Knowledge Distillation: Bad Models Can Be Good Role Models
NeurIPS 2022(2022)
摘要
Large neural networks trained in the overparameterized regime are able to fit noise to zero train error. Recent work \citep{nakkiran2020distributional} has empirically observed that such networks behave as "conditional samplers" from the noisy distribution. That is, they replicate the noise in the train data to unseen examples. We give a theoretical framework for studying this conditional sampling behavior in the context of learning theory. We relate the notion of such samplers to knowledge distillation, where a student network imitates the outputs of a teacher on unlabeled data. We show that samplers, while being bad classifiers, can be good teachers. Concretely, we prove that distillation from samplers is guaranteed to produce a student which approximates the Bayes optimal classifier. Finally, we show that some common learning algorithms (e.g., Nearest-Neighbours and Kernel Machines) can generate samplers when applied in the overparameterized regime.
更多查看译文
关键词
Knowledge Distillation,Teacher-Student,Learning Theory,Learning to Sample,Ensembling
AI 理解论文
溯源树
样例
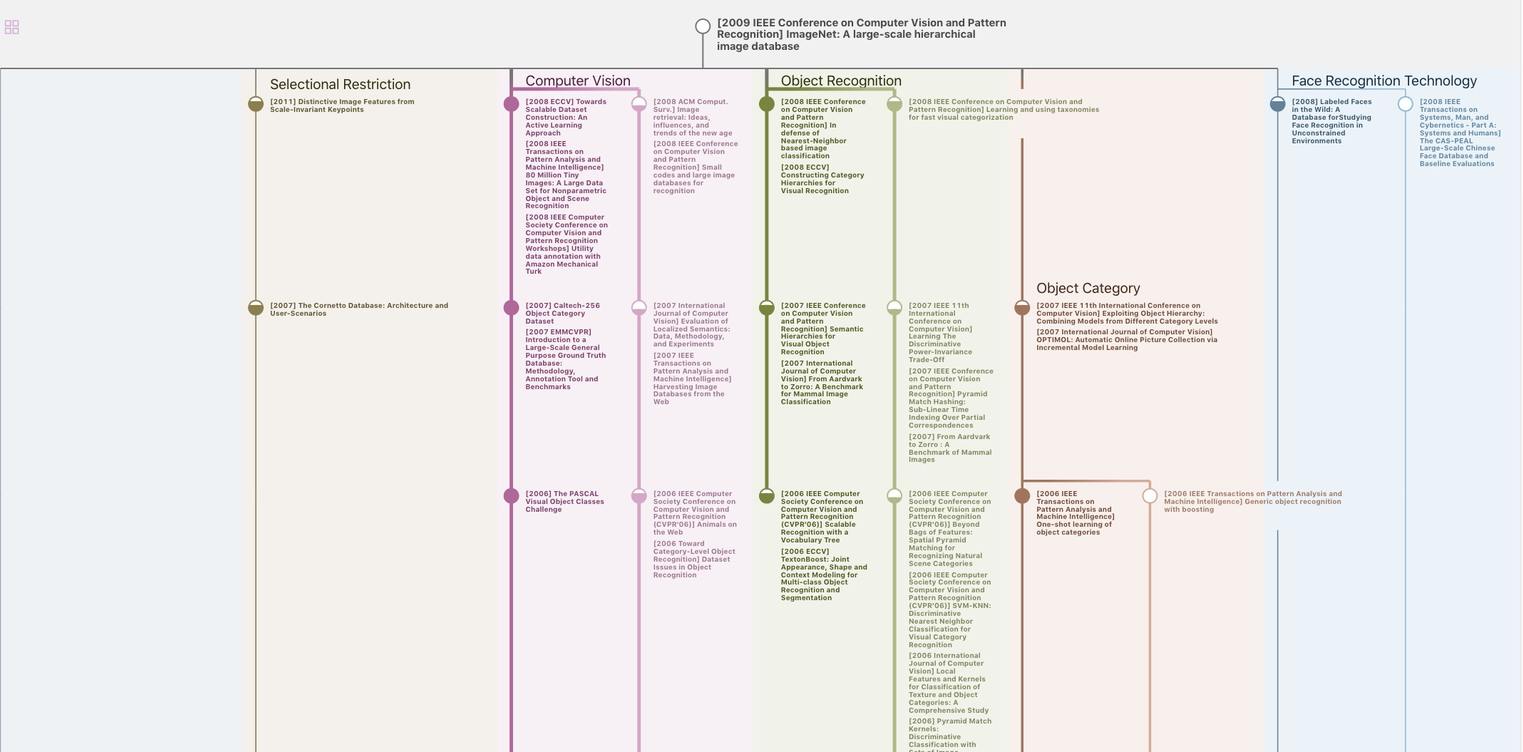
生成溯源树,研究论文发展脉络
Chat Paper
正在生成论文摘要