Personalized Preoperative Prediction of the Length of Hospital Stay after TAVI Using a Dedicated Decision Tree Algorithm
JOURNAL OF PERSONALIZED MEDICINE(2022)
摘要
Background: The aim of this study was to identify pre-operative parameters able to predict length of stay (LoS) based on clinical data and patient-reported outcome measures (PROMs) from a scorecard database in patients with significant aortic stenosis who underwent TAVI (transfemoral aortic valve implantation). Methods: 302 participants (51.7% males, age range 78.2-84.2 years.) were prospectively recruited. After computing the median LoS value (=6 days, range = 5-8 days), we implemented a decision tree algorithm by setting dichotomized values at median LoS as the dependent variable and assessed baseline clinical variables and PROMs (Clinical Frailty Scale (CFS), EuroQol-5 Dimension-5 Levels (EQ-5D) and Kansas City Cardiomyopathy Questionnaire (KCCQ)) as potential predictors. Results: Among clinical parameters, only peripheral arterial disease (p = 0.029, HR = 1.826) and glomerular filtration rate (GFR, cut-off < 33 mL/min/1.73 m(2), p = 0.003, HR = 2.252) were predictive of LoS. Additionally, two PROMs (CFS; cut-off = 3, p < 0.001, HR = 1.324 and KCCQ; cut-off = 30, p = 0.003, HR = 2.274) were strong predictors. Further, a risk score for LoS (RS_LoS) was calculated based on these predictors. Patients with RS_LoS = 0 had a median LoS of 5 days; patients RS_LoS >= 3 had a median LoS of 8 days. Conclusions: based on the pre-operative values of the above four predictors, a personalized prediction of LoS after TAVI can be achieved.
更多查看译文
关键词
prediction, patient-reported outcomes, aortic stenosis, TAVI, hospital length of stay, decision tree, algorithm
AI 理解论文
溯源树
样例
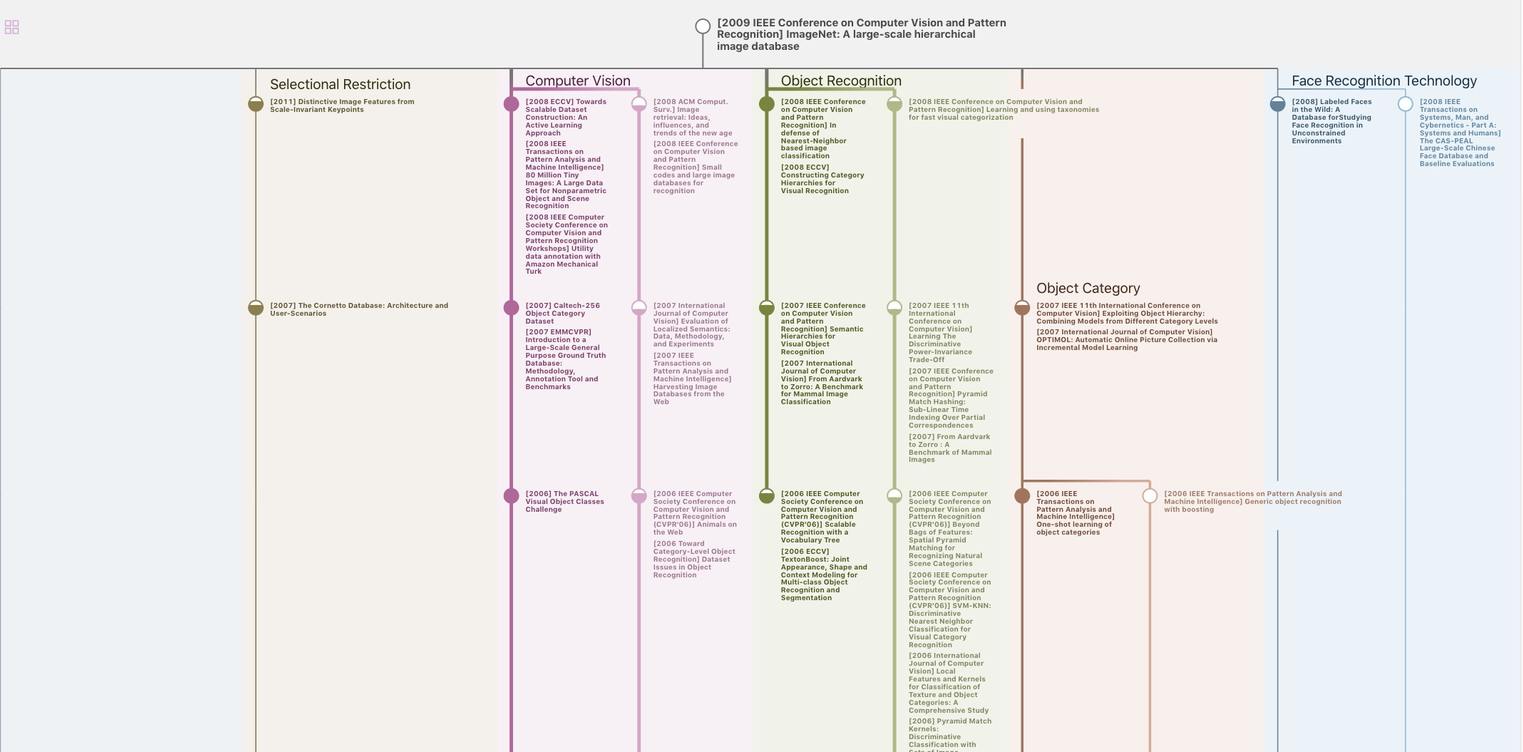
生成溯源树,研究论文发展脉络
Chat Paper
正在生成论文摘要