AudioTagging Done Right: 2nd comparison of deep learning methods for environmental sound classification
Conference of the International Speech Communication Association (INTERSPEECH)(2022)
摘要
After its sweeping success in vision and language tasks, pure attention-based neural architectures (e.g. DeiT) are emerging to the top of audio tagging (AT) leaderboards, which seemingly obsoletes traditional convolutional neural networks (CNNs), feed-forward networks or recurrent networks. However, taking a closer look, there is great variability in published research, for instance, performances of models initialized with pretrained weights differ drastically from without pretraining, training time for a model varies from hours to weeks, and often, essences are hidden in seemingly trivial details. This urgently calls for a comprehensive study since our 1st comparison is half-decade old. In this work, we perform extensive experiments on AudioSet which is the largest weakly-labeled sound event dataset available, we also did an analysis based on the data quality and efficiency. We compare a few state-of-the-art baselines on the AT task, and study the performance and efficiency of 2 major categories of neural architectures: CNN variants and attention-based variants. We also closely examine their optimization procedures. Our opensourced experimental results provide insights to trade-off between performance, efficiency, optimization process, for both practitioners and researchers. Implementation: https://github.com/lijuncheng16/AudioTaggingDoneRight
更多查看译文
关键词
environmental sound classification,deep learning methods,deep learning
AI 理解论文
溯源树
样例
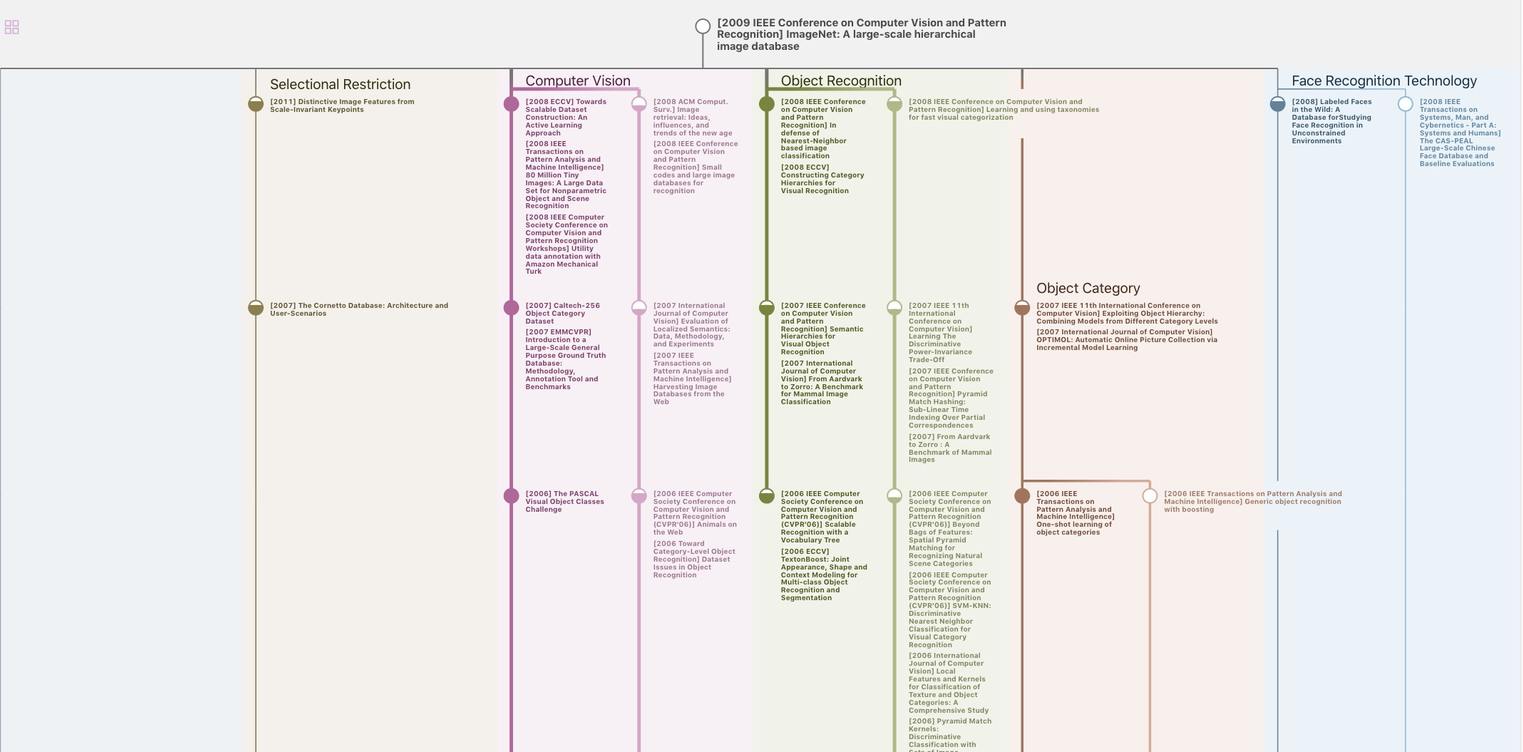
生成溯源树,研究论文发展脉络
Chat Paper
正在生成论文摘要