Robust text line detection in historical documents: learning and evaluation methods
International Journal on Document Analysis and Recognition (IJDAR)(2022)
摘要
Text line segmentation is one of the key steps in historical document understanding. It is challenging due to the variety of fonts, contents, writing styles and the quality of documents that have degraded through the years. In this paper, we address the limitations that currently prevent people from building line segmentation models with a high generalization capacity. We present a study conducted using three state-of-the-art systems Doc-UFCN, dhSegment and ARU-Net and show that it is possible to build generic models trained on a wide variety of historical document datasets that can correctly segment diverse unseen pages. This paper also highlights the importance of the annotations used during training: Each existing dataset is annotated differently. We present a unification of the annotations and show its positive impact on the final text recognition results. In this end, we present a complete evaluation strategy using standard pixel-level metrics, object-level ones and introducing goal-oriented metrics.
更多查看译文
关键词
Text line detection, Historical documents, Generic architecture, Evaluation strategy, Goal-directed evaluation
AI 理解论文
溯源树
样例
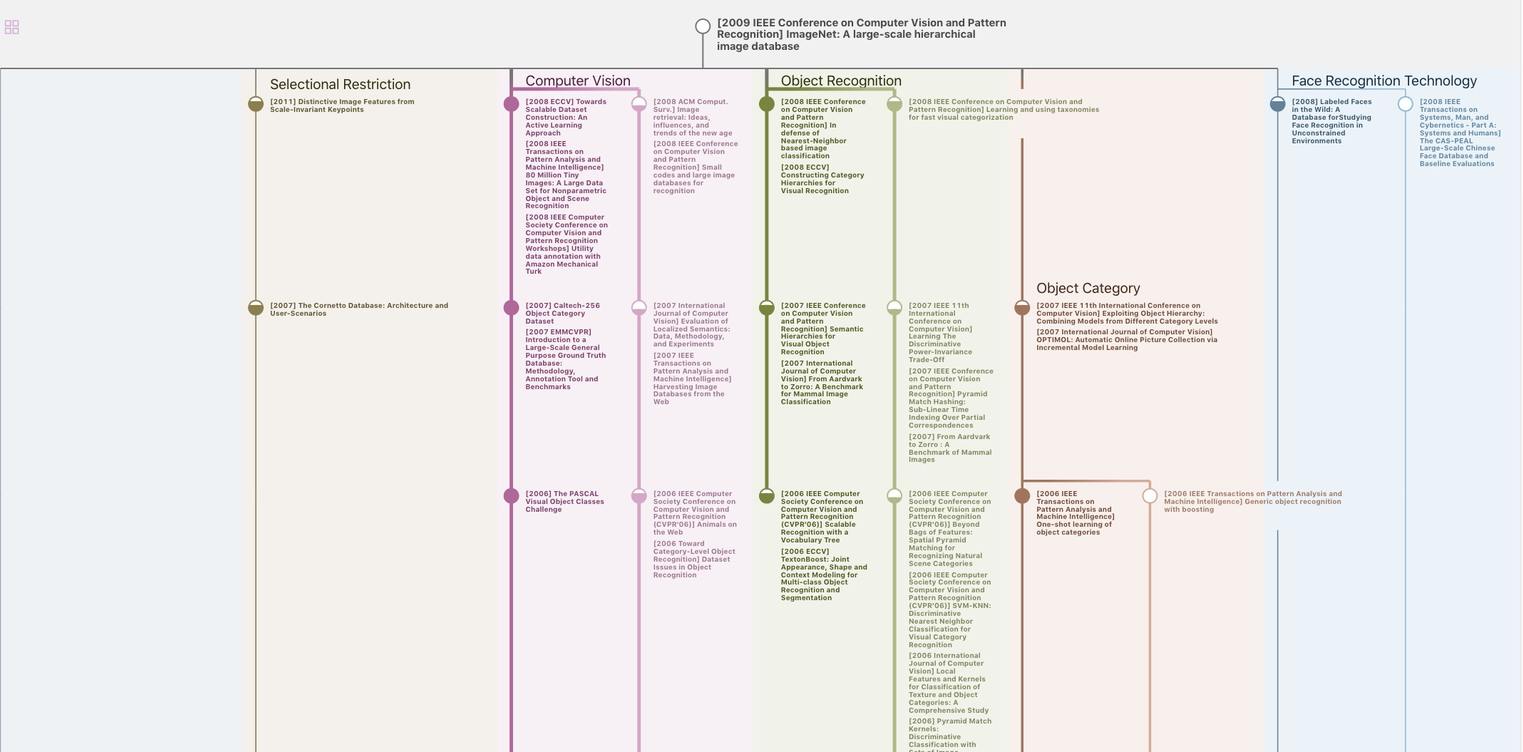
生成溯源树,研究论文发展脉络
Chat Paper
正在生成论文摘要