Traffic Anomaly Prediction Based on Joint Static-Dynamic Spatio-Temporal Evolutionary Learning
user-61442502e55422cecdaf6898(2022)
摘要
Accurate traffic anomaly prediction offers an opportunity to save the wounded at the right location in time. However, the complex process of traffic anomaly is affected by both various static factors and dynamic interactions. The recent evolving representation learning provides a new possibility to understand this complicated process, but with challenges of imbalanced data distribution and heterogeneity of features. To tackle these problems, this paper proposes a spatio-temporal evolution model named
SNIPER
for learning intricate feature interactions to predict traffic anomalies. Specifically, we design spatio-temporal encoders to transform spatio-temporal information into vector space indicating their natural relationship. Then, we propose a temporally dynamical evolving embedding method to pay more attention to rare traffic anomalies and develop an effective attention-based multiple graph convolutional network to formulate the spatially mutual influence from three different perspectives. The FC-LSTM is adopted to aggregate the heterogeneous features considering the spatio-temporal influences. Finally, a loss function is designed to overcome the ’over-smoothing’ and solve the imbalanced data problem. Extensive experiments show that SNIPER averagely outperforms state-of-the-arts by 3.9%, 0.9%, 1.9% and 1.6% on Chicago datasets, and 2.4%, 0.6%, 2.6% and 1.3% on New York City datasets in metrics of AUC-PR, AUC-ROC, F1 score, and accuracy, respectively.
更多查看译文
关键词
Anomaly prediction,spatio-temporal data,static-dynamic embedding,imbalanced data distribution,multiple graph convolutional network
AI 理解论文
溯源树
样例
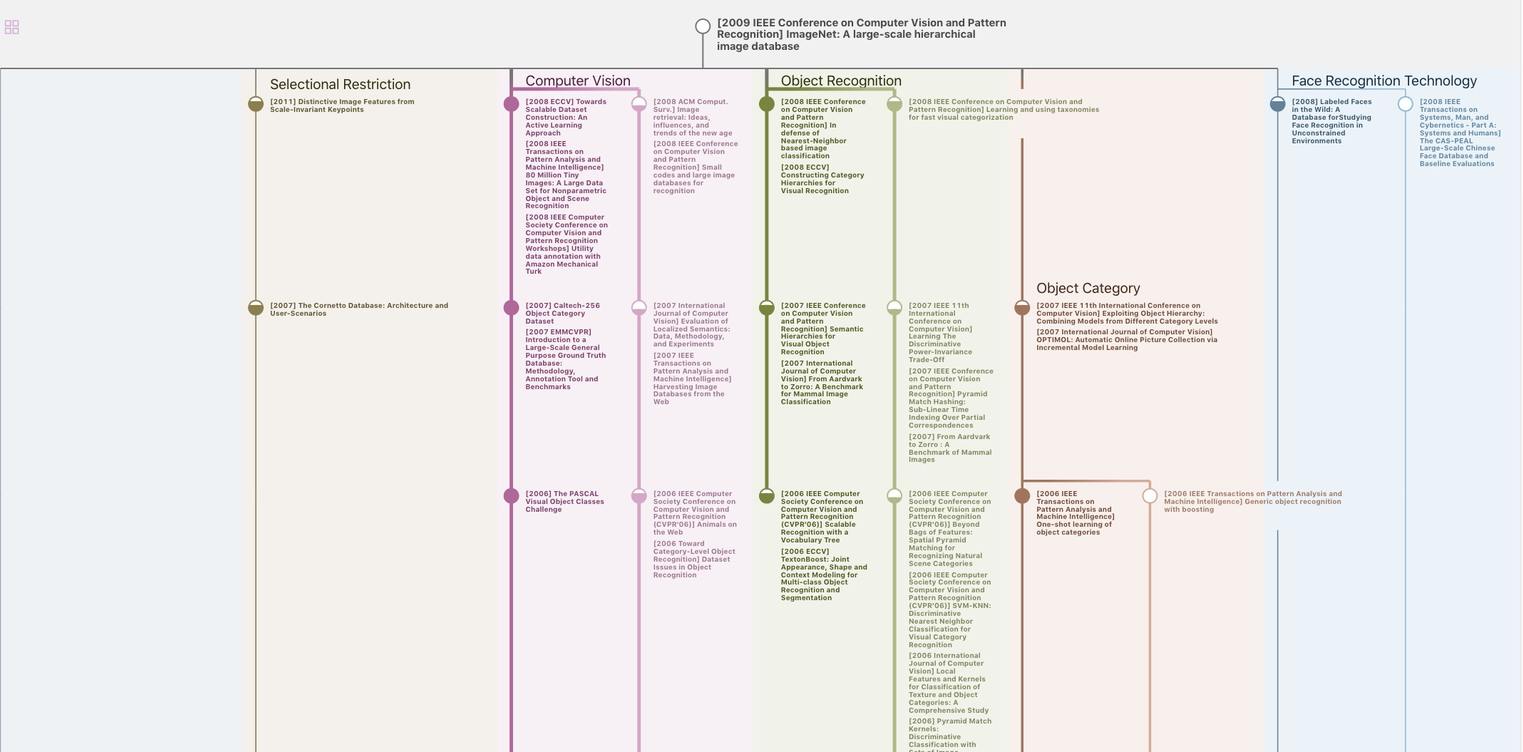
生成溯源树,研究论文发展脉络
Chat Paper
正在生成论文摘要