Non-Contact Heartbeat Detection Based on Ballistocardiogram Using UNet and Bidirectional Long Short-Term Memory
IEEE Journal of Biomedical and Health Informatics(2022)
摘要
Benefiting from non-invasive sensing tech- nologies, heartbeat detection from ballistocardiogram (BCG) signals is of great significance for home-care applications, such as risk prediction of cardiovascular disease (CVD) and sleep staging, etc. In this paper, we propose an effective deep learning model for automatic heartbeat detection from BCG signals based on UNet and bidirectional long short-term memory (Bi-LSTM). The developed deep learning model provides an effective solution to the existing challenges in BCG-aided heartbeat detection, especially for BCG in low signal-to-noise ratio, in which the waveforms in BCG signals are irregular due to measured postures, rhythm and artifact motion. For validations, performance of the proposed detection is evaluated by BCG recordings from 43 subjects with different measured postures and heart rate ranges. The accuracy of the detected heartbeat intervals measured in different postures and signal qualities, in comparison with the R-R interval of ECG, is promising in terms of mean absolute error and mean relative error, respectively, which is superior to the state-of-the-art methods. Numerical results demonstrate that the proposed UNet-BiLSTM model performs robust to noise and perturbations (e.g. respiratory effort and artifact motion) in BCG signals, and provides a reliable solution to long term heart rate monitoring.
更多查看译文
关键词
Algorithms,BCG Vaccine,Ballistocardiography,Heart Rate,Humans,Memory, Short-Term
AI 理解论文
溯源树
样例
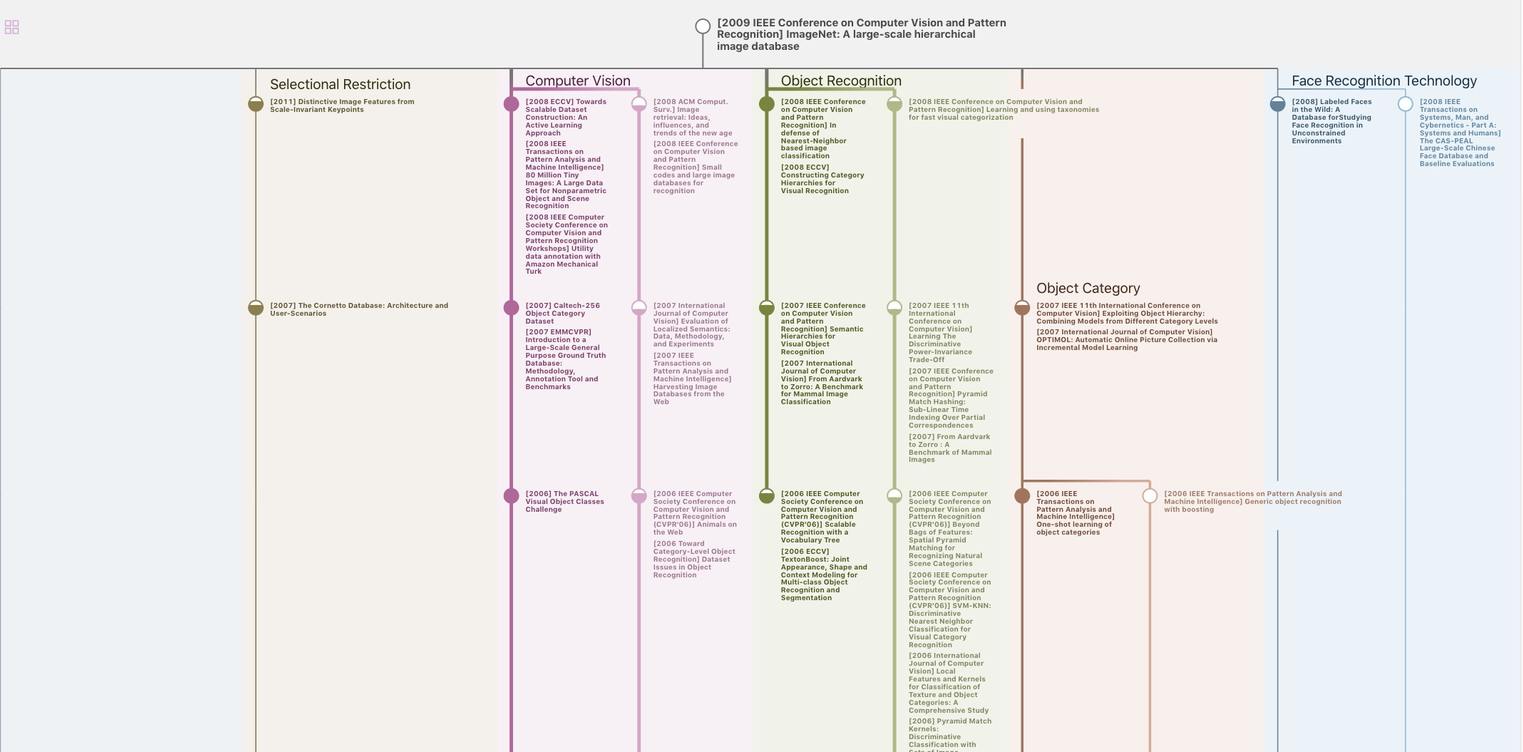
生成溯源树,研究论文发展脉络
Chat Paper
正在生成论文摘要