Considerations when learning additive explanations for black-box models
user-6073b1344c775e0497f43bf9(2023)
摘要
Many methods to explain black-box models, whether local or global, are additive. In this paper, we study global additive explanations for non-additive models, focusing on four explanation methods: partial dependence, Shapley explanations adapted to a global setting, distilled additive explanations, and gradient-based explanations. We show that different explanation methods characterize non-additive components in a black-box model’s prediction function in different ways. We use the concepts of main and total effects to anchor additive explanations, and quantitatively evaluate additive and non-additive explanations. Even though distilled explanations are generally the most accurate additive explanations, non-additive explanations such as tree explanations that explicitly model non-additive components tend to be even more accurate. Despite this, our user study showed that machine learning practitioners were better able to leverage additive explanations for various tasks. These considerations should be taken into account when considering which explanation to trust and use to explain black-box models.
更多查看译文
关键词
Black-box models,Additive explanations,Model distillation,Interaction effects,Correlated features
AI 理解论文
溯源树
样例
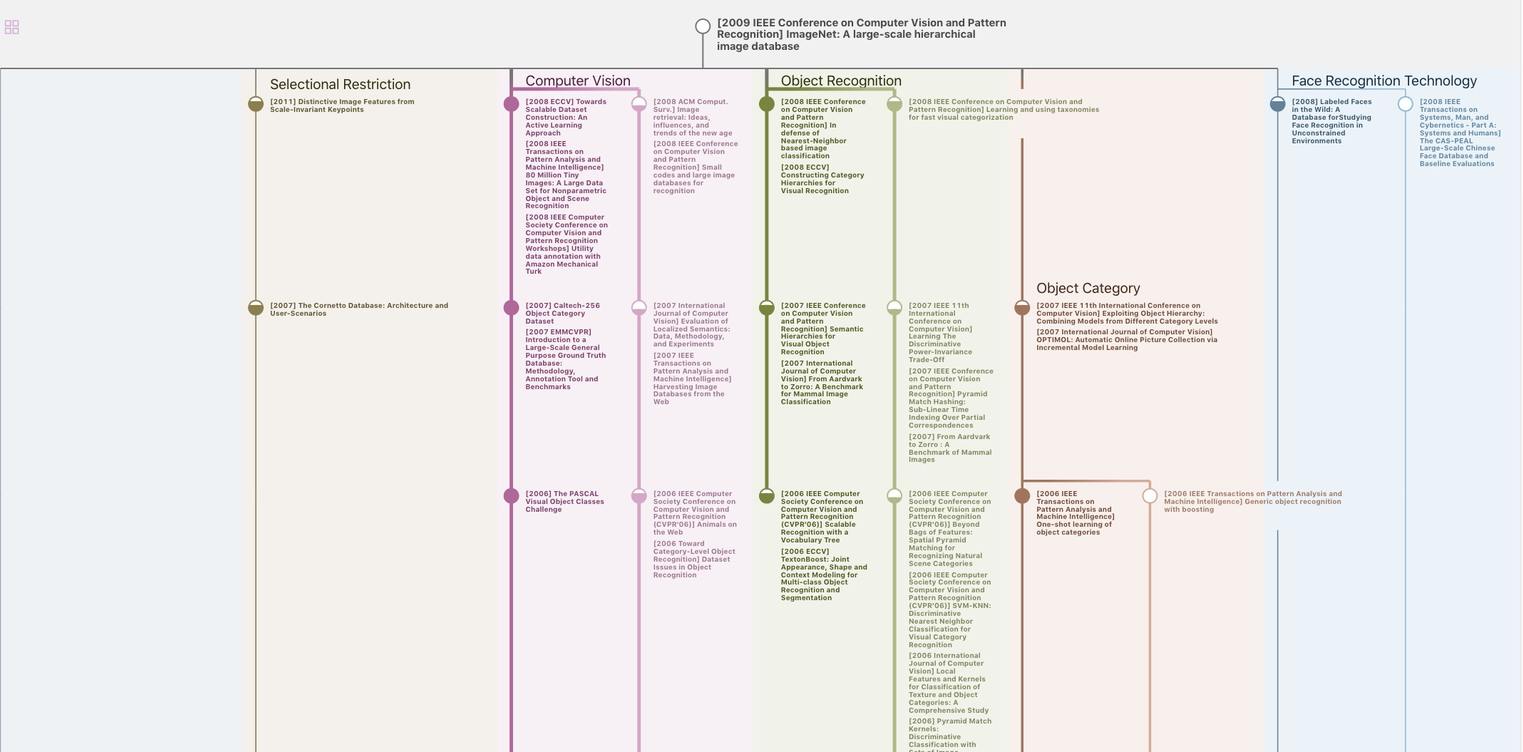
生成溯源树,研究论文发展脉络
Chat Paper
正在生成论文摘要