Adversarial Learning in Accelerometer Based Transportation and Locomotion Mode Recognition
user-616fadafe554226fb2b86252(2022)
摘要
This chapter demonstrates how adversarial learning can be used in the mobile computing domain. Specifically, we address the problem of improving the recognition of human activities from smartphone sensors, when limited training data is available. Generative Adversarial Networks (GANs) provide an approach to model the distribution of a dataset and can be used to augment data to reduce the amount of labelled data required to train accurate classifiers. By introducing another fully connected neural network as classifier into a conditional GAN framework, we utilise the adversarial learning approaches between discriminator and generator and between discriminator and classifier to perform semi–supervised learning on labelled and unlabelled samples. We evaluate our approach on the recognition of 8 modes of transportation and locomotion using a publicly available dataset. This dataset is well established and has led to 3 public machine learning challenges, which allows us to contrast our approach to the state of the art. Our GAN operates on 150 features extracted from 5s windows captured by a smartphone acceleration sensor carried at the hips. The most promising features are selected based on maximum relevance – minimum redundancy feature selection. We use Bayesian Search for hyperparameter optimisation. The resulting GAN classifier achieves 49% F1 score on a user independent evaluation, drastically outperforming our baseline at 35% F1 score.
更多查看译文
关键词
locomotion mode recognition,accelerometer based transportation,learning
AI 理解论文
溯源树
样例
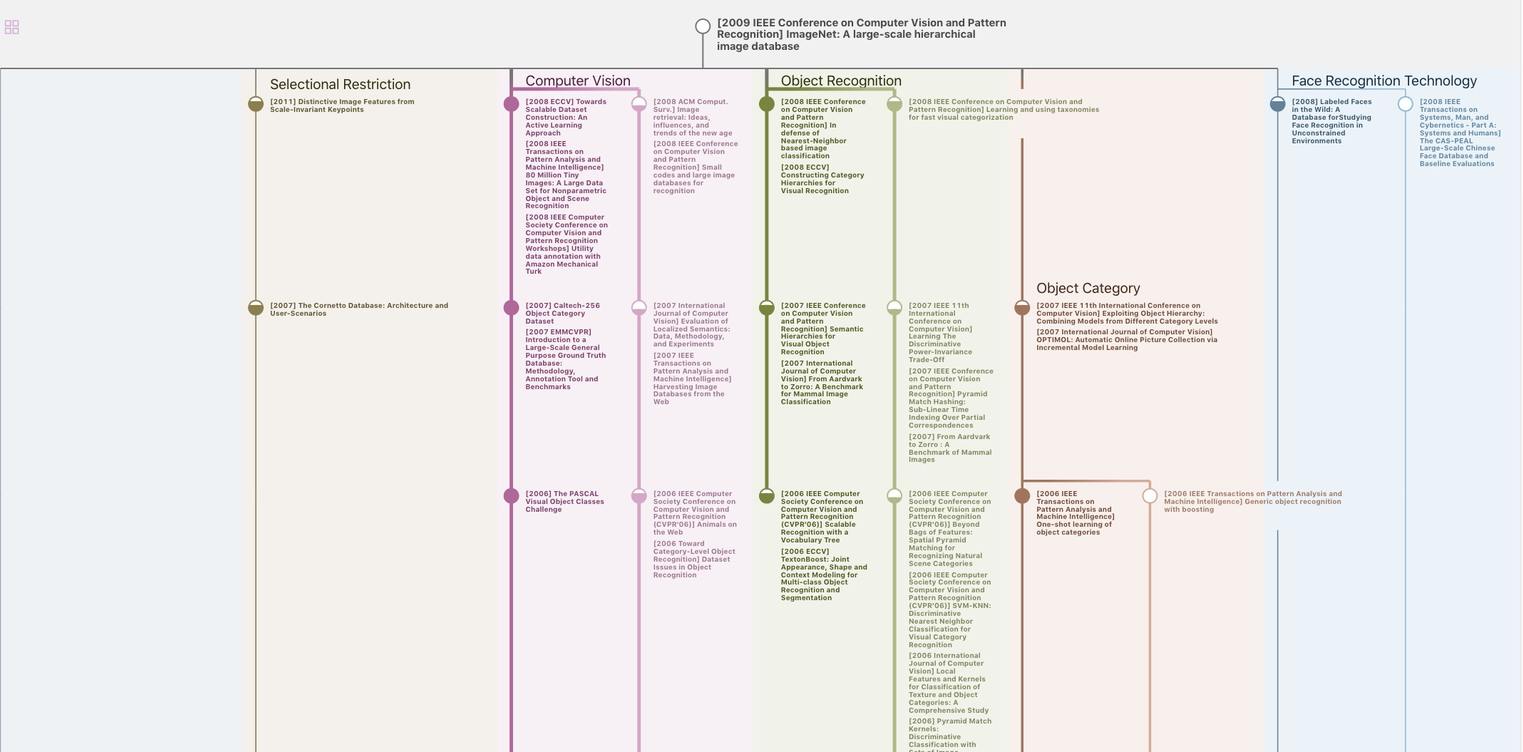
生成溯源树,研究论文发展脉络
Chat Paper
正在生成论文摘要