A Long Short-term Memory Based Recurrent Neural Network for Interventional MRI Reconstruction
arxiv(2022)
摘要
Interventional magnetic resonance imaging (i-MRI) for surgical guidance could help visualize the interventional process such as deep brain stimulation (DBS), improving the surgery performance and patient outcome. Different from retrospective reconstruction in conventional dynamic imaging, i-MRI for DBS has to acquire and reconstruct the interventional images sequentially online. Here we proposed a convolutional long short-term memory (Conv-LSTM) based recurrent neural network (RNN), or ConvLR, to reconstruct interventional images with golden-angle radial sampling. By using an initializer and Conv-LSTM blocks, the priors from the pre-operative reference image and intra-operative frames were exploited for reconstructing the current frame. Data consistency for radial sampling was implemented by a soft-projection method. An adversarial learning strategy was adopted to improve the reconstruction accuracy. A set of interventional images based on the pre-operative and post-operative MR images were simulated for algorithm validation. Results showed with only 10 radial spokes, ConvLR provided the best performance compared with state-of-the-art methods, giving an acceleration up to 40 folds. The proposed algorithm has the potential to achieve real-time i-MRI for DBS and can be used for general purpose MR-guided intervention.
更多查看译文
关键词
mri,neural network,memory,reconstruction,short-term
AI 理解论文
溯源树
样例
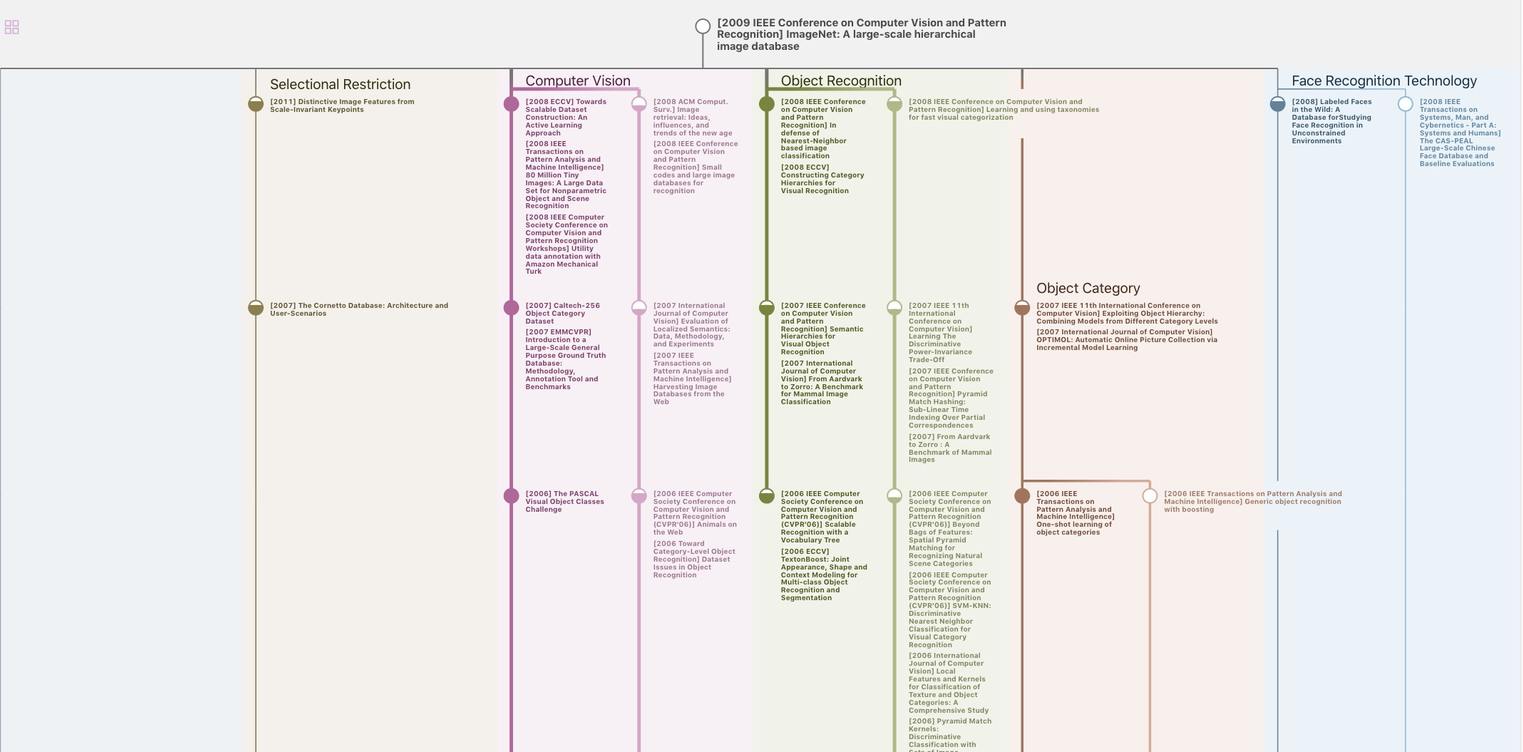
生成溯源树,研究论文发展脉络
Chat Paper
正在生成论文摘要