A Knowledge-Aided Robust Ensemble Kalman Filter Algorithm for Non-Linear and Non-Gaussian Large Systems
FRONTIERS IN APPLIED MATHEMATICS AND STATISTICS(2022)
摘要
This work proposes a robust and non-Gaussian version of the shrinkage-based knowledge-aided EnKF implementation called Ensemble Time Local H-infinity Filter Knowledge-Aided (EnTLHF-KA). The EnTLHF-KA requires a target covariance matrix to integrate previously obtained information and knowledge directly into the data assimilation (DA). The proposed method is based on the robust H-infinity filter and on its ensemble time-local version the EnTLHF, using an adaptive inflation factor depending on the shrinkage covariance estimated matrix. This implies a theoretical and solid background to construct robust filters from the well-known covariance inflation technique. The proposed technique is implemented in a synthetic assimilation experiment, and in an air quality application using the LOTOS-EUROS model over the Aburra Valley to evaluate its potential for non-linear and non-Gaussian large systems. In the spatial distribution of the PM2.5 concentrations along the valley, the method outperforms the well-known Local Ensemble Transform Kalman Filter (LETKF), and the non-robust knowledge-aided Ensemble Kalman filter (EnKF-KA). In contrast to the other simulations, the ability to issue warnings for high concentration events is also increased. Finally, the simulation using EnTLHF-KA has lower error values than using EnKF-KA, indicating the advantages of robust approaches in high uncertainty systems.
更多查看译文
关键词
data assimilation, air quality modeling, robust estimation, Ensemble Kalman filter, covariance estimation
AI 理解论文
溯源树
样例
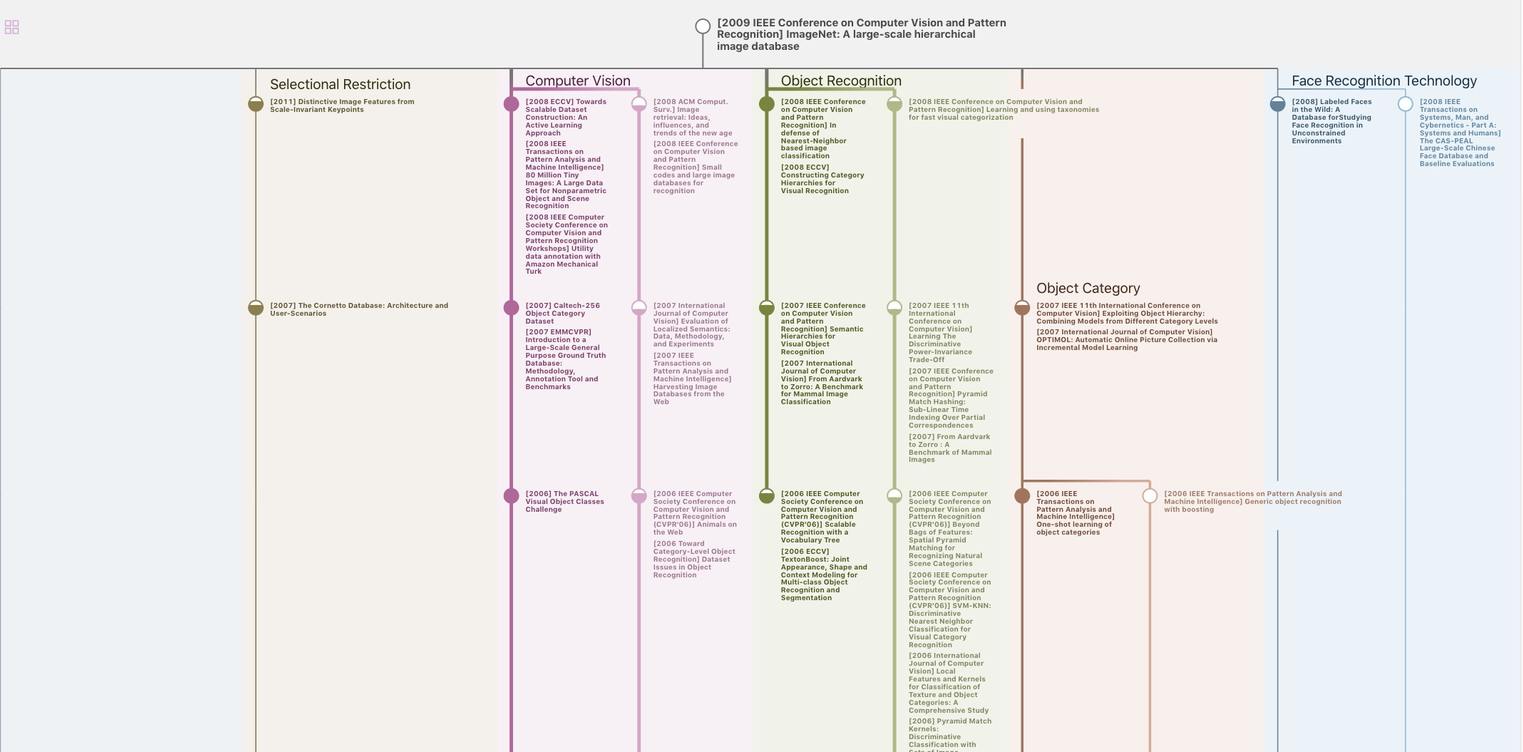
生成溯源树,研究论文发展脉络
Chat Paper
正在生成论文摘要