts containing mathematical semantics. Simply using mathematical expressions or text to retrieve scientific documents can hardly meet retrieval needs. The real difficulty in retrieving scientific documents is to effectively integrate mathematical expressions and related textual features. Therefore, this study proposes a multi-attribute scientific documents retrieval and ranking model based on GBDT (gradient boosting decision tree) and LR (logistic regression) by integrating the expressions and text contained in scientific documents. First, the similarities of the five attributes are calculated, including mathematical expression symbols, mathematical expression sub-forms, mathematical expression context, scientific document keywords and the frequency of mathematical expressions. Next, the GBDT model is used to discretize and reorganize the five attributes. Finally, the reorganized features are input into the LR model, and the final retrieval and ranking results of scientific documents are obtained. The experiment in this study was carried out on the NTCIR dataset. The average value of the final MAP@20 of the scientific document recall was 81.92%. The average value of the scientific document ranking nDCG@20 was 86.05%.
更多查看译文
关键词
scientific document retrieval and ranking, mathematical expression, multi-attribute feature, hesitation fuzzy sets, GBDT, LR
AI 理解论文
溯源树
样例
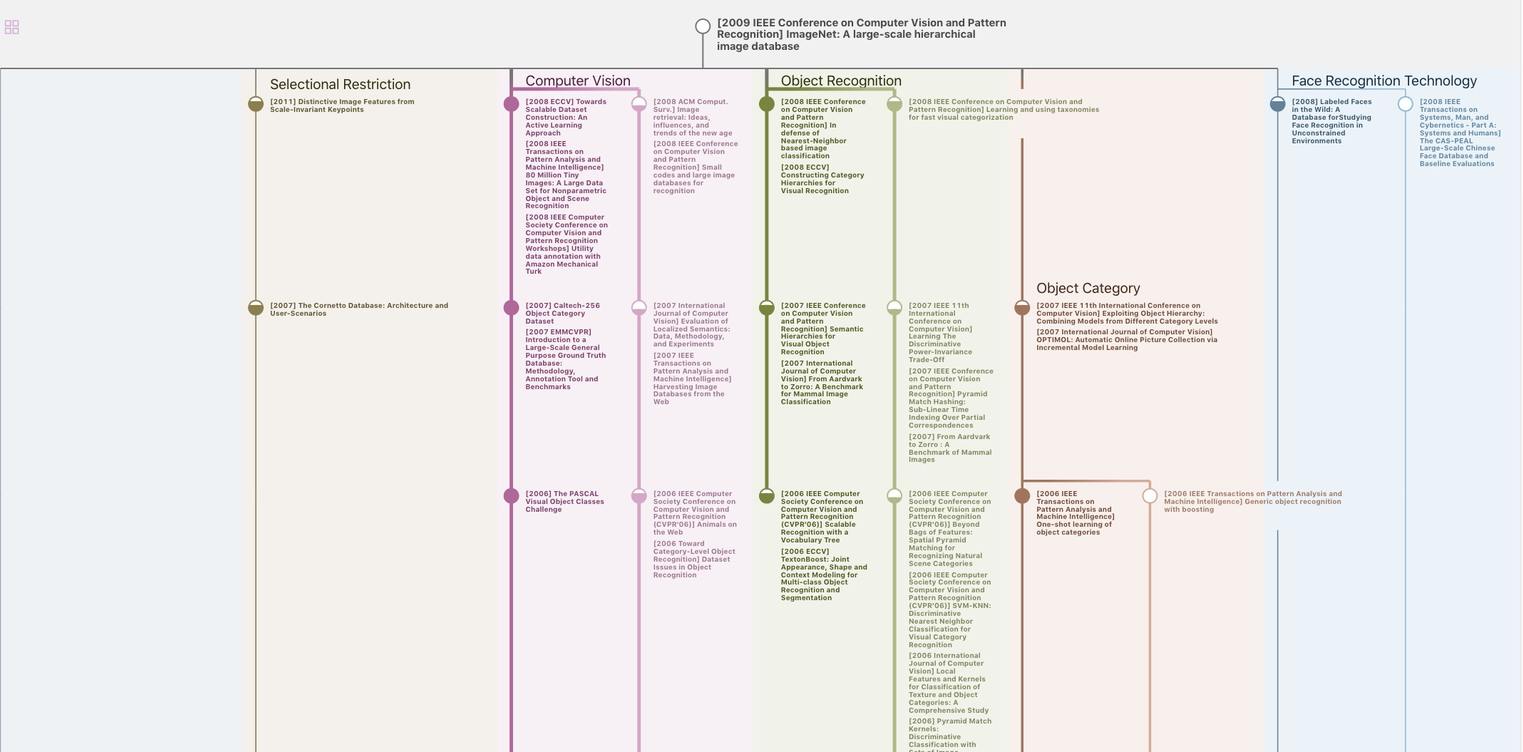
生成溯源树,研究论文发展脉络
Chat Paper
正在生成论文摘要