Learning Adaptive Node Embeddings across Graphs
user-61442502e55422cecdaf6898(2022)
摘要
Recently, learning embeddings of nodes in graphs has attracted increasing research attention. There are two main kinds of graph embedding methods, i.e., transductive embedding methods and inductive embedding methods. The former focuses on directly optimizing the embedding vectors, and the latter tries to learn a mapping function for the given nodes and features. However, little work has focused on applying the learned model from one graph to another, which is a pervasive idea in Computer Vision or Natural Language Processing. Although some of the graph neural networks (GNNs) present a similar motivation, none of them considers graph biases between graphs. In this paper, we present a novel graph embedding problem called Adaptive Task (AT), and propose a unified framework for the adaptive task, which introduces two types of alignment to learn adaptive node embeddings across graphs. Then, based on the proposed framework, a novel Graph Adaptive Embedding network (GraphAE) is designed to address the adaptive task. Furthermore, we extend GraphAE to a multi-graph version to consider a more complex adaptive situation. The extensive experimental results demonstrate that our model significantly outperforms the state-of-the-art methods, and also show that our framework can make a great improvement over a number of existing GNNs.
更多查看译文
关键词
Graph embedding,adaptive task,graph bias
AI 理解论文
溯源树
样例
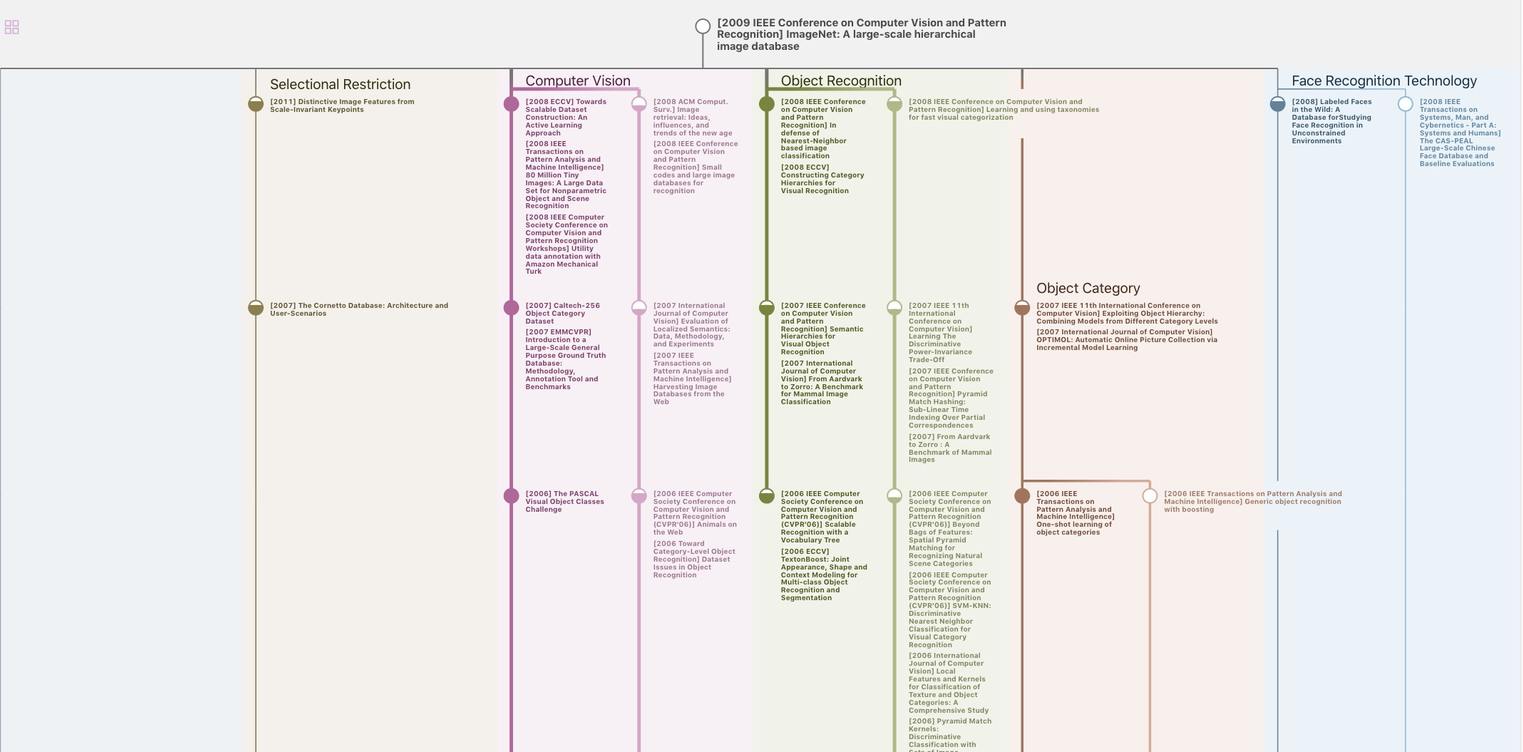
生成溯源树,研究论文发展脉络
Chat Paper
正在生成论文摘要