Unit-norm tight frame-based sparse representation with application to speech inpainting
DIGITAL SIGNAL PROCESSING(2022)
摘要
This paper deals with the unit-norm tight frames (UNTFs) that better represent and solve sparse representation problems. Under this sparse representation framework, a novel model termed tight dictionary learning (TDL) is proposed. Unlike those dictionaries that only focus on the signals of interest, TDL intends to learn a more comprehensive dictionary that better represents the signals with the UNTF properties to facilitate sparse recovery. To better describe the physical meanings, the normalization constraints on the dictionary columns are imposed. A gradient-based approach is developed to obtain the tight dictionary where the normalization constraints are embedded into the cost function by a parametrization method. The learned tight dictionary is applied to the speech inpainting problem to restore speech signals corrupted by data missing. In order to compensate the degradation effect of the data missing, a UNTF-based preconditioning technique is developed to reform the frame properties of the degraded system. Parametrization and gradient-based approaches similar to those applied in the TDL are adopted to design a tight preconditioner. Extensive experiments on speech denoising and speech segment restoration are conducted to demonstrate the superiority of the proposed schemes. (C) 2022 Elsevier Inc. All rights reserved.
更多查看译文
关键词
Sparse representation, Dictionary learning, Unit-norm tight frame, Speech inpainting, Preconditioning
AI 理解论文
溯源树
样例
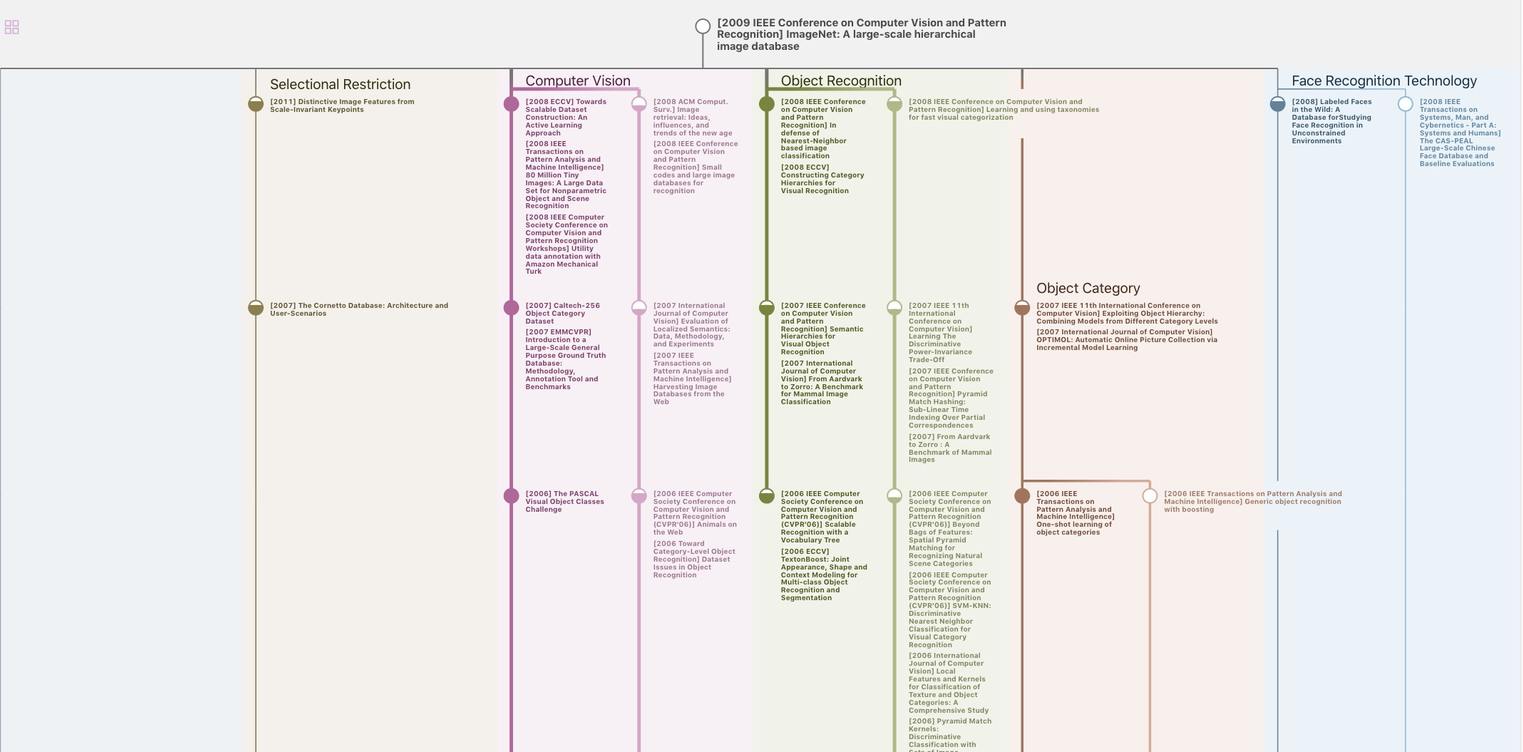
生成溯源树,研究论文发展脉络
Chat Paper
正在生成论文摘要