Generalization bounds for learning under graph-dependence: a survey
Machine Learning(2024)
摘要
Traditional statistical learning theory relies on the assumption that data are identically and independently distributed (i.i.d.). However, this assumption often does not hold in many real-life applications. In this survey, we explore learning scenarios where examples are dependent and their dependence relationship is described by a dependency graph, a commonly utilized model in probability and combinatorics. We collect various graph-dependent concentration bounds, which are then used to derive Rademacher complexity and stability generalization bounds for learning from graph-dependent data. We illustrate this paradigm through practical learning tasks and provide some research directions for future work. To our knowledge, this survey is the first of this kind on this subject.
更多查看译文
关键词
Generalization bounds,Dependency graphs,Uniform stability,Rademacher complexity,Bipartite ranking
AI 理解论文
溯源树
样例
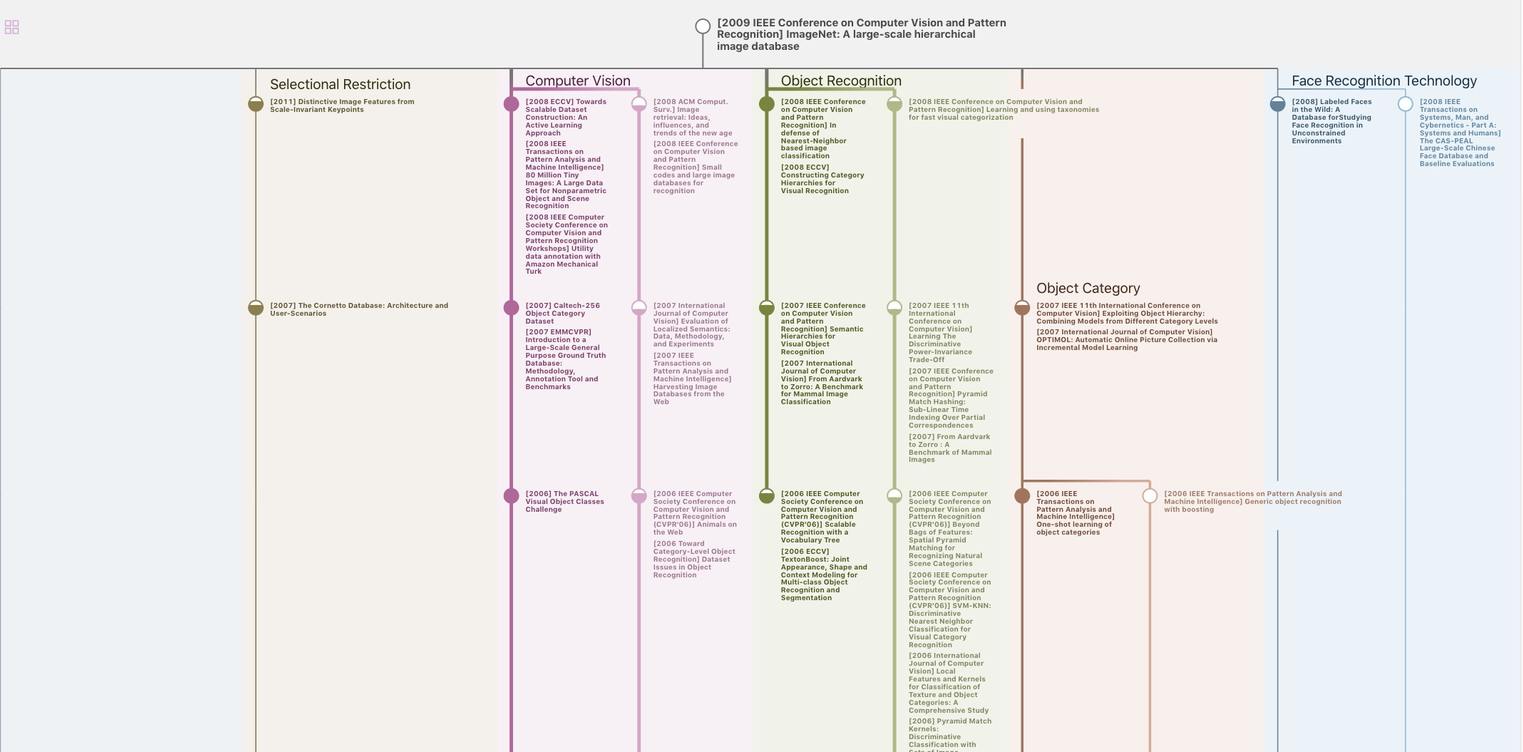
生成溯源树,研究论文发展脉络
Chat Paper
正在生成论文摘要